Falls among the elderly are not just accidents – they’re often the tipping point that leads to a decline in independence and quality of life. Each year, millions of older adults experience falls, leading to severe injuries such as fractures and head trauma. As the global population ages, the need for effective fall prevention strategies has never been more urgent. Fortunately, advances in wearable sensor technology, paired with artificial intelligence (AI), are providing promising solutions to this critical issue.
1. Understanding the Risks of Falls in Elderly People
Falls in older adults can result from a combination of intrinsic and extrinsic factors that compromise their stability and mobility. Recognizing these risks is the first step in mitigating them.
- Intrinsic Factors:
- Muscle Weakness: Loss of muscle strength, particularly in the legs, reduces stability.
- Balance Disorders: Inner ear problems and other balance disorders make standing and walking hazardous.
- Chronic Conditions: Conditions like Parkinson’s disease, arthritis, and diabetes can impair movement and balance.
- Medications: Some medications, especially those affecting the central nervous system, can cause dizziness or sedation.
- Extrinsic Factors:
- Environmental Hazards: Slippery floors, poor lighting, and clutter can increase fall risks.
- Improper Footwear: Wearing shoes without proper support can lead to slips and trips.
- Lack of Assistive Devices: Not using mobility aids like canes or walkers when needed can elevate the risk of falls.
The consequences of falls can be devastating, ranging from minor injuries to severe outcomes like hip fractures, traumatic brain injuries, and even death. Falls also lead to psychological impacts, including a fear of falling again, which can result in reduced physical activity, social isolation, and further deterioration of health. Understanding these risks underscores the importance of proactive measures to prevent falls, and that’s where wearable technology and AI come into play.
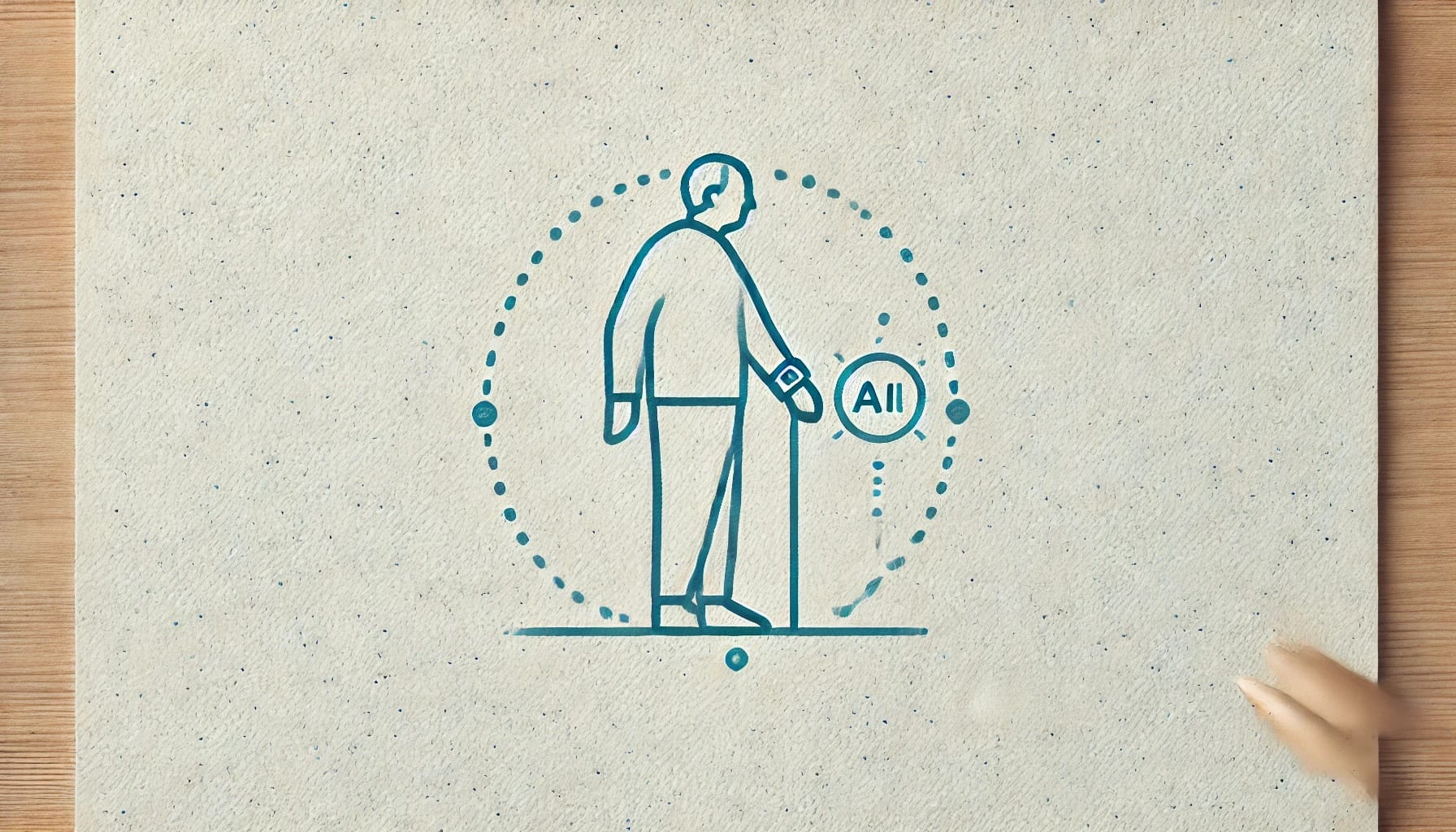
2. The Role of Wearable Sensors in Monitoring and Fall Prevention
Wearable sensors have emerged as a powerful tool in the prevention of falls among the elderly, offering continuous monitoring of various physiological and movement parameters. These small, unobtrusive devices can be worn in different positions on the body and provide valuable data that can be analyzed to detect fall risks and respond promptly.
- Types of Sensors:
- Accelerometers: Measure movement and acceleration, detecting sudden changes that might indicate a fall.
- Gyroscopes: Monitor orientation and balance, helping to detect when a person is off-balance.
- Pressure Sensors: Used in insoles or mats, they track weight distribution and identify imbalances.
- Key Benefits of Wearable Sensors:
- Continuous Monitoring: Provides real-time data on a person’s movements and balance, which can alert caregivers to potential falls.
- Early Detection: Sensors can identify subtle changes in gait or posture that precede a fall, allowing for timely intervention.
