1. Introduction
In this article, we explore how artificial intelligence (AI) is being used to analyze sedentary behavior. AI technologies offer new ways to understand and address sedentary lifestyles, which are linked to various health risks. Here, I’ll highlight two significant examples of AI applications in sedentary behavior research.
AI’s role in sedentary behavior research involves using advanced algorithms to process data from wearable sensors. These algorithms can:
- Identify Behavior Patterns: Recognize sedentary behavior over time.
- Detect Activity Changes: Notice subtle variations in physical activity levels.
- Provide Personalized Insights: Offer tailored health advice based on sedentary behavior data.
These capabilities are essential for developing interventions to reduce sedentary time and improve overall health.
“AI algorithms offer detailed insights into sedentary behavior, crucial for personalized health interventions.”
2. Real-World Applications and Scientific Studies
2.1. Recognizing Sedentary Behavior with Machine Learning
Study: Kańtoch (2018)
- Outcome: This study developed a method for identifying sedentary behavior using machine learning analysis of data from wearable sensors integrated into smart clothing. The system continuously monitored physical activities in a free-living environment, providing early detection of increased cardiovascular risk due to sedentary behavior.
- Impact: The high accuracy of the classification models (95% ± 2.11%) demonstrates the potential of AI in monitoring and managing sedentary behavior, crucial for preventing cardiovascular diseases.
2.2. Challenges and Opportunities in Analyzing Wrist Accelerometer Data
Study: Gao et al. (2021)
- Outcome: This review paper discusses the challenges and opportunities of analyzing physical activity and sedentary behavior data collected from wrist-worn accelerometers. It provides a comprehensive overview of various data processing methods, including machine learning approaches, to improve the accuracy and reliability of behavioral assessments.
- Impact: The paper highlights the importance of developing standardized methods for processing wrist-worn accelerometer data to accurately assess sedentary behavior and physical activity levels.
“Machine learning models are transforming sedentary behavior research, offering accurate and personalized insights into physical activity patterns.”
3. Conclusion
AI is significantly enhancing sedentary behavior research by providing precise and continuous analysis of activity data. These advancements support the development of personalized health interventions and improve our understanding of the impact of sedentary behavior on health. As AI technology continues to evolve, its applications in sedentary behavior studies will expand, offering more sophisticated tools to enhance health outcomes.
References
- Kańtoch, E. (2018). “Recognition of Sedentary Behavior by Machine Learning Analysis of Wearable Sensors during Activities of Daily Living for Telemedical Assessment of Cardiovascular Risk.” Sensors, 18(10), 3219. https://doi.org/10.3390/s18103219
- Gao, Z., Liu, W., McDonough, D.J., & Zeng, N. (2021). “The Dilemma of Analyzing Physical Activity and Sedentary Behavior with Wrist Accelerometer Data: Challenges and Opportunities.” Journal of Clinical Medicine, 10(24), 5951. https://doi.org/10.3390/jcm10245951
For more insights into how AI is transforming sedentary behavior research, explore our comprehensive article collection here.
You Might Also Be Interested
🚀📊 Learn more about how Fibion SENS can help you in your projects measuring physical actcivity and sedentary behaviour!
🔍 Elevate your project with Fibion SENS Motion 3 Devices Test Package for precise activity analysis. Order now for hands-on experience and comprehensive insights.
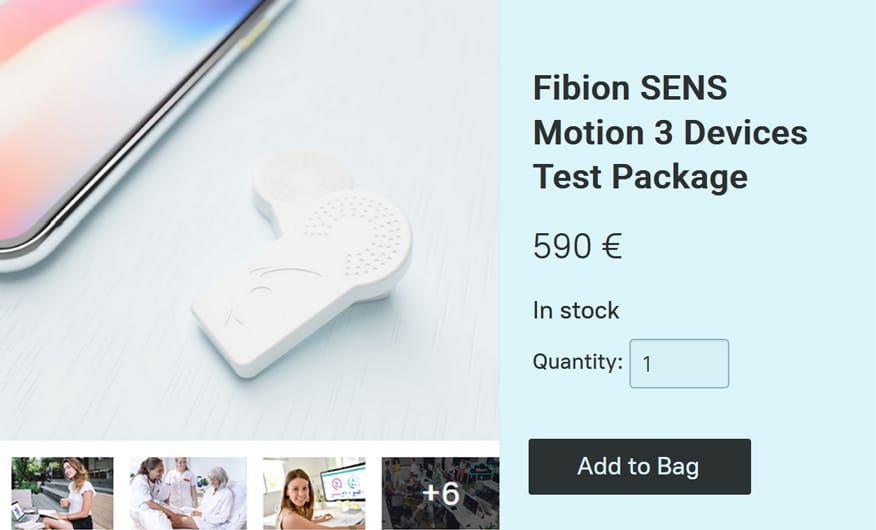
📅 If you are interested to learn more about Fibion SENS, do not hesitate to book a video call with our expert Dr. Miriam Cabrita.
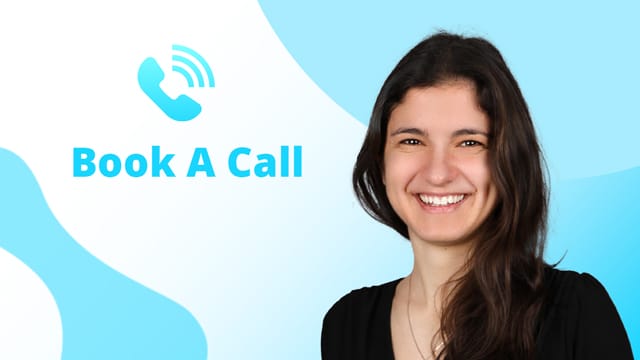
🔍 You may also discover other valid and reliable products in our portfolio, such as the Fibion Device, Fibion Sleep, Fibion Mimove, Fibion Vitals, Fibion Emfit, and Fibion Circadian, all designed to assist in research measuring physical activity, sedentary behavior, and sleep.
Frequently asked questions:
How does AI contribute to sedentary behavior research? +
AI contributes to sedentary behavior research by using advanced algorithms to process data from wearable sensors. It identifies behavior patterns, detects activity changes, and provides personalized health insights, which are crucial for developing interventions to reduce sedentary time and improve overall health.
How does AI identify sedentary behavior? +
AI identifies sedentary behavior by analyzing data from wearable sensors. Machine learning models recognize patterns of inactivity over time, providing detailed insights into sedentary behavior.
Can AI detect changes in physical activity levels? +
Yes, AI can detect changes in physical activity levels by analyzing data from wearable sensors. It notices subtle variations in activity, providing accurate assessments of physical behavior.
What are some real-world applications of AI in sedentary behavior research? +
AI has been used to recognize sedentary behavior with machine learning, as seen in studies like Kańtoch (2018), and to address challenges in analyzing wrist accelerometer data, as discussed in Gao et al. (2021). These applications provide personalized health insights and improve sedentary behavior assessments.
How does AI improve health interventions based on sedentary behavior data? +
AI improves health interventions by providing precise and continuous analysis of sedentary behavior data. These insights support the development of personalized health interventions, enhancing overall health outcomes.
What future advancements can be expected from AI in sedentary behavior research? +
As AI technology continues to evolve, its applications in sedentary behavior research will expand. Future advancements may include more sophisticated tools for analyzing sedentary patterns, leading to improved health interventions and better understanding of the impact of sedentary behavior on health.