Introduction
Artificial Intelligence (AI) is reshaping the landscape of health research, and nowhere is this more evident than in the analysis of Heart Rate Variability (HRV) data. HRV is a critical biomarker that reflects the balance and adaptability of the autonomic nervous system. When monitored over long periods, HRV provides a window into a person’s overall health, capturing subtle fluctuations that short-term measurements might miss.
However, long-term HRV data is both a treasure trove and a challenge. The sheer volume of data collected over days, weeks, or months can overwhelm traditional analytical methods. This is where AI steps in, offering powerful tools to process, analyze, and extract meaningful insights from complex datasets.
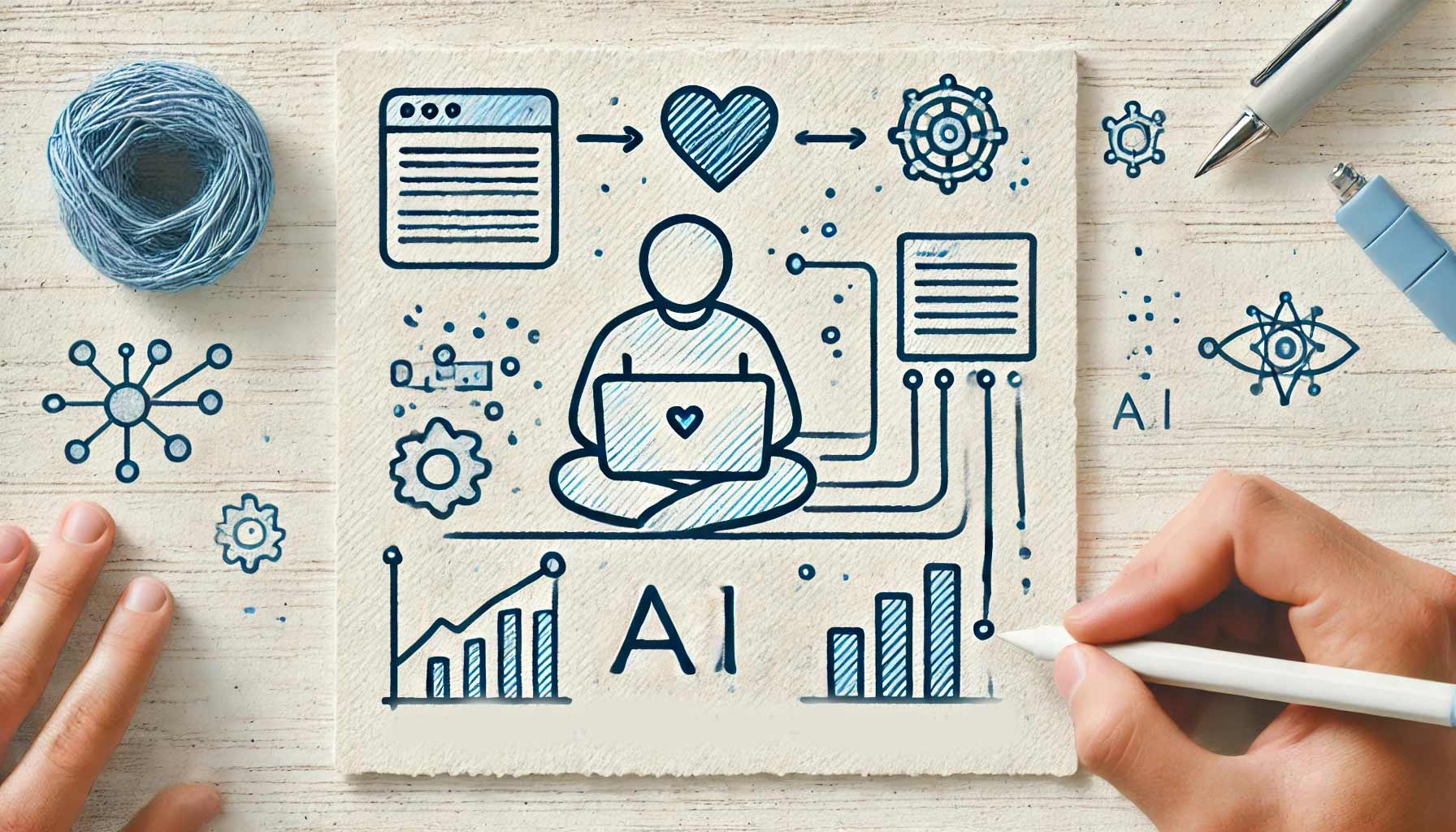
The Role of AI in Long-Term HRV Data Analysis
Long-term HRV monitoring generates vast amounts of data, often containing hidden patterns that are not immediately obvious. Traditional statistical methods can struggle to identify these patterns, especially when the data is noisy or incomplete. AI, particularly machine learning, excels in this domain by learning from the data itself, recognizing patterns, and making predictions.