- Personalized Feedback: Data from wearables can be used to tailor interventions and exercises to improve balance and strength.
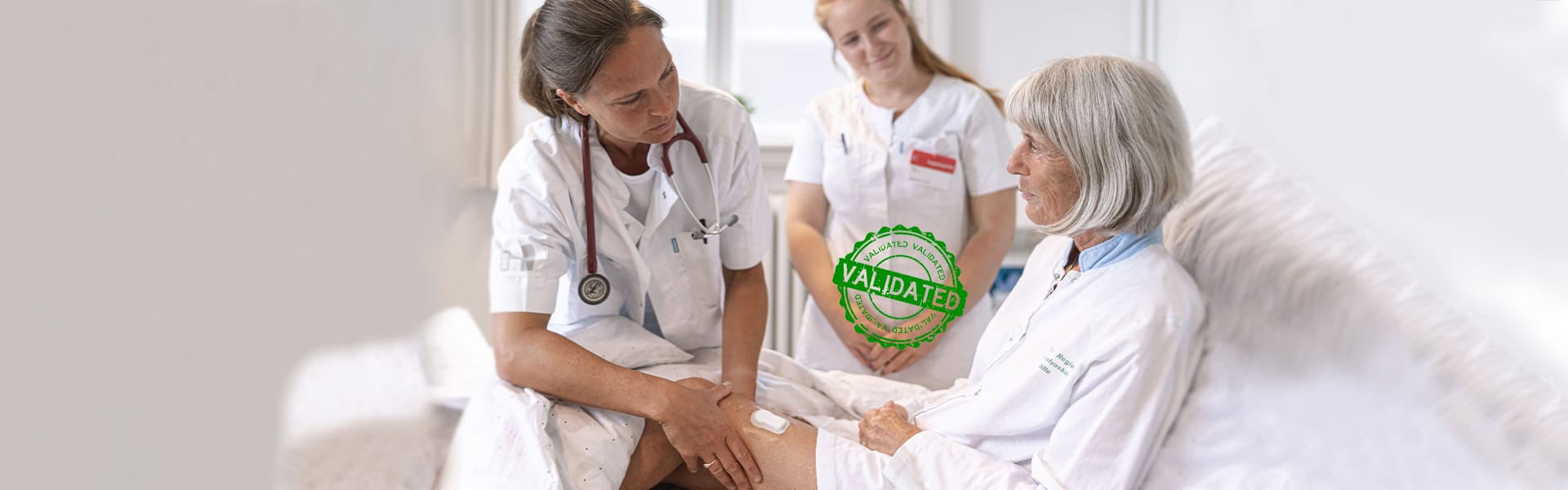
Examples of wearable devices include Fibion SENS, which is typically worn on the thigh and excels at capturing detailed leg dynamics crucial for assessing walking patterns. Other devices like Fibion Vitals, worn on the chest, offer comprehensive multi-signal measurements that include upper body movements, respiration, and heart rate – providing a holistic view of a person’s fall risk profile.
By incorporating AI, these sensors do more than just track movements – they analyze complex data sets to predict falls before they happen. This predictive capability, combined with continuous monitoring, positions wearable sensors as an essential component in the future of fall prevention strategies for the elderly.
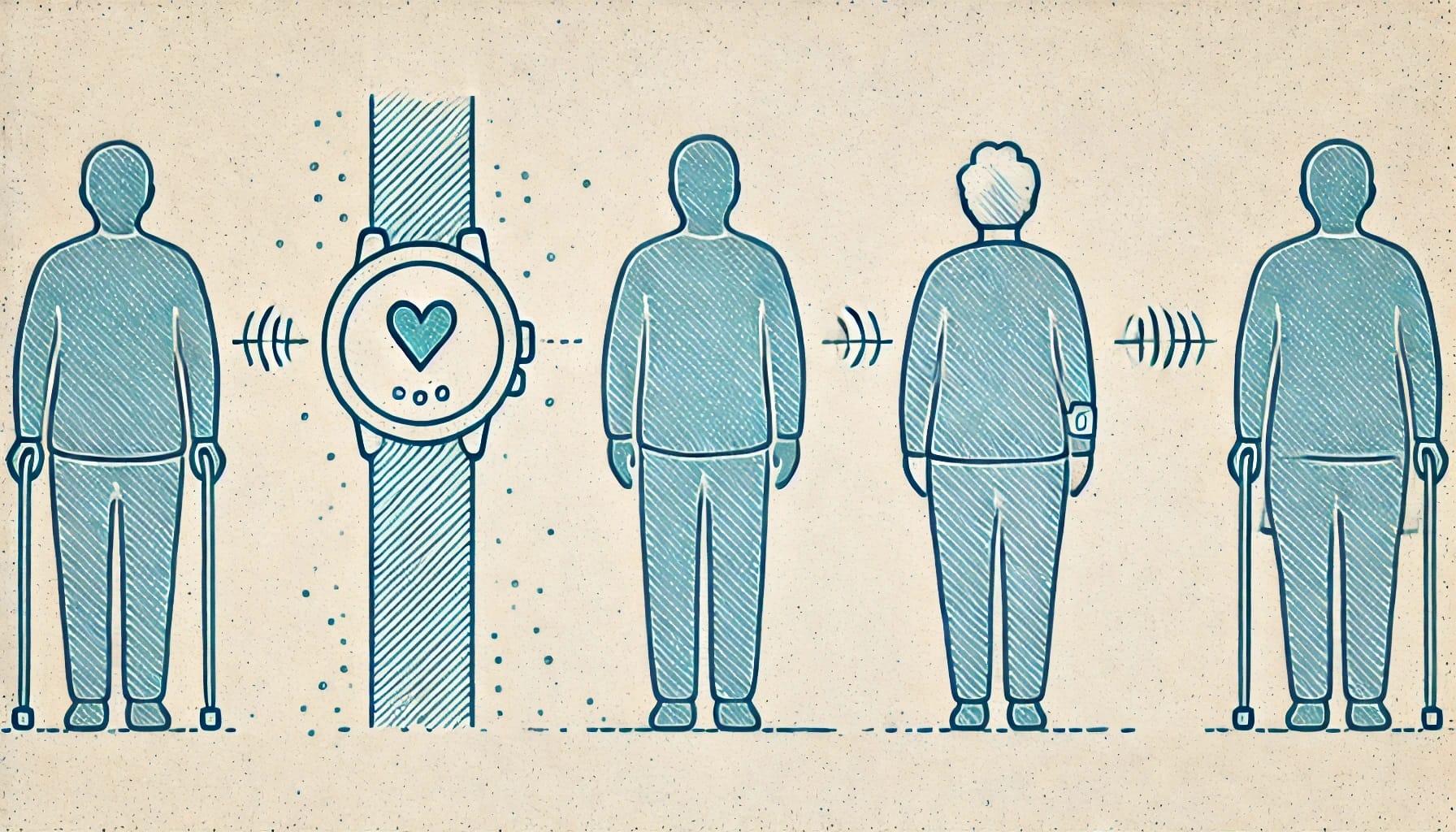
3. Artificial Intelligence: Enhancing Fall Prevention
Artificial intelligence takes wearable sensors to the next level by transforming raw data into actionable insights. Through advanced algorithms, AI can detect patterns and anomalies in movement that humans might miss, making it a powerful ally in fall prevention.
