Introduction
Efficient data management is crucial in physical activity research. How can modern technology streamline this process and improve the accuracy of our findings?
Large language models (LLMs) offer a promising solution. These advanced tools can automate data collection, cleaning, and integration, providing researchers with more accurate and comprehensive insights. In this article, we explore how LLMs can enhance data management in physical activity studies, focusing on their ability to handle large and diverse datasets efficiently.

The Importance of Efficient Data Management in Physical Activity Studies
Data Volume and Variety
Physical activity research involves collecting vast amounts of data from various sources. This can include:
- Sensor Data: Information from wearable devices tracking movement, heart rate, and other biometrics.
- Self-Reports: Participant logs detailing their daily activities, exercise routines, and perceived exertion.
- Environmental Data: Contextual information such as weather conditions, temperature, and light exposure.
Managing these diverse data types presents a significant challenge. Traditional methods often struggle with the sheer volume of data, leading to potential errors and inconsistencies.
Traditional data management methods are often labor-intensive and prone to errors. Manual data entry and cleaning can be time-consuming, and integrating data from multiple sources can be complex. These challenges can lead to delays and inaccuracies, ultimately affecting the quality of the research outcomes.
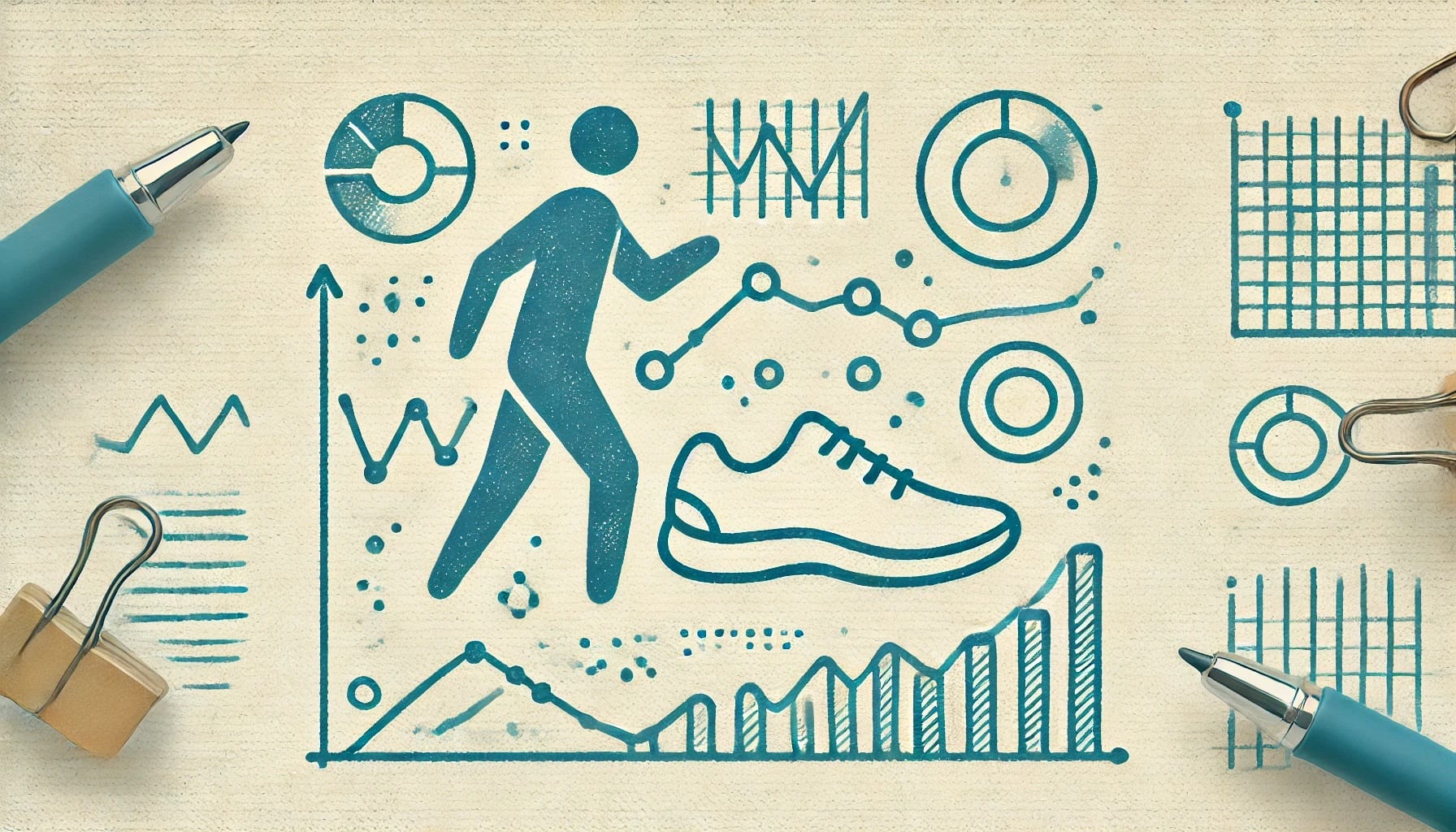
How LLMs Enhance Data Management
