Definition and Overview
Ecological Momentary Assessment (EMA) is a research method that captures human experiences and behaviors in real-time within their natural environments. It stands out for its dynamic approach to data collection, emphasizing temporal and contextual accuracy. This methodology is particularly effective in capturing the nuances and variability of human experiences, as it minimizes retrospective bias and enhances the ecological validity of the data. For further insights and practical tips on ESM data analysis, exploring resources like Analyzing ESM Data: A Guide can be immensely beneficial. Furthermore, for further insights into the foundational aspects of ESM, Introduction to Experience Sampling Method (ESM) provides a comprehensive overview. Additionally, uncover the distinct characteristics and applications of the Experience Sampling Method (ESM) and Ecological Momentary Assessment (EMA) in our detailed guide, Experience Sampling Method (ESM) vs. Ecological Momentary Assessment (EMA): Understanding the Differences and Applications. This resource provides an in-depth comparison of these two methodologies, helping researchers choose the most suitable approach for their studies.
“EMA offers a unique lens into the daily lives of individuals, unveiling patterns and interactions that are often missed in traditional research settings.”
EMA leverages technology, such as smartphones and wearable devices, to gather data through self-reports, physiological measurements, or a combination of both. This approach allows researchers to:
- Observe: Track real-world, real-time behaviors and experiences.
- Understand: Gain insights into the context and triggers of various phenomena.
- Analyze: Explore patterns, trends, and associations in everyday life.
Historical Development of EMA
The roots of EMA can be traced back to the diary studies of the early 20th century. However, it was not until the 1970s that EMA began to take shape as a formalized method, driven by advancements in technology and a growing interest in understanding behaviors in naturalistic settings.
- 1970s-1980s: Initial conceptualization and application in behavioral sciences.
- 1990s: Technological advancements lead to more sophisticated data collection.
- 2000s to Present: Integration with digital technology, expanding EMA’s capabilities.
“From paper diaries to smartphones, the evolution of EMA mirrors the technological advancements of each era, continuously enhancing its potential for in-depth human studies.”
The shift towards digital EMA has opened new horizons in research, enabling more complex study designs and real-time data analysis.
Basic Principles of EMA
At its core, EMA is governed by several key principles that define its methodology and application:
- Real-Time Data Collection: Capturing experiences and behaviors as they occur. For further insights and practical tips on ESM data analysis, exploring resources like Analyzing ESM Data: A Guide can be immensely beneficial.
- Ecological Validity: Ensuring that data reflects real-world environments.
- Temporal Precision: Focusing on the timing and sequence of events.
These principles contribute to the unique strengths of EMA, such as:
- Reduced Recall Bias: By recording events as they happen, EMA minimizes the inaccuracies often found in retrospective reports.
- Contextual Richness: It provides a deeper understanding of behaviors and experiences within their natural context.
- Dynamic Data: EMA captures the ebb and flow of human experiences, allowing for a more nuanced analysis.
EMA’s methodology is adaptable across various domains, making it a versatile tool for researchers interested in the intricate dynamics of human behavior and experience. The next sections of this chapter will delve deeper into the historical development, technological advancements, and basic principles that underpin Ecological Momentary Assessment.