- How AI Works with Wearable Sensors:
- Data Processing: AI algorithms analyze data from sensors in real-time, assessing factors like gait speed, balance, and body posture.
- Pattern Recognition: Machine learning models are trained to recognize patterns associated with high fall risk, such as slow walking speed, erratic gait, or frequent stumbles.
- Predictive Analysis: Beyond detecting falls, AI can predict when a fall is likely to occur by identifying gradual changes in a person’s movement patterns over time.
- Practical Applications:
- Real-Time Alerts: AI-driven wearables can send alerts to caregivers or family members if a fall is detected or if there is a significant risk, allowing for immediate intervention.
- Personalized Interventions: Based on the data collected, AI can suggest personalized exercise routines or adjustments in daily activities to reduce fall risk.
- Behavioral Insights: AI provides insights into the wearer’s daily habits, identifying times or situations when they are most at risk, such as getting out of bed or navigating stairs.
For instance, AI applications in wearable devices like the Fibion SENS and Fibion Vitals can distinguish between typical movements and those that signal a potential fall, offering a level of precision that traditional monitoring methods cannot achieve. This technology doesn’t just react to falls; it anticipates them, empowering users to take proactive steps toward safety.
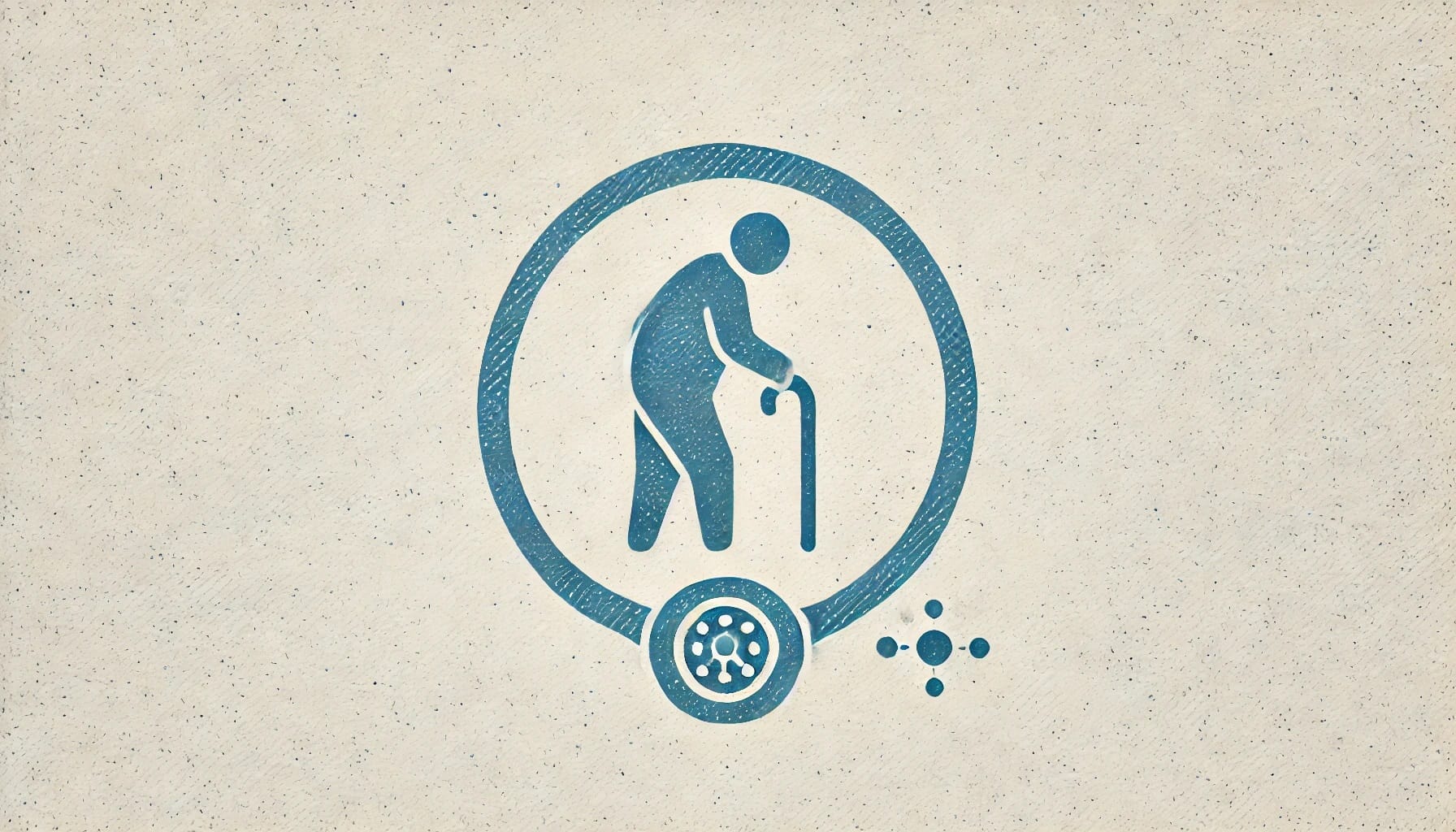
4. Predicting Fall Risks: Slow Walking Speed and Altered Gait Patterns
Walking is one of the most fundamental indicators of mobility and independence in the elderly. Changes in walking speed and gait patterns are often early warning signs of fall risk.
- Slow Walking Speed:
- Walking slower than usual can indicate declining physical capabilities and a higher likelihood of falling. AI-powered wearables measure and analyze walking speed continuously, providing a clear picture of when a person’s mobility begins to decline.