- Automated Data Collection: LLMs can automate the collection of real-time data from various sources, significantly improving the efficiency of this process. By using wearables and mobile apps, researchers can continuously capture accurate and comprehensive physical activity data without manual intervention. Example: Continuous data capture allows for precise tracking of physical activity patterns throughout the day, providing a more detailed picture of participant behavior.
- Data Cleaning and Standardization: LLMs are capable of automatically cleaning and standardizing data, reducing the likelihood of errors and inconsistencies. This involves handling missing data, correcting anomalies, and ensuring that all data is in a uniform format. Example: AI can detect and correct discrepancies in self-reported activity logs and sensor data, ensuring that all data points are accurate and comparable.
- Data Integration: One of the most powerful features of LLMs is their ability to integrate data from different sources. By combining biometric sensors, self-reported activity logs, and environmental data, LLMs provide a holistic view of an individual’s physical activity patterns. Example: Integrating data from wearables, sleep diaries, and weather conditions can reveal how external factors influence physical activity and overall health.
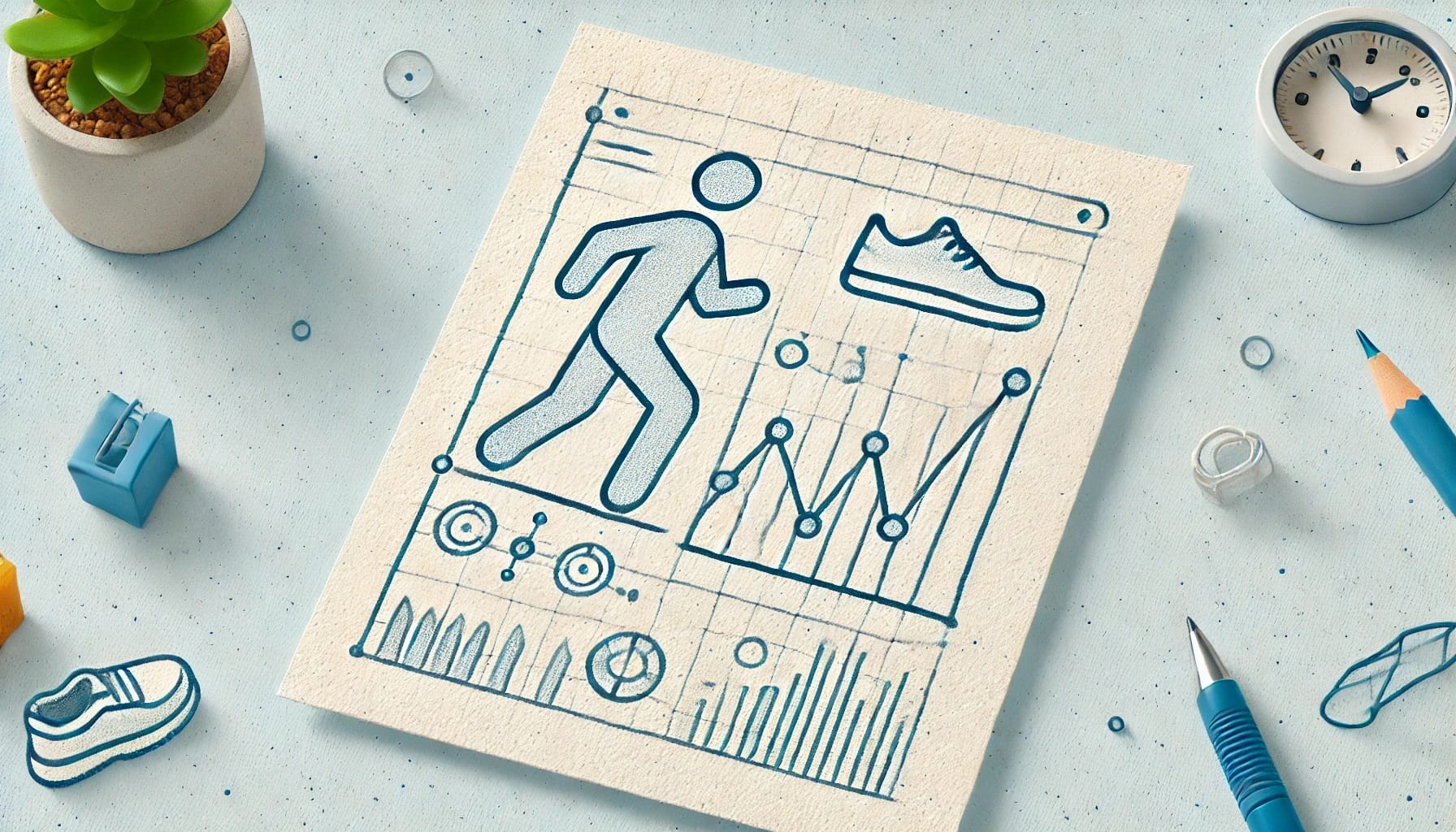
Practical Applications in Physical Activity Studies
Enhanced Data Analysis
LLMs excel at identifying patterns and trends in large datasets. In physical activity research, this ability is crucial for understanding how different factors influence behavior.
- Pattern Recognition: LLMs can analyze data to identify daily, weekly, and seasonal activity patterns. For example, recognizing that participants are more active on weekends can help tailor intervention strategies. Example: AI can analyze data from wearables and self-reported logs to determine the most effective times for physical activity interventions, increasing adherence and overall effectiveness.
- Predictive Modeling; LLMs can also create predictive models to forecast health outcomes based on historical data. This capability allows researchers to anticipate future behavior and health risks, leading to more proactive health management.
- Forecasting Health Outcomes: By analyzing past activity levels and integrating various data sources, LLMs can predict the likelihood of developing health issues such as obesity, cardiovascular diseases, or diabetes. Example: AI can identify individuals at risk of health problems due to insufficient physical activity, allowing for early interventions tailored to their specific needs.
- Real-Time Feedback: LLMs enable real-time data analysis and feedback, providing immediate insights and recommendations based on current activity levels.
- Immediate Interventions: Real-time analysis helps in delivering instant feedback to participants. For instance, if a wearable device detects prolonged inactivity, AI can send alerts encouraging users to move. Example: Real-time alerts can prompt users to take short walks after periods of sitting, helping to reduce sedentary behavior and promote regular physical activity throughout the day.