For instance, machine learning models can be trained on HRV data to detect early signs of health deterioration, such as the onset of cardiovascular disease or the buildup of chronic stress. These models can handle the intricacies of HRV data, accounting for individual differences and adapting to new data over time.
One of the most powerful applications of AI in HRV analysis is deep learning. This subset of machine learning involves neural networks that can analyze data in layers, identifying complex relationships that simpler models might overlook. In the context of HRV, deep learning can uncover subtle patterns linked to specific health outcomes, providing researchers with insights that can inform both preventative measures and personalized treatment plans.
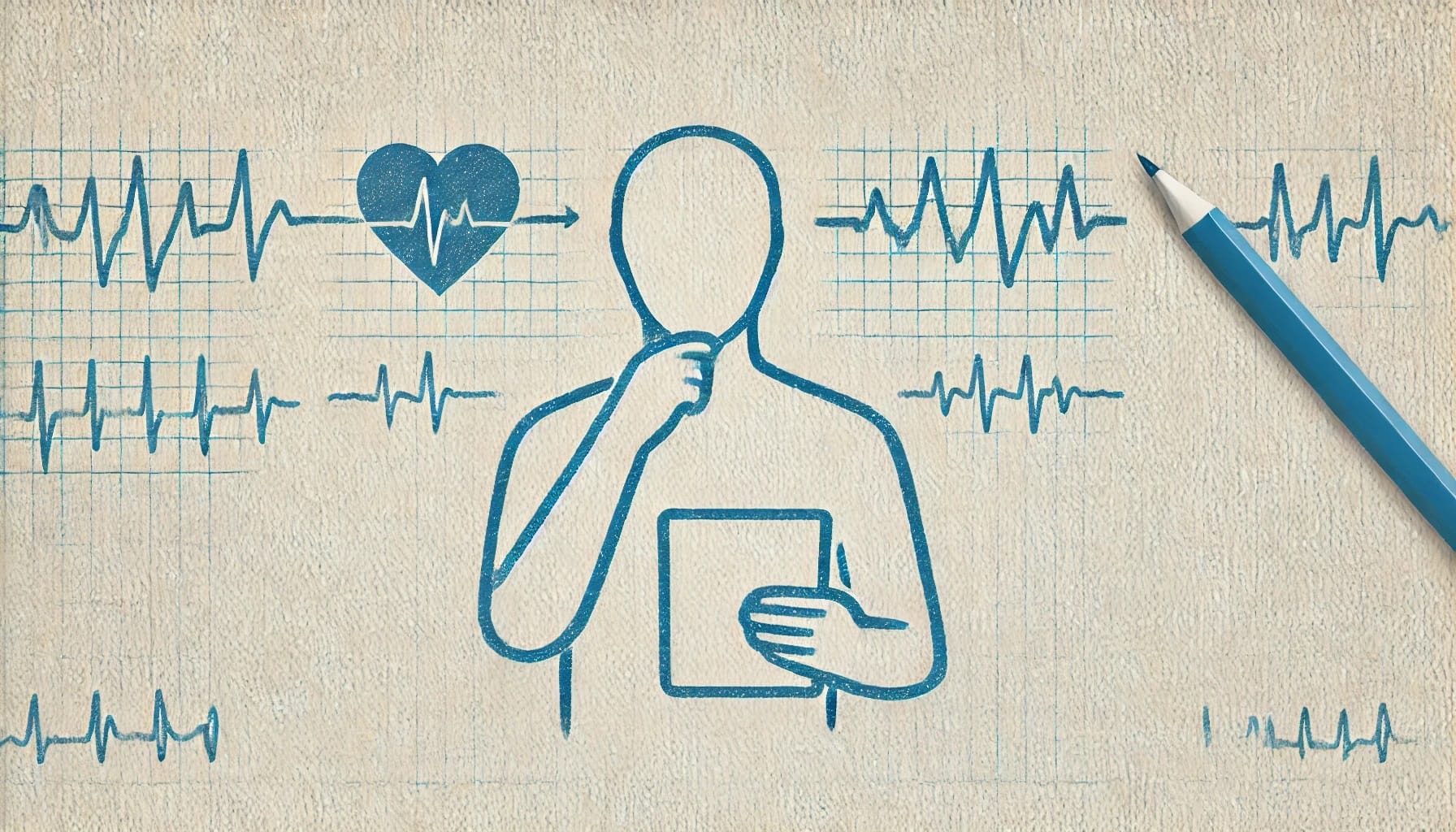
Practical Applications of AI in HRV Research
The application of AI in HRV research is not just theoretical – it’s already making a difference in the real world. In predictive modeling, AI enhances the ability to forecast health outcomes based on HRV data. For example, researchers can use AI to predict the likelihood of a heart attack or stroke by analyzing long-term trends in a person’s HRV. This predictive power is particularly valuable in chronic disease management, where early intervention can significantly improve patient outcomes.
AI also plays a crucial role in personalized health monitoring. By analyzing an individual’s HRV data in real-time, AI can provide tailored recommendations for lifestyle changes, stress management, and even exercise routines. This level of personalization is a game-changer for fields like sports science, where optimizing an athlete’s training and recovery is essential for peak performance.