Key Components and Methodology of EMA
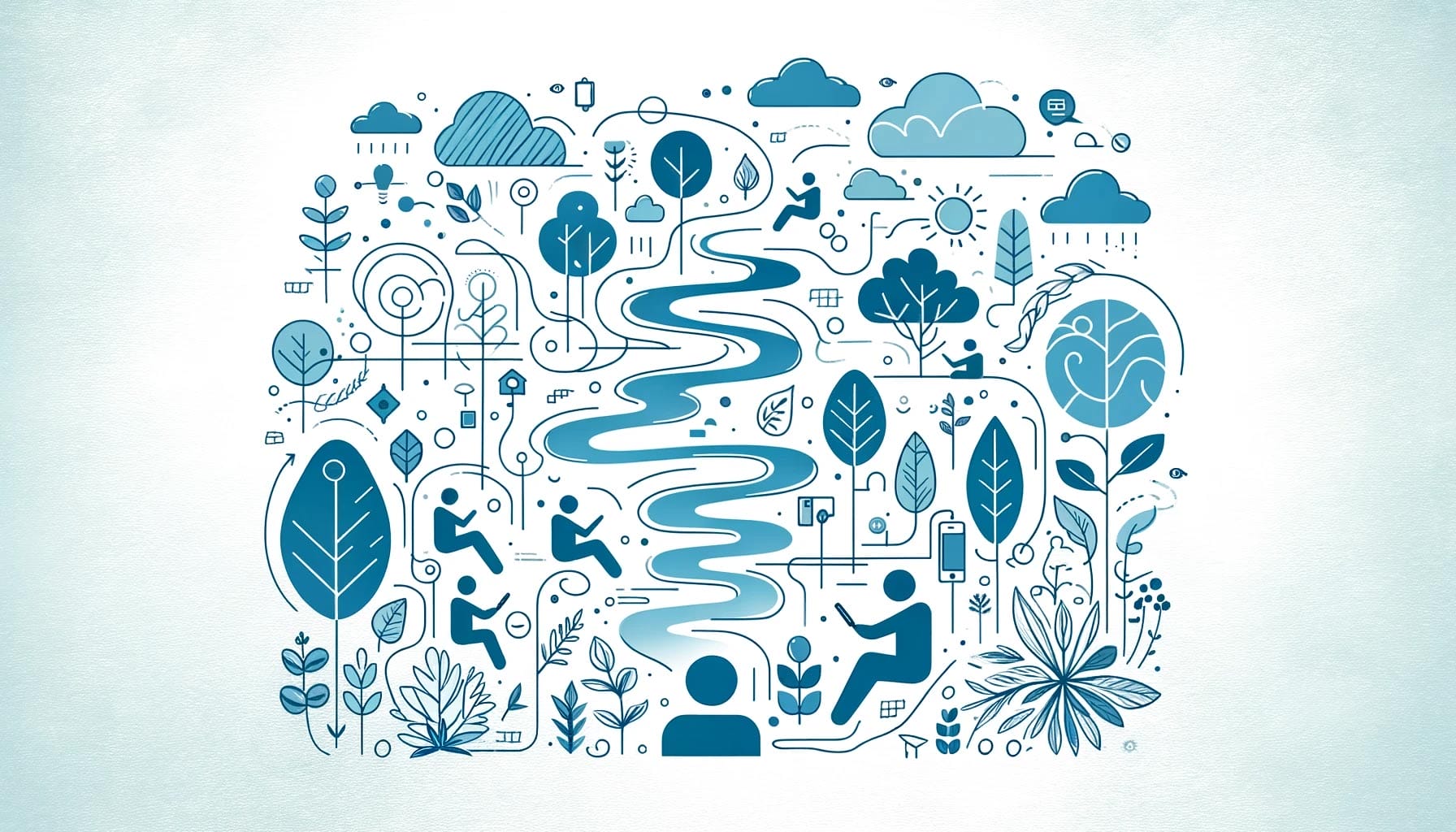
Designing EMA Studies
Designing an EMA study involves several critical considerations to ensure data quality and relevance. These include determining the sampling strategy, the frequency and timing of assessments, and the type of data to be collected. The design phase should balance scientific rigor with the practicalities of participants’ daily lives.
Key Considerations:
- Sampling Strategy: Random or event-based triggers for data collection.
- Assessment Frequency: Balancing the need for detailed data with participant burden.
- Data Types: Combining self-reports with physiological measures for a comprehensive view.
“A well-designed EMA study is a symphony of precision and practicality, harmonizing rigorous scientific inquiry with the real-world experiences of participants.”
Technological Tools in EMA
The advancement of technology has significantly enhanced the capabilities of EMA. Modern tools include smartphones, wearable devices, and custom applications, enabling more efficient and unobtrusive data collection.
Technologies Utilized:
- Smartphones: Ubiquitous and user-friendly, ideal for self-report data.