- A significant reduction in walking speed could trigger alerts for preventive measures, such as physical therapy or balance training.
- Changes in Stance and Swing Phases:
- The stance phase (when the foot is on the ground) and the swing phase (when the foot moves forward) are critical components of walking. A higher stance-to-swing ratio can indicate a cautious walking style due to fear of falling, which ironically increases fall risk.
- Key Indicators:
- Increased Stance Time: Spending more time with both feet on the ground can reflect an attempt to maintain balance but may lead to instability when transitioning to the swing phase.
- Asymmetrical Swing Phase: Uneven leg movements can signal a decline in coordination and strength, heightening the risk of falls.
- AI’s Role in Gait Analysis:
- AI algorithms can detect subtle changes in these gait parameters, often before they become visible to healthcare providers. This early detection enables preemptive actions, such as adjusting medication, implementing balance exercises, or even modifying the living environment to reduce fall hazards.
By monitoring these gait changes, wearable sensors equipped with AI can provide a comprehensive assessment of an individual’s fall risk, offering invaluable support to caregivers and clinicians in preventing falls before they occur.
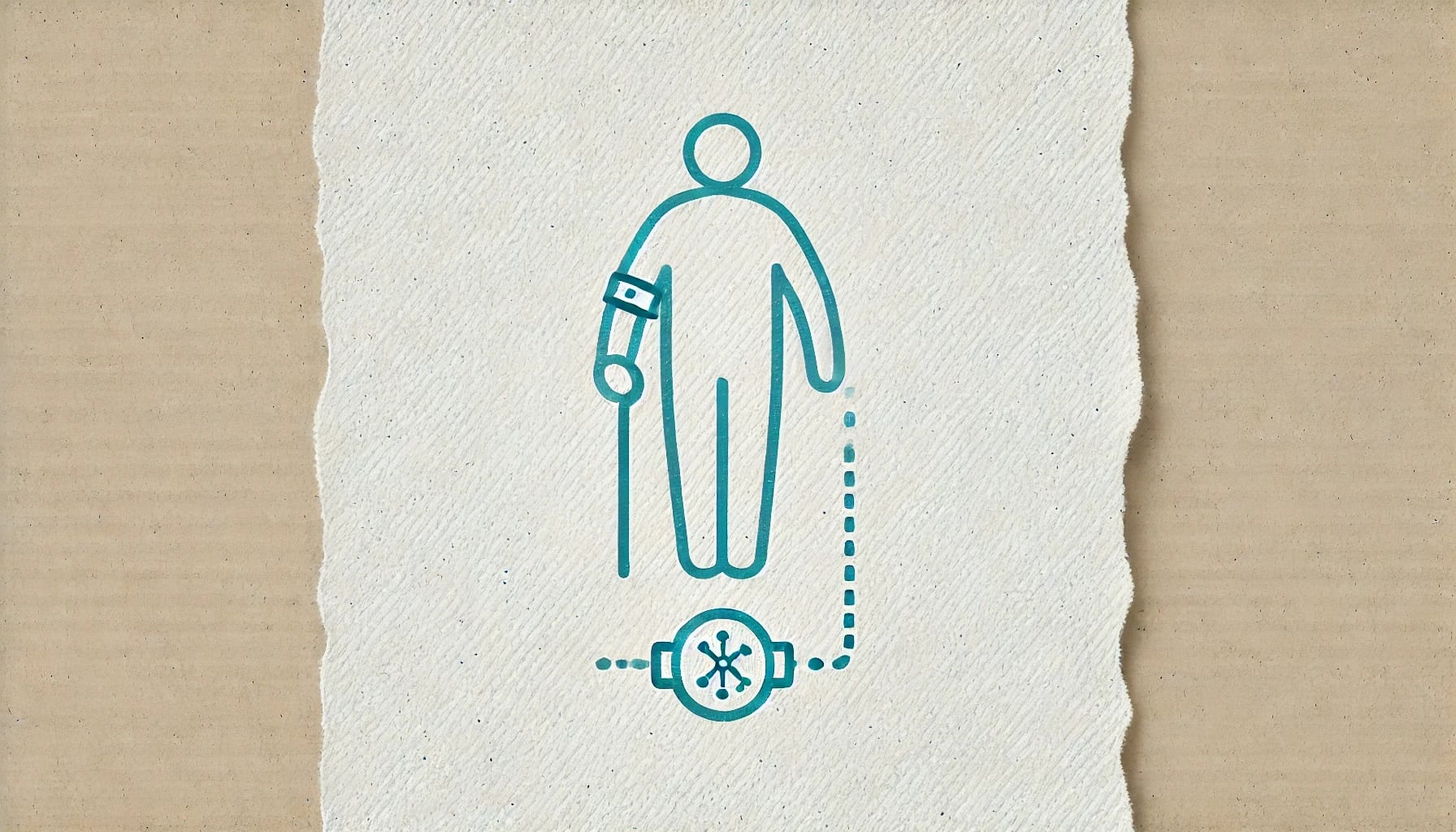
5. Wearing Locations of Accelerometers and Their Impact on Fall Risk and Fall Detection
The placement of accelerometers significantly affects their ability to detect falls and assess fall risk. Different locations on the body provide distinct insights into movement, influencing the accuracy and specificity of the data collected. Here’s how various placements impact fall detection:
- Thigh:
- Devices: For example, Fibion SENS and Fibion Research are designed for thigh placement.
- Impact on Detection: Thigh placement allows for precise tracking of lower limb movements, including stride length, stance, and swing phases. This location is particularly effective for analyzing detailed gait characteristics, which are crucial for identifying fall risks related to leg instability. It provides comprehensive insights into walking patterns, making it one of the most accurate placements for fall risk prediction.
- Center of Mass (Waist/Hip):
- Devices: For example, Fibion SENS and Fibion Research can also be worn on the hip.
- Impact on Detection: Positioned at the body’s center of mass, this placement provides a balanced overview of overall body movement and general activity levels. It is particularly useful for detecting shifts in balance and whole-body dynamics. However, while it captures broad movement patterns, it may lack the granularity needed for specific limb movements, making it slightly less precise for detailed gait analysis compared to thigh placement.

- Chest:
- Devices: For example, Fibion Vitals is designed for chest placement.