Moreover, AI-driven HRV analysis is making strides in mental health research. Continuous monitoring of HRV, combined with AI’s ability to process vast amounts of data, allows for a more nuanced understanding of how stress and anxiety affect the body over time. This can lead to more effective mental health interventions, customized to the needs of each individual.
In the next part of this article, we will delve deeper into the challenges of applying AI to HRV data, including data quality issues and the interpretability of AI models. We will also explore future trends, such as the integration of AI with wearable technology, that promise to further enhance the field of HRV research. Stay tuned for insights into how AI is not just analyzing HRV data but revolutionizing the way we understand and apply it in health research.
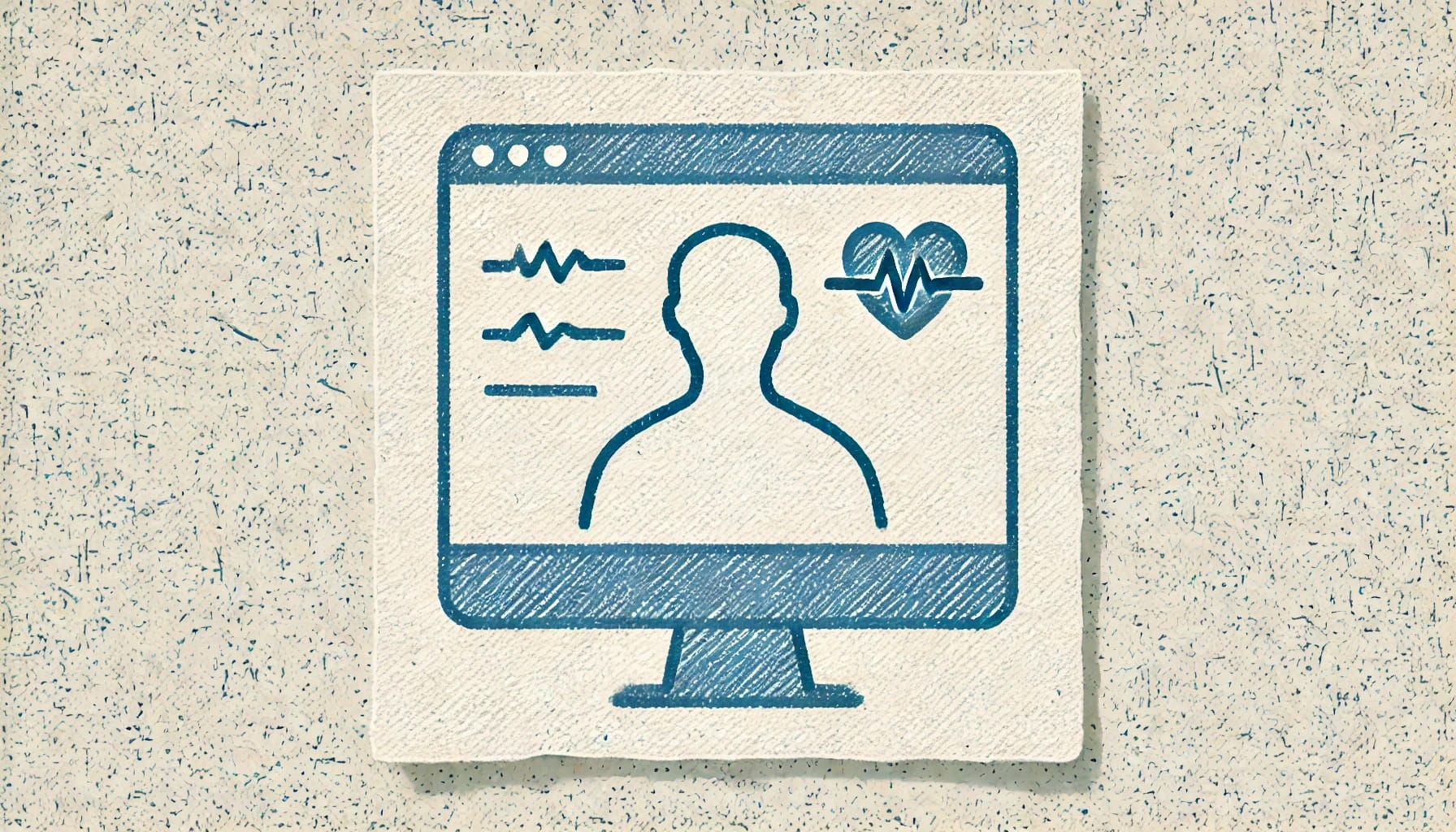
Challenges in AI-Driven HRV Data Analysis
While AI offers powerful tools for analyzing long-term HRV data, it’s not without its challenges. One of the primary issues researchers face is data quality. HRV data, particularly when collected over long periods, can be prone to noise and inconsistencies. Variations in sensor performance, changes in user behavior, and even environmental factors can introduce artifacts that complicate analysis. AI models, no matter how advanced, rely on the quality of the data they are fed. If the input data is flawed, the output – whether it’s a predictive model or a personalized health insight – can be equally unreliable.
To address these issues, AI-driven preprocessing techniques are essential. These techniques can filter out noise, correct for missing data, and standardize inputs to ensure that the analysis is as accurate as possible. For example, machine learning algorithms can be trained to recognize and exclude irregularities in HRV data, such as those caused by poor sensor contact or movement artifacts. This preprocessing step is crucial for maintaining the integrity of the analysis and ensuring that the insights generated are both accurate and actionable.
Another significant challenge is the interpretability of AI models. While AI, particularly deep learning, can uncover complex patterns in HRV data, these models often operate as “black boxes,” making it difficult for researchers to understand how they arrive at their conclusions. This lack of transparency can be a barrier, especially in health research, where understanding the underlying mechanisms is as important as the outcomes themselves.
To overcome this, researchers are increasingly focusing on developing interpretable AI models. These models are designed to be more transparent, allowing researchers to trace how decisions are made and to ensure that the AI’s conclusions are based on sound reasoning. Techniques such as feature importance analysis, where the AI highlights which aspects of the data most influence its predictions, can help bridge the gap between AI’s computational power and the need for human understanding.