- Wearable Devices: Continuous physiological monitoring without intruding into daily life.
- Custom Apps: Tailored data collection interfaces, enhancing participant engagement and data quality.
Data Collection and Management
Data collection in EMA is a dynamic process that requires careful planning and execution. Ensuring data accuracy and integrity is paramount, as is safeguarding participant privacy.
Critical Aspects:
- Real-Time Data Capture: Accurate and timely recording of experiences and behaviors.
- Data Integrity: Robust systems to ensure the accuracy and reliability of collected data.
- Privacy Protections: Safeguarding participant data in line with ethical standards.
Analytical Techniques in EMA
EMA data analysis can be complex due to the volume and intricacy of the data. Advanced statistical methods are often employed to uncover patterns and relationships within the data.
Analytical Methods:
- Descriptive Analysis: Understanding basic trends and patterns.
- Multilevel Modeling: Accounting for the nested structure of EMA data.
- Time-Series Analysis: Exploring temporal dynamics and causal relationships.
“The analytical phase of EMA transforms raw data into meaningful insights, revealing the intricate tapestry of human experience.”
In summary, the key components and methodology of EMA revolve around thoughtful study design, leveraging modern technology, meticulous data collection and management, and sophisticated analytical techniques. These elements work in concert to uncover the rich, nuanced details of human behavior and experience sampling in natural settings. The following sections will further explore these components, offering insights into their practical application and impact on EMA research.
Applications and Benefits of EMA
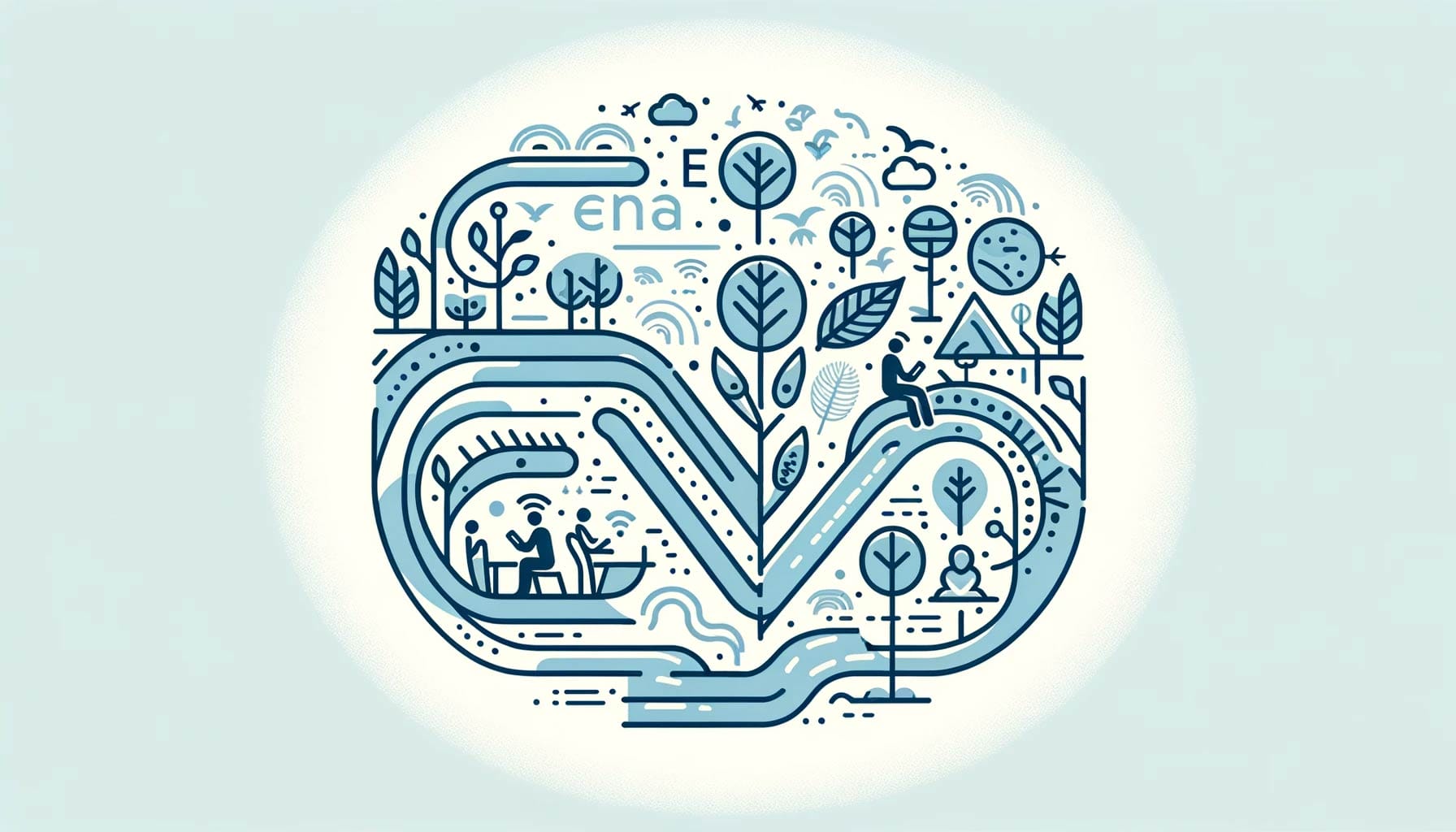
Broad Spectrum of Applications
Ecological Momentary Assessment has found diverse applications across various fields, significantly enhancing our understanding of human behavior and experiences in real-world settings.
“EMA’s versatility allows it to illuminate the intricacies of human behavior across multiple domains, from mental health to physical well-being.”
Key Areas of Application:
- Psychology: Understanding mood fluctuations and mental health patterns.
- Healthcare: Monitoring symptoms and treatment effects in patients.
