1. Introduction
In this article, we will share insights on how researchers are using artificial intelligence (AI) to advance sleep research. AI technologies are opening new opportunities for analyzing sleep patterns, diagnosing sleep disorders, and improving overall sleep quality. Below are four compelling examples of AI applications in sleep studies.
AI’s contribution to sleep research is impressive, involving the processing of vast amounts of data from various sensors and devices. These algorithms can:
- Map Sleep Stages: Accurately outline sleep stages and cycles.
- Detect Anomalies: Identify disorders such as sleep apnea.
- Provide Personalized Insights: Offer tailored insights into sleep quality.
These capabilities are crucial for developing effective interventions and enhancing sleep health.
“Advanced AI algorithms can map out sleep stages with remarkable accuracy, paving the way for better sleep health insights.”
2. Real-World Applications and Scientific Studies
2.1. Machine Learning Assisted Wearable Wireless Device for Sleep Apnea Syndrome Diagnosis
Study: Wang et al. (2023)
Outcome: This study developed a small, low-cost wearable device using photoplethysmography (PPG) optical sensors to diagnose sleep apnea syndrome (SAS). The device employs multiscale entropy and random forest algorithms to analyze PPG signals and blood oxygen saturation, achieving over 85% accuracy compared to traditional polysomnography (PSG) systems.
Significance: This wearable device offers a feasible and cost-effective solution for the portable and accurate screening and diagnosis of SAS, enhancing early detection and intervention.
2.2. Deep Learning-Based Sleep Stage Classification
Study: Morokuma et al. (2023)
Outcome: This study assessed the performance of a deep learning-based model using cardiorespiratory and body movement data to classify sleep stages in individuals with suspected sleep disorders. The model achieved an accuracy of 71.2% and showed substantial correlations with polysomnography-derived sleep parameters.
Significance: The findings demonstrate the efficacy of the biLSTM model for accurately classifying sleep stages, offering a less obtrusive and potentially in-home monitoring solution for sleep disorder diagnosis.
2.3. Effectiveness of an App-Based Short Intervention to Improve Sleep
Study: Vollert et al. (2023)
Outcome: This study evaluated the effectiveness of an app-based short intervention called Refresh, designed to improve sleep quality. The intervention, which is based on the principles of cognitive behavioral therapy for insomnia (CBT-I), was compared with a waitlist control group. The primary outcome was sleep quality, measured by the Regensburg Insomnia Scale (RIS). The intervention showed a significant improvement in insomnia symptoms, particularly among participants with poor sleep quality.
Significance: The findings suggest that app-based interventions can effectively improve sleep quality, offering a scalable solution for individuals experiencing insomnia.
2.4. Using Machine Learning to Predict Sleep-Disordered Breathing Diagnosis
Study: Cokim and Ghaly (2023)
Outcome: This study utilized machine learning algorithms to predict the presence of sleep-disordered breathing (SDB) based on patients’ medical comorbidities and craniofacial features. The research involved 69 adult patients and applied logistic regression, k-nearest neighbors, support vector machines, and naive Bayes models. The logistic regression model performed the best, achieving an accuracy of 86%, with 100% specificity and 77.8% sensitivity.
Significance: The study demonstrated that machine learning models could effectively predict sleep-disordered breathing using non-invasive measures, which could lead to a more accessible and earlier diagnosis of SDB.
“Machine learning models are transforming how we detect and manage sleep disorders, offering new hope for improved sleep health.”
3. Conclusion
AI is making significant strides in sleep research by providing detailed analyses and insights into sleep patterns and disorders. These technological advancements support the development of tailored interventions and enhance our understanding of sleep health. As AI continues to evolve, its role in sleep research will expand, offering even more sophisticated tools for improving sleep quality and health outcomes.
References
- Wang, S., Xuan, W., Chen, D., Gu, Y., Liu, F., Chen, J., Xia, S., Dong, S., & Luo, J. (2023). Machine Learning Assisted Wearable Wireless Device for Sleep Apnea Syndrome Diagnosis. Biosensors, 13(4), 483. Read more
- Morokuma, S., Hayashi, T., Kanegae, M., Mizukami, Y., Asano, S., Kimura, I., Tateizumi, Y., Ueno, H., Ikeda, S., & Niizeki, K. (2023). Deep learning-based sleep stage classification with cardiorespiratory and body movement activities in individuals with suspected sleep disorders. Scientific Reports, 13, 17730. Read more
- Vollert, B., Müller, L., Jacobi, C., Trockel, M., & Beintner, I. (2023). Effectiveness of an App-Based Short Intervention to Improve Sleep: Randomized Controlled Trial. JMIR Mental Health, 10, e39052. Read more
- Cokim, S., & Ghaly, J. (2023). Using Machine-Learning to Predict Sleep-Disordered Breathing Diagnosis From Medical Comorbidities and Craniofacial Features. Cureus, 15(5), e39798. Read more
For further insights into AI’s impact on sleep research, check out our comprehensive sleep article collection here.
Call to action
🚀📊 Learn more about how AI and wearable sensors like Fibion Vitals provide comprehensive insights into physical activity, sedentary behavior, and sleep research.