- Impact on Detection: Chest placement captures multi-signal data, including upper body movements, respiration, and heart rate. This position is advantageous for detecting falls that involve upper body imbalance or abrupt tilting. It provides a comprehensive view of a person’s upper body dynamics, but may not capture the fine details of lower limb movements essential for precise fall detection related to leg instability.
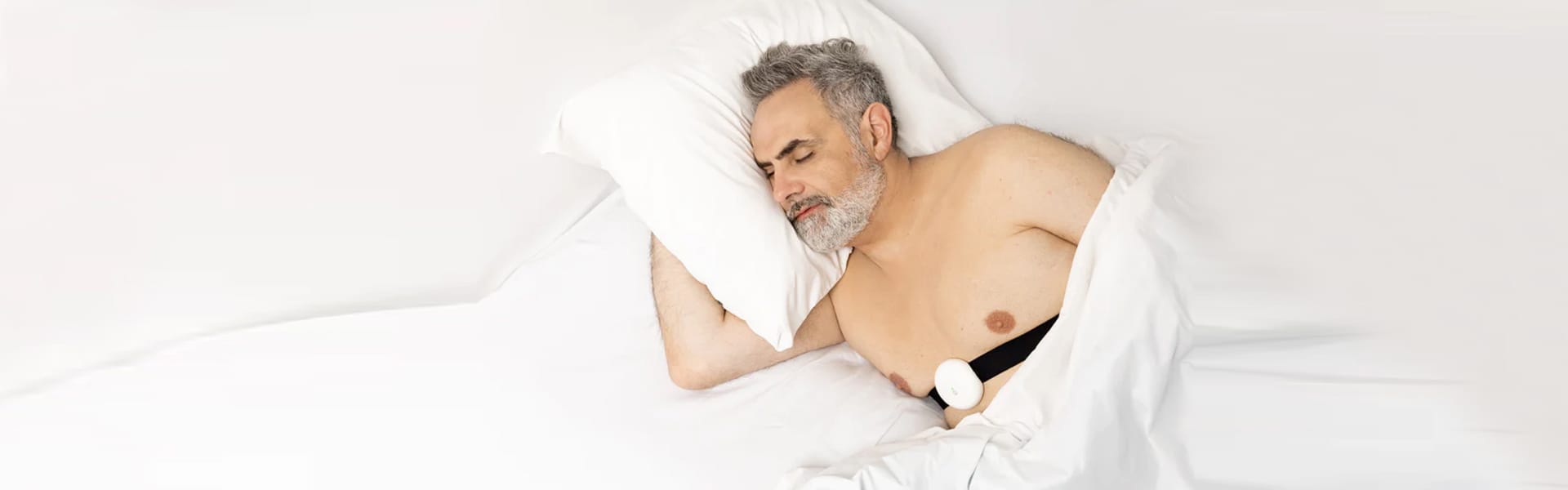
- Wrist:
- Devices: Commonly used consumer fitness trackers, such as smartwatches, are worn on the wrist.
- Impact on Detection: Wrist-worn devices are highly popular for their convenience and ease of use. They effectively track general activity levels, hand movements, and daily routines. However, wrist placement is less ideal for detailed fall detection, as it primarily captures arm movements, which may not accurately reflect whole-body stability or lower limb dynamics critical to predicting falls. Wrist-worn devices are best used as supplementary tools rather than primary fall detectors.
- Choosing the Right Placement:
- The optimal accelerometer placement depends on the specific needs of the user and the goals of the fall prevention strategy. For detailed gait analysis and fall risk related to leg movements, thigh placement is typically the most informative. For broader balance and body movement monitoring, hip or chest placements are valuable. Wrist-worn devices offer convenience and general activity tracking but should be complemented by other placements for comprehensive fall detection.
Selecting the right device and placement is key to maximizing the potential of wearable sensors in fall prevention. For more guidance on choosing the appropriate accelerometer, refer to our detailed guide on choosing the right accelerometer for your research.
By understanding the implications of device placement, researchers and clinicians can design targeted fall prevention interventions, enhancing the safety and independence of elderly individuals at risk of falls.
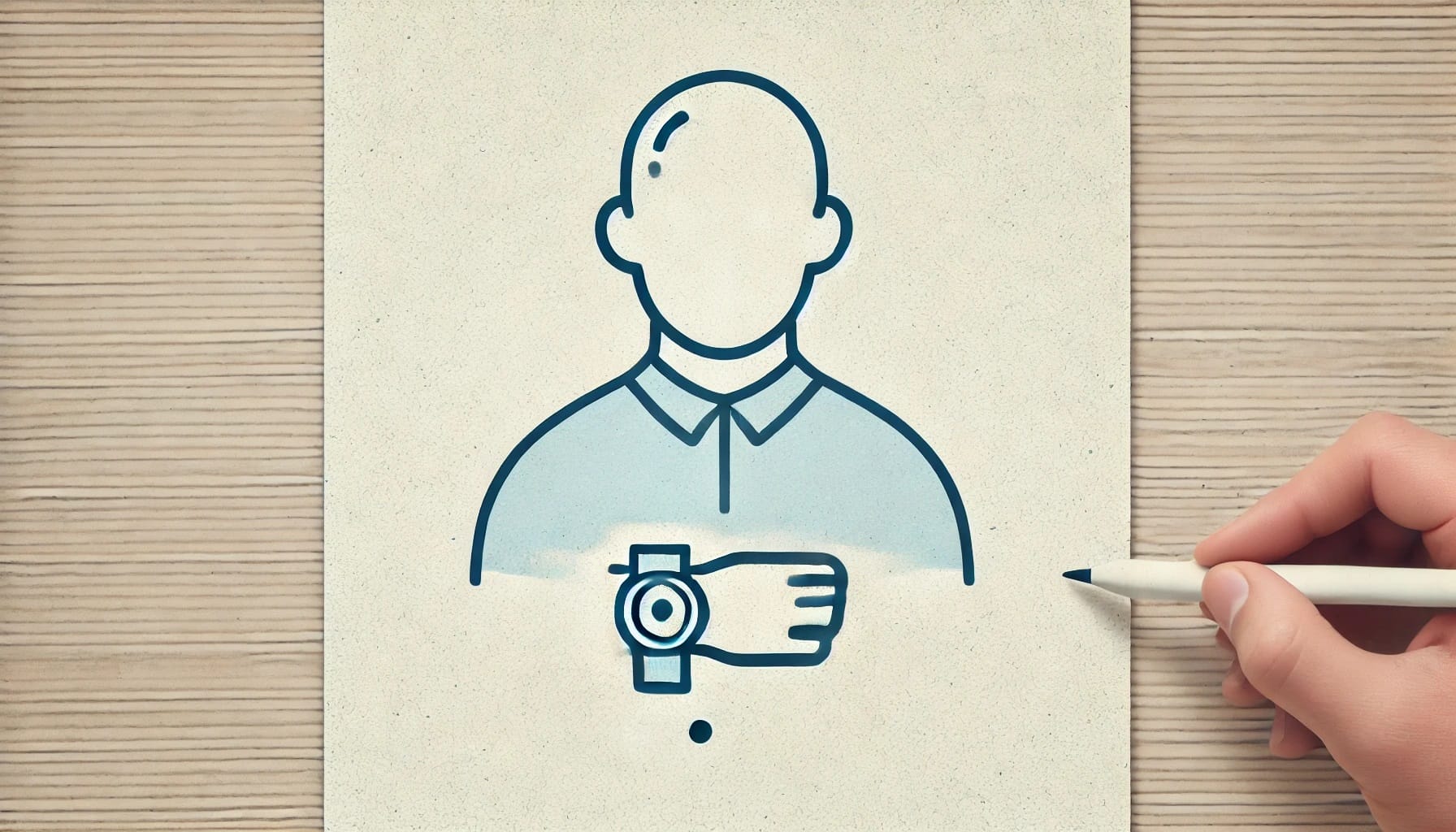
6. Real-World Applications and Research Evidence
The integration of AI-enhanced wearable sensors in fall prevention strategies has shown promising results in real-world applications. Various studies and pilot programs have demonstrated how these technologies can effectively reduce fall risks and improve safety among the elderly.
