Introduction
Understanding sleep patterns is crucial for improving overall health and well-being. How can we leverage modern technology to gain deeper insights into sleep behavior?
Large language models (LLMs) and artificial intelligence (AI) provide a valuable solution. These advanced tools can process and interpret vast amounts of data, uncovering patterns that were previously difficult to detect. In this article, we explore how LLMs and AI can enhance sleep research by integrating and analyzing diverse data sources.
Large language models are AI systems designed to understand and generate human language. They can process large datasets, identify patterns, and make predictions. AI, encompassing a broader range of technologies including machine learning and LLMs, offers powerful capabilities for data analysis.
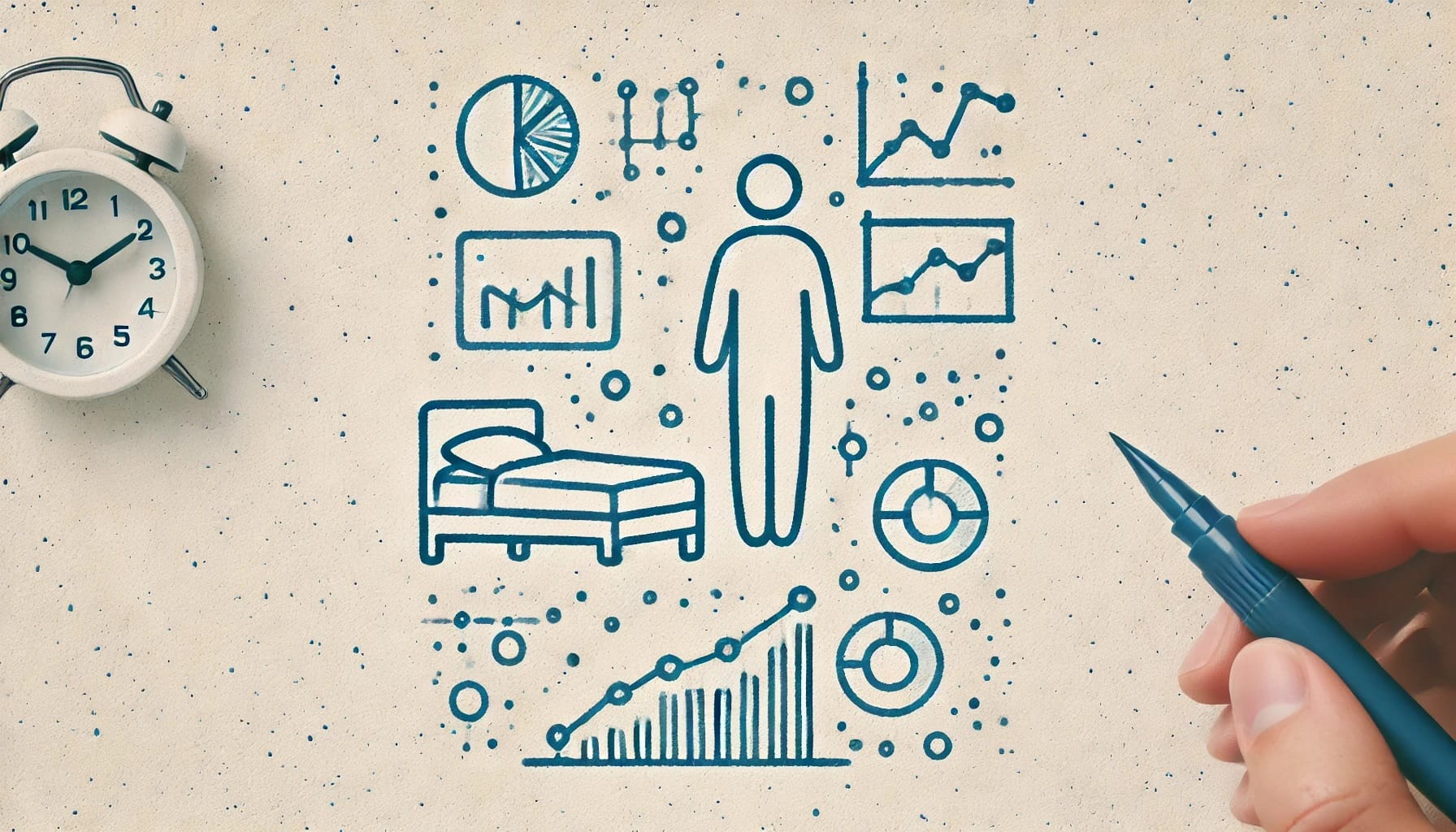
Relevance to Sleep Research
LLMs and AI are particularly useful in sleep research because they can handle and integrate various types of data. This ability to combine different data sources provides a more comprehensive understanding of sleep patterns and factors affecting sleep quality.