- Behavioral Research: Studying real-time decision-making and lifestyle choices.
Advantages of EMA in Research
EMA offers several advantages over traditional research methods, primarily due to its real-time, context-rich data collection approach.
Core Benefits:
- Reduced Recall Bias: Capturing data in real-time mitigates memory distortion.
- Contextual Relevance: Gathering data in natural environments enhances ecological validity.
- Dynamic Data: Tracking changes over time provides insights into patterns and triggers.
Illustrative Examples:
- In mental health research, EMA identifies triggers of anxiety attacks in real-time, leading to more effective coping strategies.
- In lifestyle studies, EMA captures dietary choices throughout the day, offering a detailed understanding of eating habits.
“By bridging the gap between the laboratory and life, EMA offers a window into the complexities of human behavior in its natural habitat.”
Impact on Participant Behavior
EMA’s interactive nature can also influence participant behavior, an aspect researchers need to be mindful of.
Considerations:
- Reactivity: Awareness of being monitored can alter behavior.
- Self-reflection: Regular assessments may prompt changes in awareness and behavior.
Balancing Act:
- Designing EMA studies to minimize reactivity while capitalizing on self-reflective benefits is crucial for obtaining authentic data.
EMA’s Role in Personalized Interventions
EMA data can be instrumental in developing personalized interventions, particularly in healthcare and psychological treatment.
Applications in Personalization:
- Tailored Treatment Plans: Using EMA data to customize therapeutic approaches.
- Real-time Feedback: Providing immediate interventions based on EMA input.
“EMA not only captures the rhythm of daily life but also holds the potential to orchestrate better, more personalized interventions, tuning into each individual’s unique patterns.”
In conclusion, the applications and benefits of EMA are wide-ranging and significant. Its ability to capture real-time, ecologically valid data offers unparalleled insights into human behavior, making it an invaluable tool in research and personalized interventions. The following sections will delve into the challenges and limitations of EMA, providing a comprehensive understanding of its scope and efficacy.