- Pilot Programs and Studies:
- Fall Prevention in Assisted Living Facilities: Several assisted living facilities have implemented wearable sensors to monitor residents’ movement patterns. AI algorithms analyze the data to identify individuals at high risk of falls, enabling staff to intervene proactively with personalized care plans, such as targeted exercise routines and balance training.
- Remote Monitoring in Home Settings: Wearable devices allow for continuous, remote monitoring of elderly individuals living independently. This approach not only enhances safety by providing immediate alerts to caregivers or family members when a fall is detected but also offers peace of mind to the elderly, knowing that help is always available.
- Clinical Trials and Research Studies: Research has shown that AI-powered wearables can reduce the incidence of falls by identifying subtle changes in gait or balance that may precede a fall. For instance, studies have found that early detection of slow walking speeds or altered gait patterns can lead to timely interventions, such as physical therapy or adjustments in medication, significantly lowering fall risks.
- Benefits Observed:
- Reduced Fall Rates: Implementing wearable sensors has led to a measurable decrease in fall rates among elderly users, particularly in environments where continuous monitoring and rapid response are critical.
- Improved Response Times: Real-time alerts generated by AI systems ensure that help can be dispatched immediately when a fall occurs, reducing the time an individual may spend on the ground, which is crucial for preventing complications from falls.
- Enhanced User Confidence: Knowing they are being monitored, elderly individuals often feel more secure in their movements, which can reduce the fear of falling—a common psychological barrier that can lead to reduced physical activity and further decline in mobility.
These real-world applications underscore the potential of AI-enhanced wearable technology in transforming fall prevention strategies, making them more effective and responsive to the needs of elderly populations.
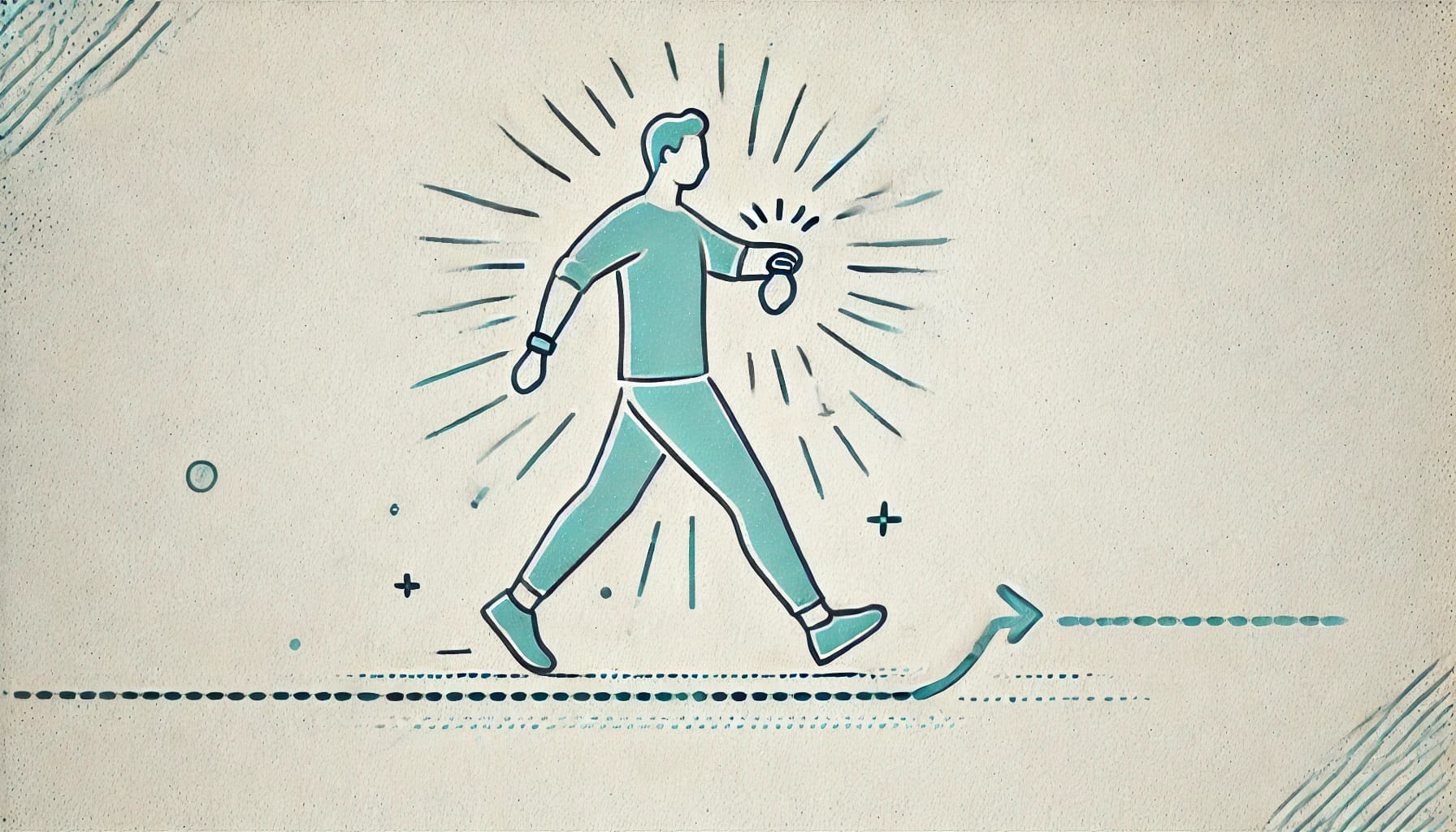
7. Advantages of AI-Integrated Wearables for Elderly Care
AI-integrated wearables offer a range of benefits that go beyond traditional fall prevention methods, making them an invaluable tool in elderly care.