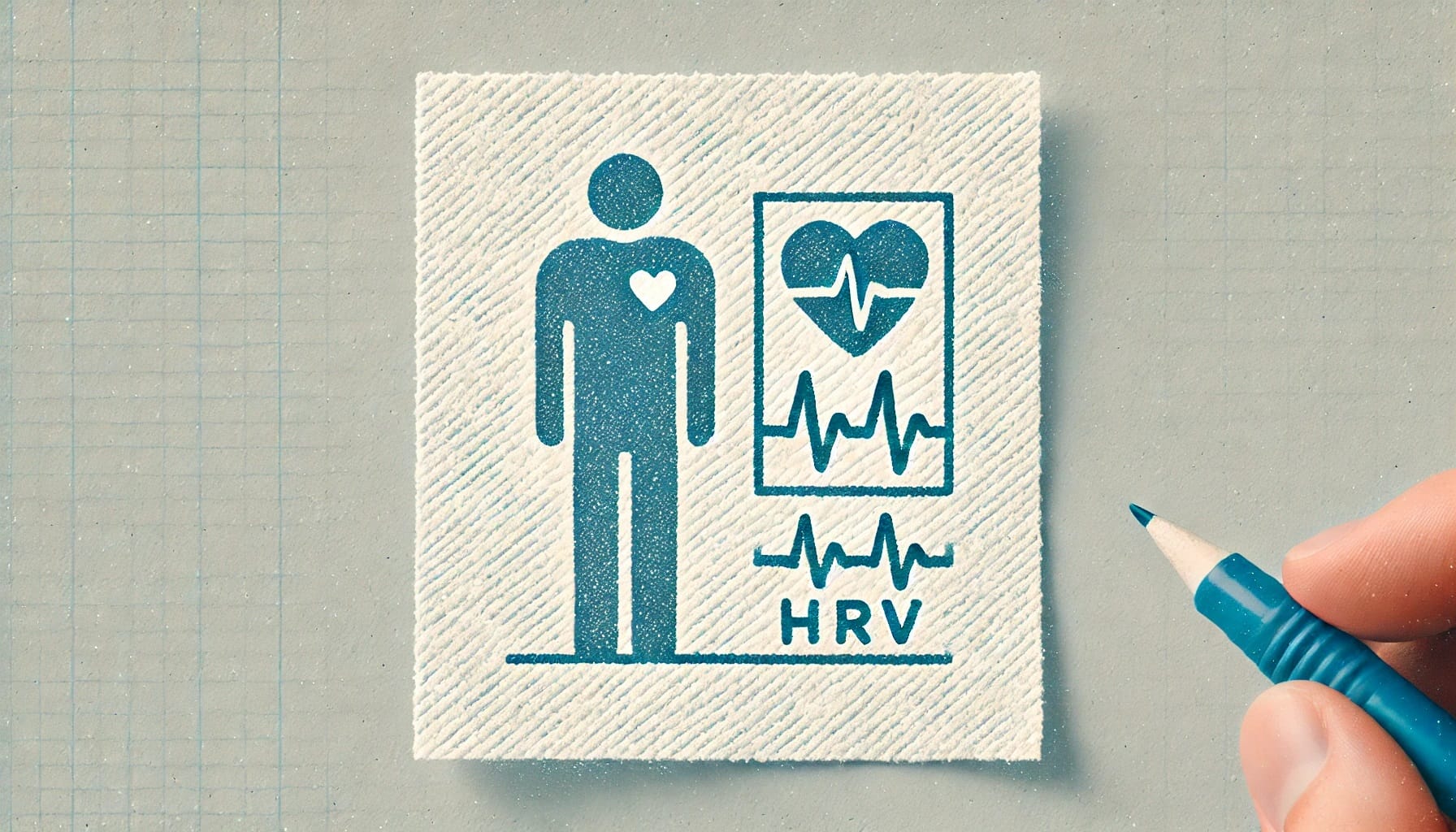
Future Trends in AI for HRV Analysis
Looking ahead, the integration of AI with wearable technology promises to take HRV research to the next level. Wearables are already capable of collecting vast amounts of HRV data in real-time, but the addition of AI can make this data immediately actionable. Imagine a future where your wearable device not only tracks your HRV but also uses AI to provide instant feedback on your stress levels, suggesting relaxation techniques or adjustments to your daily routine to optimize your well-being.