Sleep research involves collecting various data types, such as:
- EEG Readings: Monitoring brain activity during sleep.
- Actigraphy: Tracking movement and rest cycles using wearable devices.
- Sleep Diaries: Self-reported data on sleep habits and quality.
- Weather Data: Information on daylight exposure, which affects circadian rhythms.
- Room Temperature: Environmental conditions that influence sleep.
- Participant Photos: Images of the sleep environment to assess factors like lighting and comfort.
These are examples of the diverse data that can be collected, and many other factors can be considered.
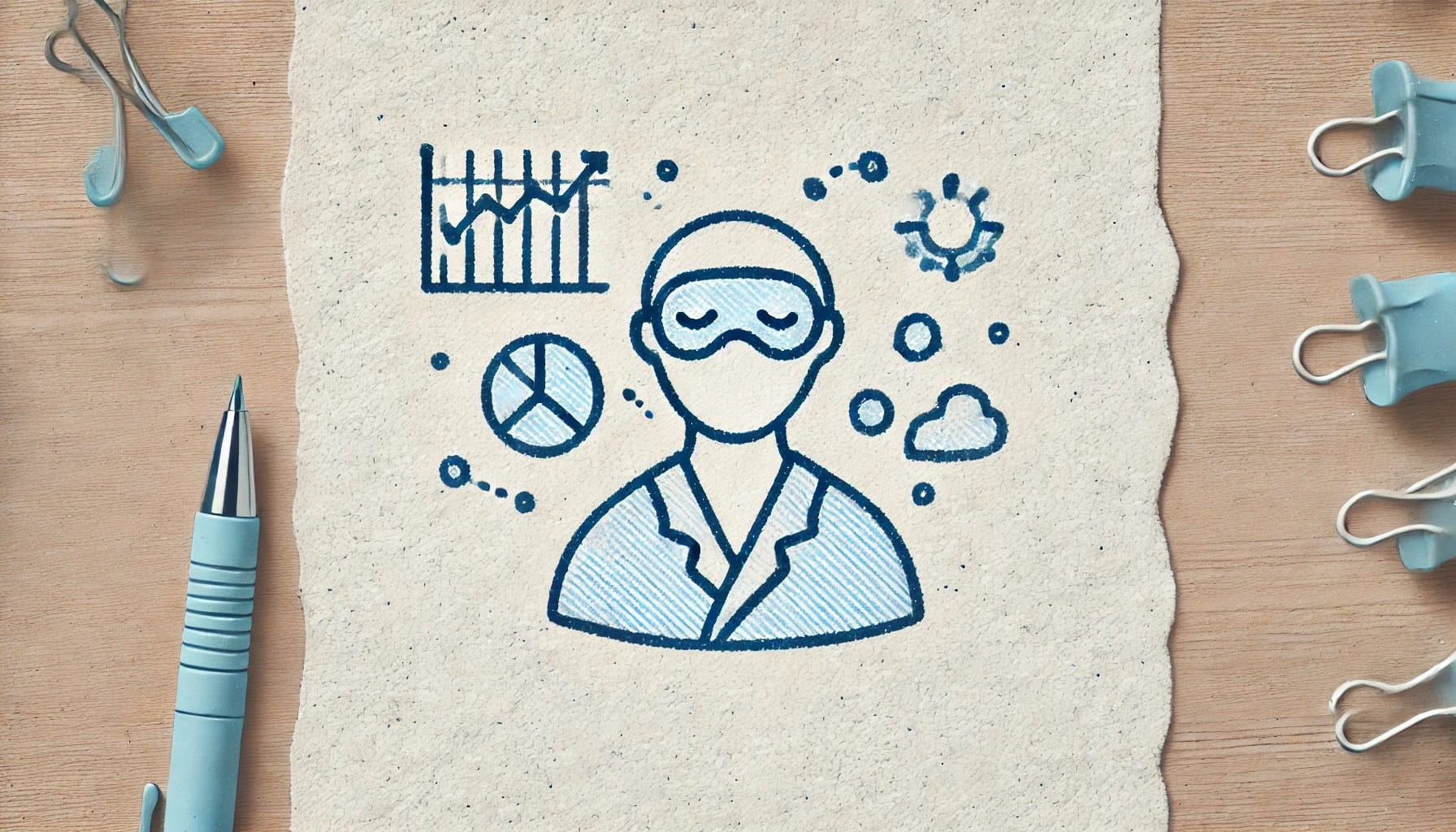
Traditional Analysis Challenges
Analyzing this diverse data manually is time-consuming and prone to errors. Integrating multiple data sources to form a cohesive understanding of sleep patterns is challenging without advanced tools. Traditional methods may miss subtle correlations and trends, limiting the insights that can be gained.
- Data Integration: LLMs can integrate various data types, such as EEG readings, actigraphy, self-reported sleep diaries, weather data, and room temperature. By combining these sources, researchers can gain a comprehensive view of sleep patterns. For example, integrating sleep diaries with EEG readings and weather data can reveal how environmental factors and personal habits influence sleep quality.
- Pattern Recognition: AI excels at identifying patterns in complex data. For instance, it can accurately determine sleep stages (e.g., REM, deep sleep) by analyzing EEG and actigraphy data. This precision helps in understanding the nuances of sleep behavior and identifying potential sleep disorders.