- Continuous and Non-Intrusive Monitoring: Wearable sensors provide around-the-clock monitoring without interfering with daily activities. Unlike periodic check-ins or manual assessments, these devices offer continuous data collection, ensuring that no significant changes in movement patterns are missed.
- Actionable Insights and Personalized Care: AI algorithms analyze the data collected to provide actionable insights, which can be used to tailor interventions to the specific needs of each individual. For example, if a wearable detects a decline in balance, it might suggest balance-enhancing exercises or adjustments in daily routines to reduce fall risks.
- Enhanced Data for Caregivers and Healthcare Providers: The detailed data collected by wearables helps caregivers and healthcare providers make informed decisions about the care plan. This data-driven approach leads to better outcomes by enabling targeted interventions based on the specific movement patterns and risks identified.
- Ease of Use and Comfort: Modern wearables are designed with user comfort in mind. Devices like Fibion SENS and Fibion Vitals are lightweight, discreet, and easy to wear, ensuring that users do not feel burdened or inconvenienced by the technology. This ease of use is crucial for ensuring compliance and maximizing the effectiveness of fall prevention strategies.
AI-integrated wearables not only enhance safety but also empower elderly individuals to maintain their independence by providing continuous support that adapts to their changing needs.
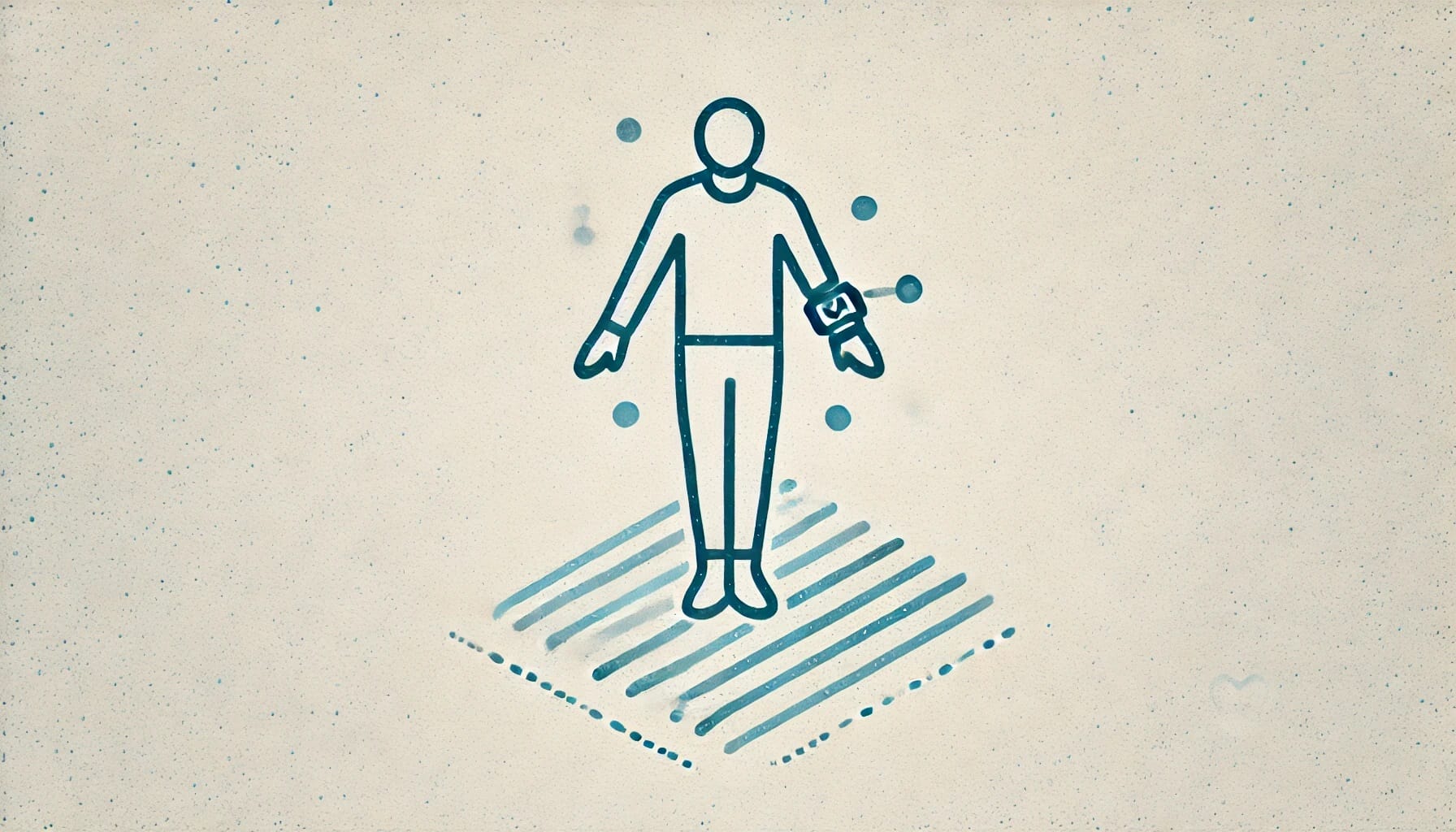
8. Challenges and Considerations
While AI-powered wearable sensors hold significant promise for fall prevention, several challenges and considerations must be addressed to ensure their effectiveness and widespread adoption.