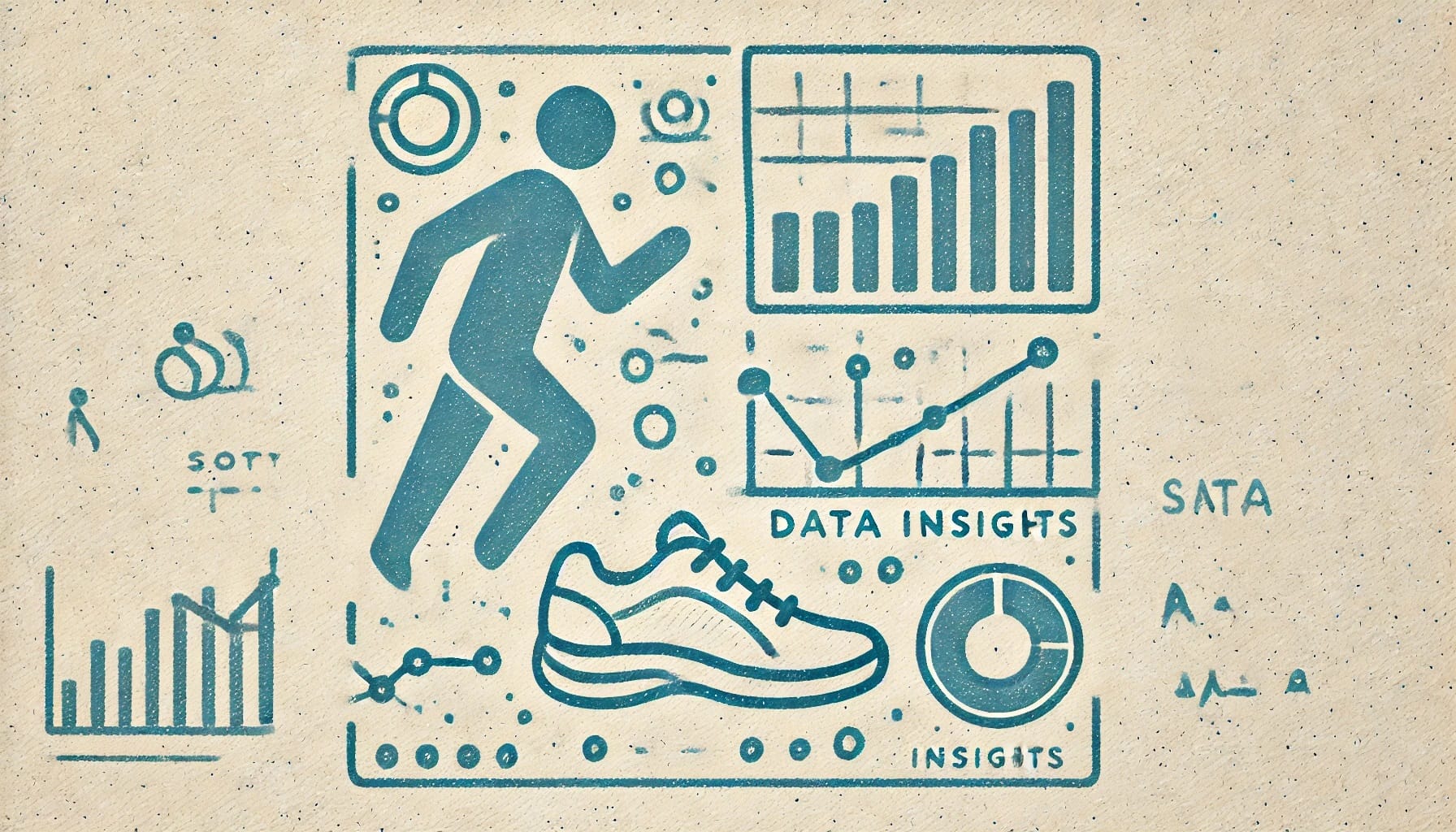
Benefits of Using LLMs for Data Management
- Efficiency: LLMs streamline data management processes, significantly reducing the time and resources needed for data collection and analysis. This efficiency allows researchers to focus more on interpreting results and developing interventions rather than on manual data processing.
- Accuracy: Automated data cleaning and standardization improve the accuracy of research data. By minimizing errors and inconsistencies, LLMs ensure that the data used for analysis is reliable and precise.
- Comprehensive Insights: Integrating diverse data sources provides a richer and more comprehensive understanding of physical activity patterns. This holistic view helps researchers identify subtle correlations and trends that might be overlooked with traditional methods.
- Combining Multiple Variables: AI can handle the integration and analysis of numerous variables simultaneously, which would be challenging and time-consuming manually. This capability allows for more complex and detailed studies, considering a wide range of factors affecting physical activity and health outcomes.
- Cost and Workload Reduction: By automating complex tasks, LLMs reduce the cost and workload for researchers. This makes it feasible to conduct large-scale studies that were previously too expensive or labor-intensive, enabling more ambitious and comprehensive research projects.

