1. Introduction
In this article, we explore how artificial intelligence (AI) is advancing physical activity research. AI technologies are utilized to analyze data from accelerometers, providing deeper insights and more accurate assessments. Here, we will highlight three notable examples of AI applications in physical activity studies.
AI’s role in physical activity research involves advanced algorithms that process data from wearable devices like accelerometers. These algorithms can:
- Classify Various Activities: Identify different types of physical activities.
- Detect Movement Patterns: Recognize subtle variations and patterns in movement.
- Provide Personalized Insights: Offer tailored health recommendations based on activity data.
These capabilities are crucial for understanding physical behavior and developing customized health interventions.
“AI algorithms offer detailed insights into physical activity patterns, crucial for personalized health interventions.”
2. Real-World Applications and Scientific Studies
2.1. Deep Learning for Activity Classification
Study: Nunavath et al. (2021)
- Outcome: Researchers used deep learning techniques to classify physical activities from accelerometer data, achieving high precision in recognizing various daily activities. Their approach effectively managed activities with overlapping feature spaces, enhancing the accuracy of physical activity monitoring.
- Impact: This approach is particularly beneficial for applications in rehabilitation and general monitoring systems, providing accurate detection of specific activities.
2.2. Unsupervised Machine Learning for Activity Quantification
Study: PLOS Digital Health (2021)
- Outcome: This research applied unsupervised machine learning methods, specifically a hidden semi-Markov model (HSMM), to segment and cluster accelerometer data from young children. The HSMM approach was found to be more suitable for analyzing physical activity in rapidly developing and heterogeneous populations compared to traditional methods.
- Impact: The HSMM approach provided a more accurate measure of physical activity, aligning closely with developmental assessments.
2.3. Multi-Scale Ensemble Method for Activity Recognition
Study: Zheng et al. (2013)
- Outcome: This study introduced a multi-scale ensemble method to improve the recognition of physical activities from triaxial accelerometer data. By dividing the data into short, non-overlapping windows and converting each into a feature vector, the researchers applied supervised learning algorithms to classify the activities. The multi-scale approach enhanced the accuracy of recognizing physical activities by leveraging patterns at different temporal scales.
- Impact: This method improved the accuracy of activity recognition, making it a valuable tool for detailed physical activity analysis.
“Machine learning models are transforming physical activity recognition, offering accurate and personalized insights into movement patterns.”
3. Conclusion
AI is significantly enhancing physical activity research by providing precise and continuous analysis of movement data. These advancements support the development of personalized health interventions and improve our understanding of physical behavior. As AI technology continues to evolve, its applications in physical activity studies will expand, offering more sophisticated tools to enhance health outcomes.
References
- Nunavath, V., Johansen, S., Johannessen, T.S., Jiao, L., Hansen, B.H., Berntsen, S., & Goodwin, M. (2021). Deep Learning for Classifying Physical Activities from Accelerometer Data. Sensors, 21(16), 5564. https://doi.org/10.3390/s21165564
- PLOS Digital Health. (2021). Using Unsupervised Machine Learning to Quantify Physical Activity from Accelerometry in a Diverse and Rapidly Changing Population. PLOS Digital Health. https://doi.org/10.1371/journal.pdig.0000220
- Zheng, Y., Won, W.-K., Guan, X., & Trost, S. (2013). Physical Activity Recognition from Accelerometer Data Using a Multi-Scale Ensemble Method. Proceedings of the AAAI Conference on Artificial Intelligence, 27(2), 1575-1581. https://doi.org/10.1609/aaai.v27i2.18997
Call to action
🚀📊 Learn more about how AI and wearable sensors like Fibion Vitals provide comprehensive insights into physical activity and sedentary behavior, enhancing health outcomes.