- Data Privacy and Security: As with any technology that collects personal health data, ensuring the privacy and security of this information is paramount. Wearable devices must adhere to stringent data protection regulations, and users need to feel confident that their data is handled securely.
- User Training and Technology Adoption: Elderly users may face challenges in adopting new technologies. It is important to provide adequate training and support to ensure that users can operate the devices comfortably and understand the data being presented to them. Simplified interfaces and clear instructions can help bridge the technology gap.
- Accuracy and Reliability: Ensuring the accuracy of the data collected by wearable sensors is critical. False positives or negatives in fall detection can lead to unnecessary alarm or missed opportunities for intervention. Continuous validation and improvement of AI algorithms are necessary to maintain high levels of reliability. For further insights on this topic, refer to our article on validating wearable device data.
- Cost and Accessibility: While the cost of wearable technology has been decreasing, affordability remains a concern, particularly for widespread use among the elderly. Strategies to make these devices more accessible, including potential subsidies or integration into broader healthcare programs, could enhance their reach and impact.
Addressing these challenges is crucial to realizing the full potential of wearable sensors and AI in preventing falls and supporting the health and independence of elderly individuals.
9. Future Directions
The future of wearable sensors and AI in elderly care looks promising, with continuous advancements in technology poised to further enhance their capabilities.
- Advancements in Predictive Analytics: Future developments in AI could lead to even more sophisticated predictive models that not only detect fall risks but also offer comprehensive insights into overall health trends, potentially predicting other health issues before they arise.
- Integration with Broader Health Systems: Integrating wearable sensor data with electronic health records (EHRs) and broader healthcare systems could enable more holistic care approaches, allowing healthcare providers to have a more complete picture of a patient’s health status.
- Enhanced Personalization: As AI algorithms become more advanced, wearable sensors will be able to offer highly personalized recommendations that are tailored to the unique movement patterns, health conditions, and lifestyles of each individual user.
- Increased Adoption in Diverse Settings: Beyond individual use, the adoption of wearable sensors in hospitals, rehabilitation centers, and assisted living facilities is likely to increase, offering a scalable solution to monitor and protect larger populations at risk of falls.
Continued research and innovation in wearable technology and AI will play a pivotal role in shaping the future of elderly care, making fall prevention smarter, more efficient, and more personalized.
Conclusion
Wearable sensors powered by AI represent a groundbreaking approach to fall prevention in elderly populations. By providing continuous monitoring, early detection of fall risks, and personalized interventions, these technologies offer a proactive solution to a problem that affects millions worldwide. As the technology continues to evolve, the potential for AI-enhanced wearables to improve safety, independence, and quality of life for elderly individuals will only grow. Encouraging the exploration and adoption of these innovative tools is a crucial step toward a safer and healthier future for our aging population.
Additional Resources
Explore more about wearable technology and fall prevention in our wearables article collection and accelerometer article collection, offering further insights into the advancements and applications of these transformative tools.
Call to Action
📅 If you want to learn more about Fibion SENS, Research and Vitals for fall risk and fall detection, do not hesitate to book a video call with our expert Dr. Miriam Cabrita.
🔍 Order Fibion SENS Motion 3 Devices Test Package to get first-hand experience of the system. Order now for hands-on experience and comprehensive insights.
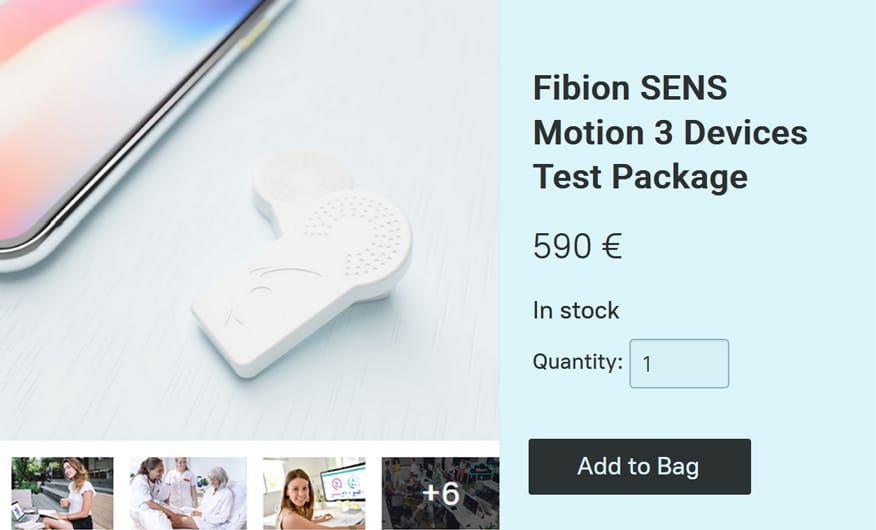
🔍 You may also discover other valid and reliable products in our portfolio, such as the Fibion Sleep, Fibion Emfit, and Fibion Circadian, all designed to assist in research measuring physical activity, sedentary behavior, and sleep.
Frequently asked questions about this topic:
How do wearable sensors help prevent falls in the elderly? +
Wearable sensors monitor movement and physiological data to detect fall risks, allowing for early intervention. They provide real-time alerts and personalized feedback to reduce the likelihood of falls.
What types of sensors are commonly used in wearable devices for fall prevention? +
Common sensors include accelerometers, gyroscopes, and pressure sensors. Accelerometers detect movement, gyroscopes track orientation, and pressure sensors monitor weight distribution, all of which contribute to fall risk assessment.
How does artificial intelligence enhance the effectiveness of wearable sensors? +
AI processes the data collected by wearable sensors to identify patterns and predict fall risks. It uses machine learning algorithms to provide real-time insights and personalized interventions based on the user’s movement patterns.
What are the benefits of using AI-driven wearables for elderly fall prevention? +
AI-driven wearables offer continuous monitoring, early detection of fall risks, and tailored feedback, enhancing safety and independence. They provide caregivers with actionable insights to improve elderly care.
Which wearable sensor placements are most effective for fall detection? +
Thigh placement is highly effective for detailed gait analysis, while hip and chest placements provide broader movement monitoring. Wrist-worn devices are convenient but less precise for fall detection.
What challenges exist in implementing AI-powered wearable sensors for fall prevention? +
Challenges include data privacy, user training, and ensuring the accuracy of fall detection algorithms. Cost and accessibility can also be barriers, requiring strategies to improve affordability and user adoption.