- Predictive Analytics: LLMs can predict the likelihood of sleep disorders based on historical data. For example, by analyzing long-term sleep patterns and environmental factors, AI can identify risks for conditions like insomnia or sleep apnea. This predictive capability allows for early intervention and better management of sleep health.
- Real-Time Monitoring: AI enables continuous data collection through wearable devices for real-time sleep monitoring. This data can be processed instantly, providing immediate feedback to participants. For example, if a wearable device detects poor sleep quality, the AI can offer suggestions to improve it right away, such as adjusting room temperature or altering bedtime routines.
- Personalized Sleep Interventions: AI can offer tailored recommendations to enhance sleep quality. By analyzing individual sleep patterns, environmental factors, and lifestyle habits, AI can provide personalized advice. For instance, it can suggest specific sleep hygiene practices, such as optimal bedtimes, relaxation techniques, or changes in the sleep environment to improve overall sleep quality.
- Environmental Factors: AI can also incorporate various environmental data to understand their effects on sleep. This includes room temperature, weather conditions, and participant photos of their sleep environment. By analyzing this data, AI can identify factors that negatively impact sleep and provide strategies to mitigate these issues.
- Longitudinal Studies: LLMs are valuable in analyzing long-term sleep data to track changes and trends over time. This helps researchers understand the progression of sleep disorders or the long-term impact of interventions. For example, AI can analyze data collected over several months to determine how changes in sleep habits or environment affect sleep quality.