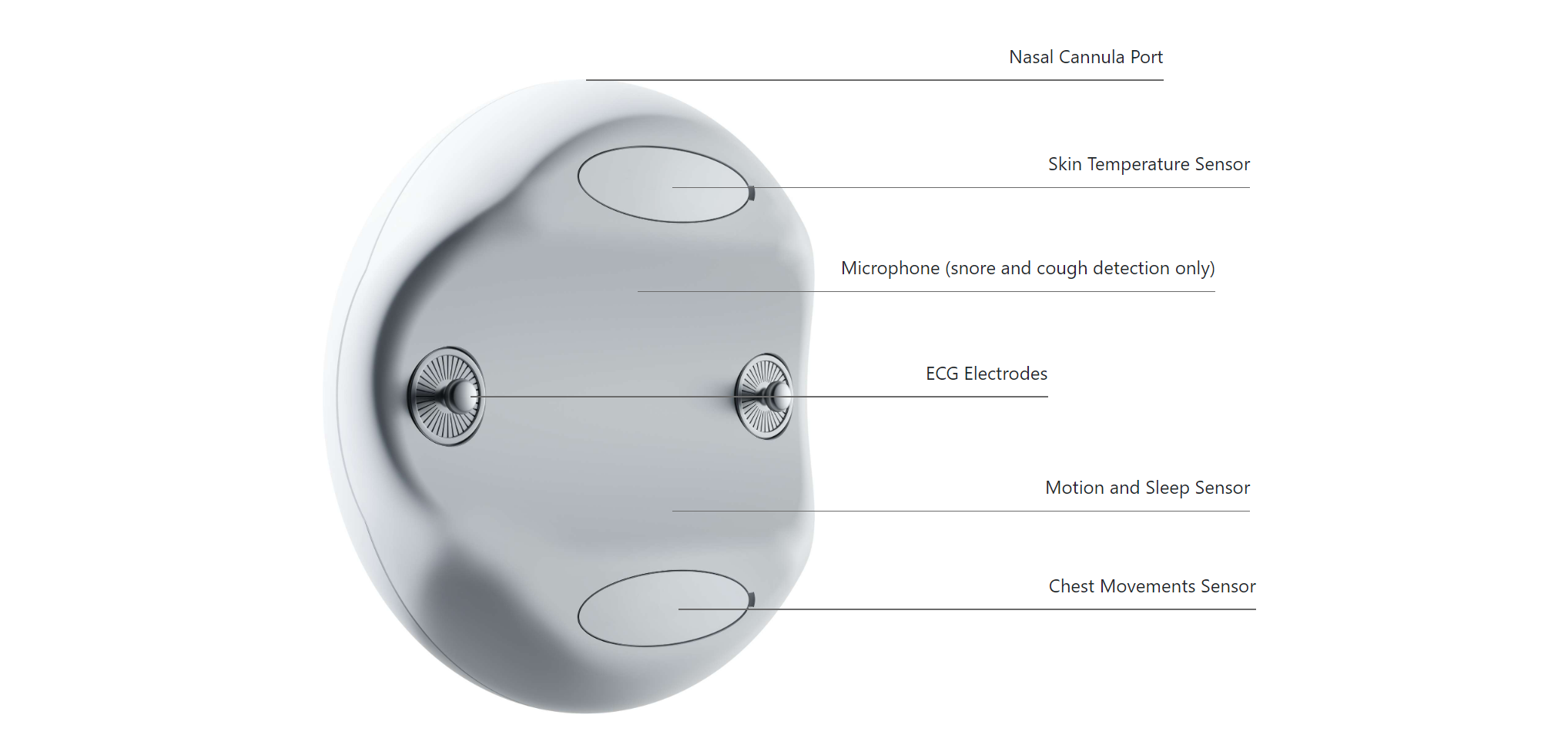
📅 If you want to learn more about Fibion Vitals, do not hesitate to book a video call with our expert Dr. Miriam Cabrita.
📚 Explore Our Article Collection on Sedentary Behavior and Physical Activity.
🔍 You may also discover other valid and reliable products in our portfolio, such as the Fibion Device, Fibion Sleep, Fibion Mimove, Fibion Sens, Fibion Emfit, and Fibion Circadian, all designed to assist in research measuring physical activity, sedentary behavior, and sleep.
Frequently asked questions:
How does AI contribute to physical activity research? +
AI contributes to physical activity research by using advanced algorithms to process data from wearable devices like accelerometers. These algorithms classify various activities, detect movement patterns, and provide personalized health insights based on activity data.
How does AI classify physical activities? +
AI classifies physical activities by analyzing data from accelerometers. Deep learning techniques and machine learning models recognize various daily activities with high precision, enhancing the accuracy of physical activity monitoring.
Can AI detect subtle movement patterns? +
Yes, AI can detect subtle movement patterns by processing data from wearable devices. Advanced algorithms analyze variations and patterns in movement, providing detailed insights into physical behavior.
What are some real-world applications of AI in physical activity research? +
AI has been used for deep learning in activity classification, unsupervised machine learning for activity quantification, and multi-scale ensemble methods for activity recognition. These applications provide personalized health insights and improve physical activity monitoring.
How does AI improve health interventions based on physical activity data? +
AI improves health interventions by providing precise and continuous analysis of physical activity data. These insights support the development of personalized health interventions, enhancing overall health outcomes.
What future advancements can be expected from AI in physical activity research? +
As AI technology continues to evolve, its applications in physical activity research will expand. Future advancements may include more sophisticated tools for analyzing movement patterns, leading to better health interventions and improved understanding of physical behavior.