Future Directions and Implications
- Technological Advancements: As AI and LLM technology continue to evolve, their capabilities for data management will be further enhanced. Future improvements may include more sophisticated natural language processing, better integration of diverse data types, and enhanced predictive modeling.
- Broader Applications: The potential applications of these data management techniques extend beyond physical activity research. They can be applied to other areas of health research, public health monitoring, and personalized medicine, improving research quality and outcomes across various fields.
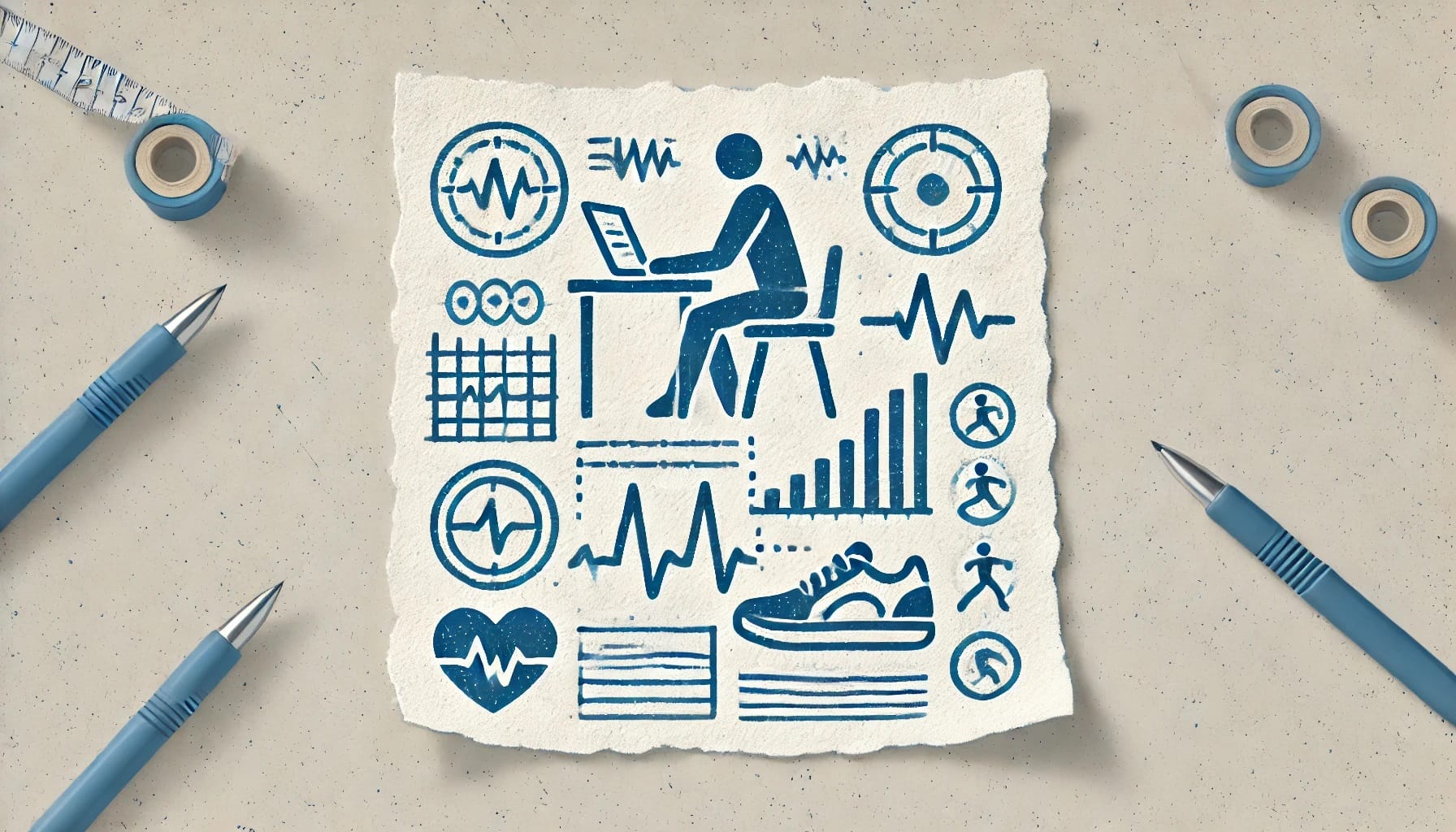
Conclusion
Large language models and AI offer transformative tools for efficient data management in physical activity studies. By automating data collection, cleaning, and integration, these technologies improve the accuracy and comprehensiveness of research data. This not only enhances the quality of research but also enables new, cost-effective study designs that were previously infeasible.