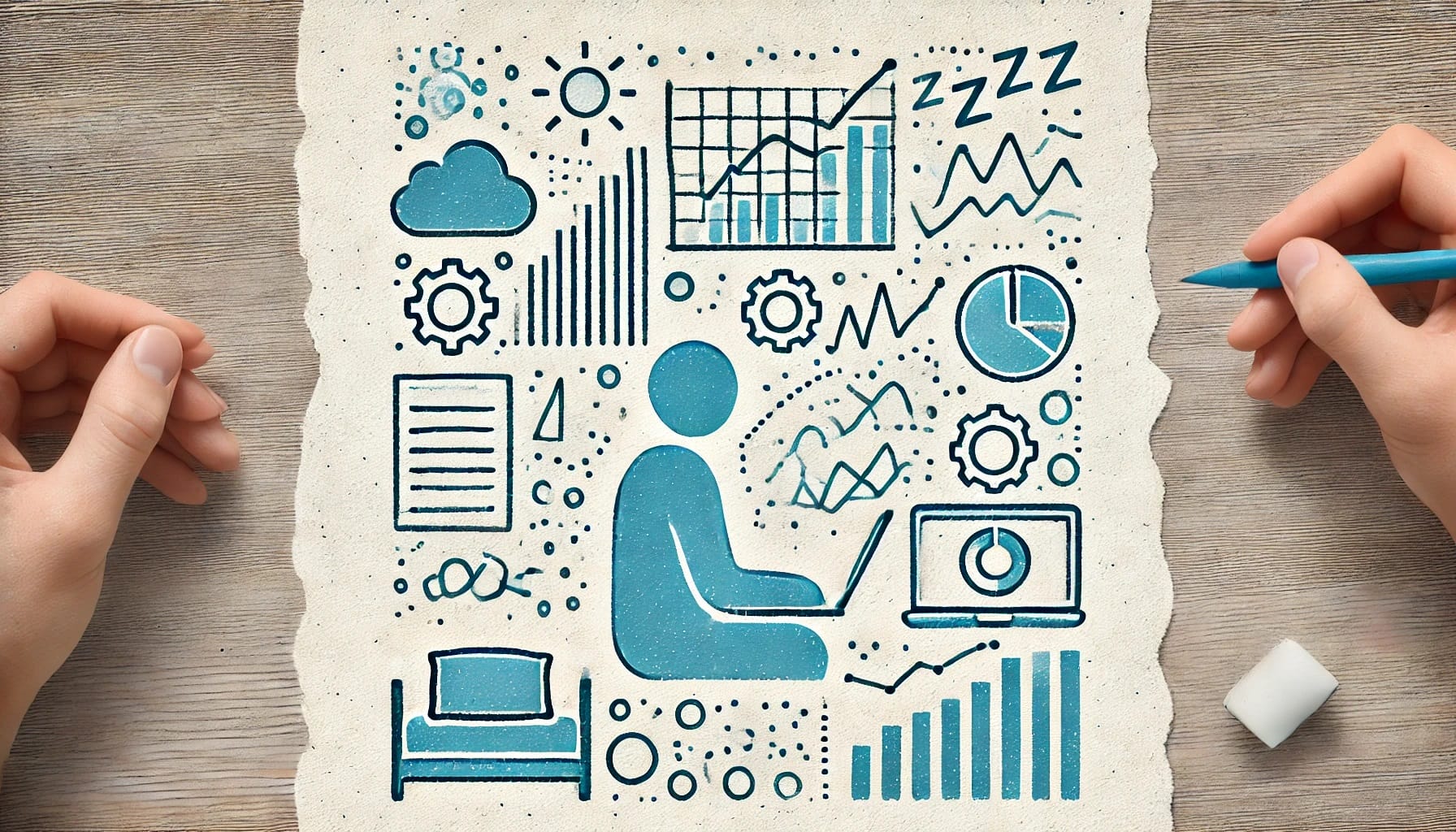
Benefits of Using LLMs and AI in Sleep Research
- Enhanced Accuracy: AI improves the accuracy of sleep data analysis by integrating diverse data types and identifying subtle patterns that traditional methods might miss.
- Efficiency: AI automates the data processing, reducing the time and effort required for manual analysis. This efficiency allows researchers to focus on interpreting results and developing interventions.
- Deeper Insights: By combining multiple variables and large datasets, AI provides nuanced insights into sleep behavior and the factors affecting it. This comprehensive analysis enables more effective and personalized sleep interventions.
- Combining Multiple Variables: AI can handle the integration and analysis of numerous variables, which would be challenging manually. This capability allows researchers to consider a wider range of factors in their studies.
Future Directions and Implications
- Technological Advancements: Future improvements in AI and LLM technology will further benefit sleep research. Enhanced natural language processing and more sophisticated machine learning algorithms will provide even more detailed and accurate data interpretations.