Emerging AI techniques, such as reinforcement learning and transfer learning, are also poised to enhance HRV analysis. Reinforcement learning, which involves AI learning through trial and error, could be used to develop personalized health strategies that adapt to an individual’s changing needs over time. Transfer learning, where a model developed for one task is repurposed for another, could allow insights gained from one population to be applied to another, speeding up the research process and broadening the applicability of findings.
Moreover, the ongoing evolution of AI is likely to make these technologies more accessible to researchers. As AI tools become more user-friendly, even those without extensive backgrounds in computer science will be able to apply sophisticated AI techniques to their HRV data. This democratization of AI could lead to a surge in innovative HRV research, with more scientists exploring the possibilities that long-term HRV monitoring offers.
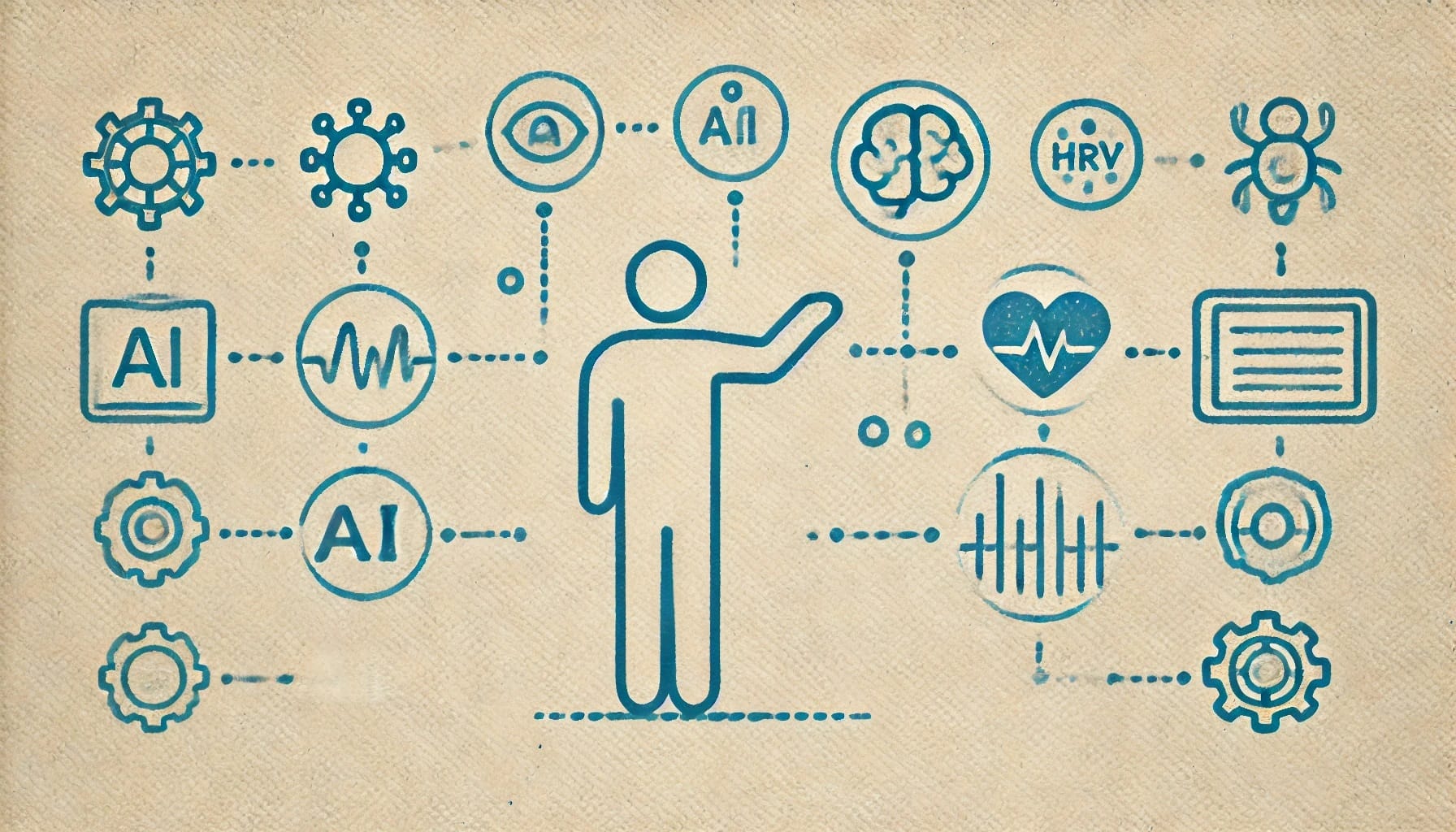
Conclusion
AI is revolutionizing the way we approach long-term HRV data analysis, addressing the challenges of data quality, handling large datasets, and uncovering personalized health insights. While there are still hurdles to overcome, the potential for AI to enhance HRV research is immense. As AI continues to evolve, so too will our ability to understand and utilize HRV data, opening new frontiers in health research.
As we continue this series, we’ll dive deeper into specific AI tools and platforms that researchers can use to analyze HRV data, providing practical guidance on how to integrate these technologies into your research workflow. The future of HRV analysis is bright, and with AI, it’s within reach. Stay tuned for more insights and practical tips on harnessing the power of AI in your HRV research.
Call to Action
📅 If you want to learn more about Fibion’s solution for measuring HRV, do not hesitate to book a video call with our expert Dr. Miriam Cabrita.