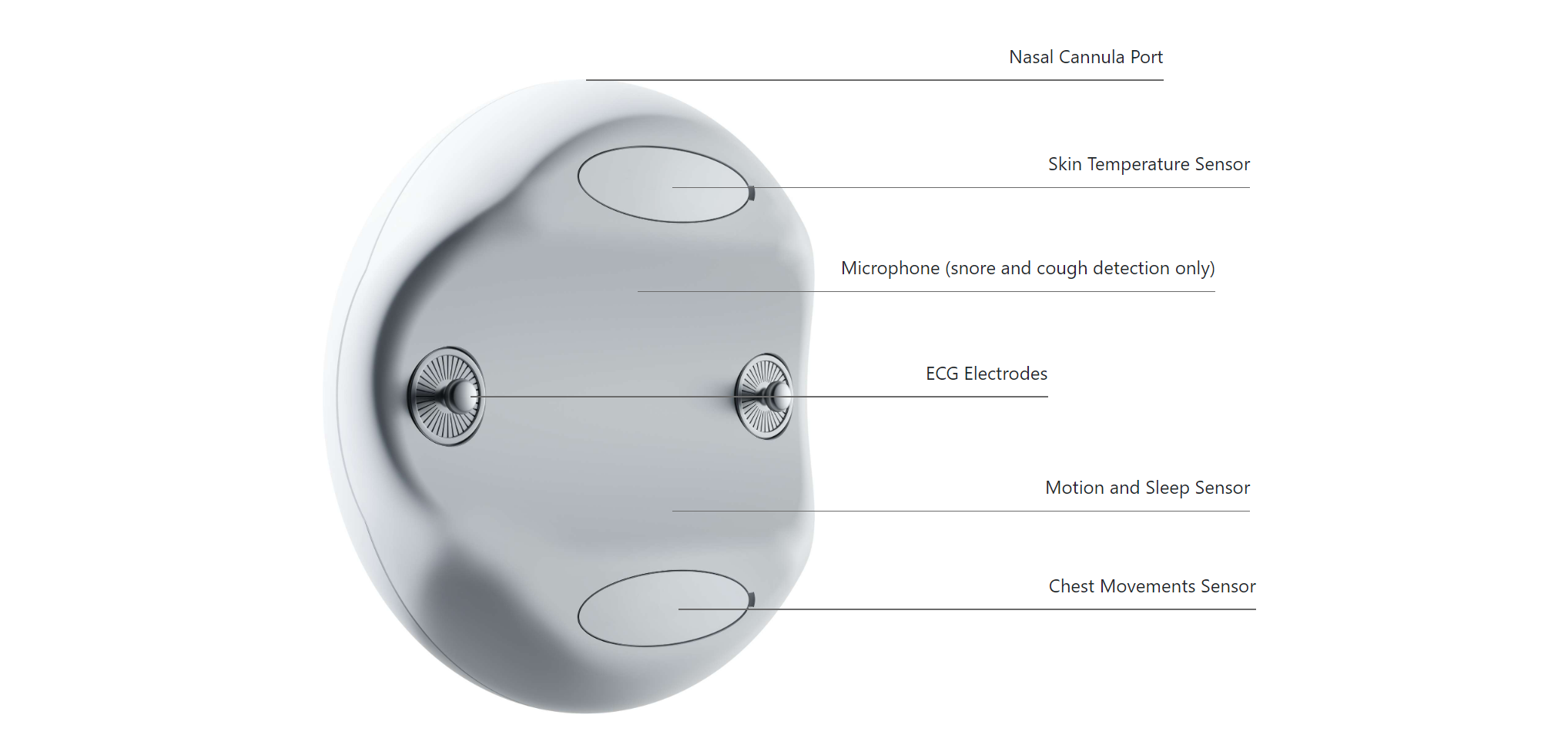
📅 If you want to learn more about Fibion Vitals, do not hesitate to book a video call with our expert Dr. Miriam Cabrita.
🔍 You may also discover other valid and reliable products in our portfolio, such as the Fibion Sens, Fibion Device, Fibion Sleep, Fibion Mimove, Fibion Emfit, and Fibion Circadian, all designed to assist in research measuring physical activity, sedentary behavior, and sleep.
Frequently asked questions:
How does AI contribute to sleep research? +
AI processes vast amounts of data from sensors and devices to map sleep stages, detect anomalies like sleep apnea, and provide personalized insights into sleep quality. These capabilities help develop effective interventions and enhance sleep health.
How does AI map sleep stages? +
AI maps sleep stages by analyzing data from polysomnography and other sensors. Advanced algorithms accurately outline sleep stages and cycles, providing detailed insights into sleep architecture.
Can AI detect sleep disorders? +
Yes, AI can detect sleep disorders such as sleep apnea by processing data from wearable sensors. Machine learning algorithms identify anomalies in sleep patterns, facilitating early diagnosis and treatment.
What are some real-world applications of AI in sleep research? +
AI has been used to detect sleep apnea, analyze sleep stages with neural networks, develop mobile apps for sleep improvement, and create predictive models for sleep disorders. These applications enhance our understanding of sleep health and aid in developing targeted interventions.
How do AI-powered mobile apps improve sleep quality? +
AI-powered mobile apps monitor sleep patterns and offer personalized recommendations for better sleep. These apps help users make informed adjustments to their sleep habits, promoting better sleep quality and overall health.
What future advancements can be expected from AI in sleep research? +
As AI technology continues to evolve, its role in sleep research will expand. Future advancements may include more sophisticated tools for analyzing sleep patterns and disorders, leading to improved sleep quality and health outcomes.