- Broader Implications: The potential for these technologies to impact public health and personalized medicine is significant. By providing better sleep management strategies, AI can contribute to improved health outcomes on a broader scale.

Conclusion
Large language models and AI offer valuable tools for enhancing sleep research. By improving data quality, increasing research efficiency, and providing deeper insights, AI enables researchers to conduct more comprehensive and effective sleep studies. As these technologies advance, their applications will continue to expand, opening up new opportunities for innovation in health research. Researchers are encouraged to explore the use of AI and LLMs in their studies to gain more profound insights and develop more effective interventions.
You Might Also Be Interested
🚀📊 Learn more about how Fibion SENS can help you in your projects measuring physical activity and sedentary behavior!
🔍 Elevate your project with Fibion SENS Motion 3 Devices Test Package for precise activity analysis. Order now for hands-on experience and comprehensive insights.
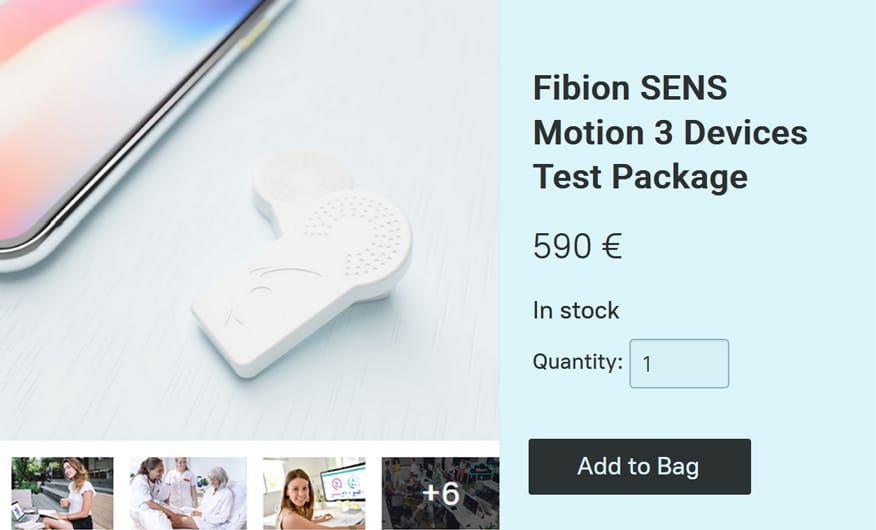
📅 If you want to learn more about Fibion SENS, do not hesitate to book a video call with our expert Dr. Miriam Cabrita.
🔍 You may also discover other valid and reliable products in our portfolio, such as the Fibion Device, Fibion Sleep, Fibion Mimove, Fibion Vitals, Fibion Emfit, and Fibion Circadian, all designed to assist in research measuring physical activity, sedentary behavior, and sleep.
Frequently Asked Questions
How do AI and LLMs improve sleep research? +
AI and LLMs enhance sleep research by integrating diverse data types like EEG readings, actigraphy, and sleep diaries. This comprehensive analysis provides deeper insights into sleep patterns and helps develop personalized interventions.
What types of data are used in sleep research? +
Sleep research uses various data types including EEG readings, actigraphy, sleep diaries, weather data, room temperature, and participant photos. These data help provide a comprehensive view of sleep patterns and influencing factors.
How does AI provide personalized sleep interventions? +
AI analyzes individual sleep patterns, environmental factors, and lifestyle habits to offer tailored recommendations. These may include optimal bedtimes, relaxation techniques, or changes in the sleep environment to improve overall sleep quality.
What are the benefits of using AI in sleep research? +
AI improves the accuracy of sleep data analysis, enhances research efficiency by automating data processing, and provides deeper insights by identifying subtle patterns. This leads to more effective and personalized sleep interventions.
What future advancements in AI and LLM technology can benefit sleep research? +
Future advancements in AI and LLM technology, such as enhanced natural language processing and more sophisticated machine learning algorithms, will provide even more detailed and accurate data interpretations, further benefiting sleep research.