Researchers are encouraged to adopt LLMs and AI in their studies to gain deeper insights and develop more effective interventions. By leveraging these powerful tools, the field of health research can make significant strides toward improving public health and personalized medicine.
Researchers looking to explore the potential of AI and LLMs in their work should consider the following steps:
- Further Reading: Delve into academic papers and articles on the latest advancements in AI and LLM technology.
- Workshops and Training: Participate in workshops and training sessions to gain hands-on experience with these technologies.
- Collaborations: Partner with technology experts to integrate AI into your research projects.
You Might Also Be Interested
🚀📊 Learn more about how Fibion SENS can help you in your projects measuring physical activity and sedentary behavior!
🔍 Elevate your project with Fibion SENS Motion 3 Devices Test Package for precise activity analysis. Order now for hands-on experience and comprehensive insights.
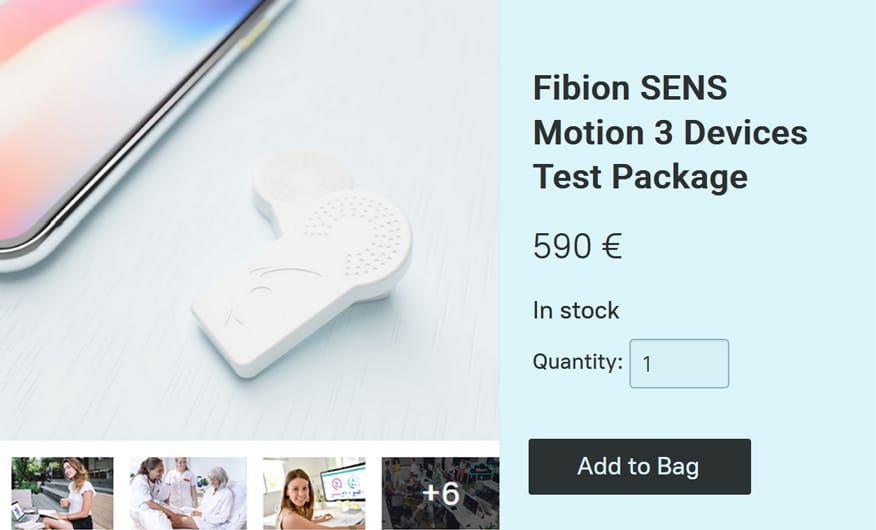
📅 If you want to learn more about Fibion SENS, do not hesitate to book a video call with our expert Dr. Miriam Cabrita.
🔍 You may also discover other valid and reliable products in our portfolio, such as the Fibion Device, Fibion Sleep, Fibion Mimove, Fibion Vitals, Fibion Emfit, and Fibion Circadian, all designed to assist in research measuring physical activity, sedentary behavior, and sleep.
Frequently Asked Questions
How do AI and LLMs improve data management in physical activity research? +
AI and LLMs automate data collection, cleaning, and integration, enhancing accuracy and efficiency. This allows researchers to process large and diverse datasets with ease.
What types of data can LLMs handle in physical activity studies? +
LLMs can handle sensor data from wearables, self-reported activity logs, environmental data, and more. This comprehensive approach provides a holistic view of physical activity patterns.
How do AI and LLMs enhance data analysis in physical activity research? +
AI and LLMs excel at identifying patterns and trends in large datasets, enabling more precise analysis and prediction of health outcomes based on physical activity data.
What are the benefits of using AI and LLMs in physical activity studies? +
AI and LLMs improve efficiency, accuracy, and provide comprehensive insights by automating data processes and integrating diverse data sources. This leads to better research outcomes and personalized health interventions.