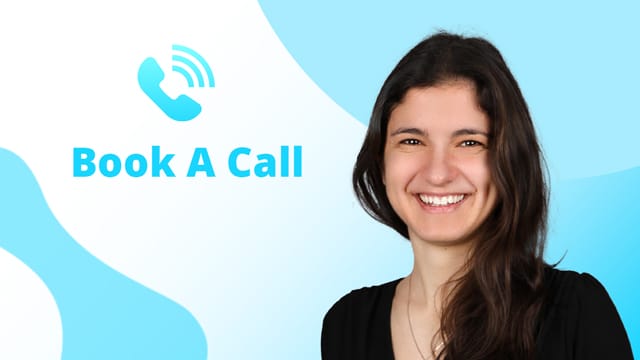
🔍 You may also discover other valid and reliable products in our portfolio, such as the Fibion Research, Fibion Vitals, Fibion Sleep, Fibion Sens, Fibion Emfit, and Fibion Circadian, all designed to assist in research measuring physical activity, sedentary behavior, and sleep.
Frequently asked questions about this topic:
How does AI enhance long-term HRV data analysis? +
AI provides tools to process extensive HRV data, uncovering patterns and insights that may be missed by traditional methods. It can predict health trends and customize interventions based on an individual’s HRV data.
What is the importance of analyzing HRV data over a long period? +
Long-term HRV analysis captures subtle health trends that short-term analysis may miss, allowing for a more comprehensive understanding of a person’s health, stress levels, and potential risk factors for chronic conditions.
Can AI predict health issues using HRV data? +
Yes, AI algorithms can detect patterns in HRV data that may indicate early signs of health problems, such as cardiovascular diseases or chronic stress, enabling earlier and more targeted interventions.
How is AI used in mental health research through HRV analysis? +
AI helps analyze continuous HRV data to understand how stress and anxiety impact the body over time. This analysis can lead to personalized mental health interventions based on individual stress response patterns.
What challenges are associated with AI-driven HRV analysis? +
Key challenges include data quality issues, such as noise and inconsistencies, and the interpretability of AI models. Efforts are underway to create more transparent AI models and improve data preprocessing techniques.
What is the future of AI in HRV analysis? +
The future holds significant advancements with the integration of AI and wearable technology, enabling real-time health feedback. New AI techniques like reinforcement and transfer learning will further personalize and expand HRV research.