Methodological Considerations in EMA
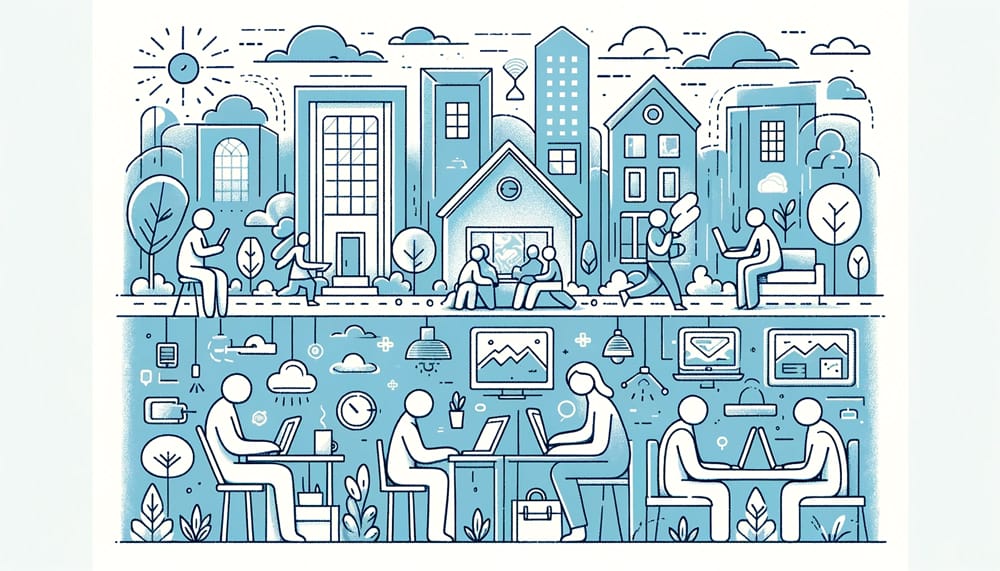
Designing EMA Studies
Effective EMA study design is critical for collecting high-quality data. This section explores key aspects of designing robust EMA research.
Components of Study Design:
- Sampling Strategies: Balancing frequency and timing of assessments.
- Questionnaire Design: Crafting concise, relevant questions.
- Technology Use: Selecting appropriate platforms and devices.
Consideration: A well-designed EMA study considers the balance between data richness and participant burden.
Data Collection and Management
EMA relies on efficient data collection and management practices to ensure data integrity and usability.
Key Practices:
- Secure Data Storage: Protecting participant data with robust security measures.
- Data Processing: Efficient handling and processing of large datasets.
- Quality Control: Implementing checks to ensure data accuracy.
“The backbone of effective EMA research lies in meticulous data collection and management, ensuring the integrity and reliability of the findings.”
Analyzing EMA Data
EMA data analysis requires specialized approaches due to the nature and volume of the data collected. For more detailed methodologies on adjusting frequency and timing in ESM, Analyzing ESM Data: A Step-by-Step Guide can be a valuable resource. Furthermore, explore essential strategies for dealing with missing data in ESM studies in our detailed guide, Handling Missing Data in Experience Sampling Method (ESM) Research: Best Practices, where we cover best practices and effective approaches.
Analytical Techniques:
- Time-Series Analysis: Understanding patterns over time.
- Contextual Analysis: Interpreting data within environmental or situational contexts.
- Mixed-Methods Approaches: Combining quantitative and qualitative data analysis.
Challenge: Navigating the complexity of EMA data requires both statistical expertise and an understanding of the contextual nuances.
Adapting to Technological Advances
EMA research must evolve with technological advancements to remain effective and relevant.
Technological Integration:
- Mobile Health Applications: Leveraging smartphone technology for data collection.
- Wearable Devices: Utilizing wearables for physiological data tracking.
- Artificial Intelligence: Applying AI for sophisticated data analysis.
“Staying abreast of technological trends is not just beneficial but essential for EMA researchers to enhance the scope and accuracy of their studies.”
In summary, methodological considerations in EMA encompass study design, data collection, analysis, and technological adaptation. Each element plays a crucial role in the success of an EMA study, ensuring the collection of meaningful, reliable data. The next sections will explore the diverse applications and benefits of EMA, highlighting its impact and utility in various research domains.
EMA in Psychological Research
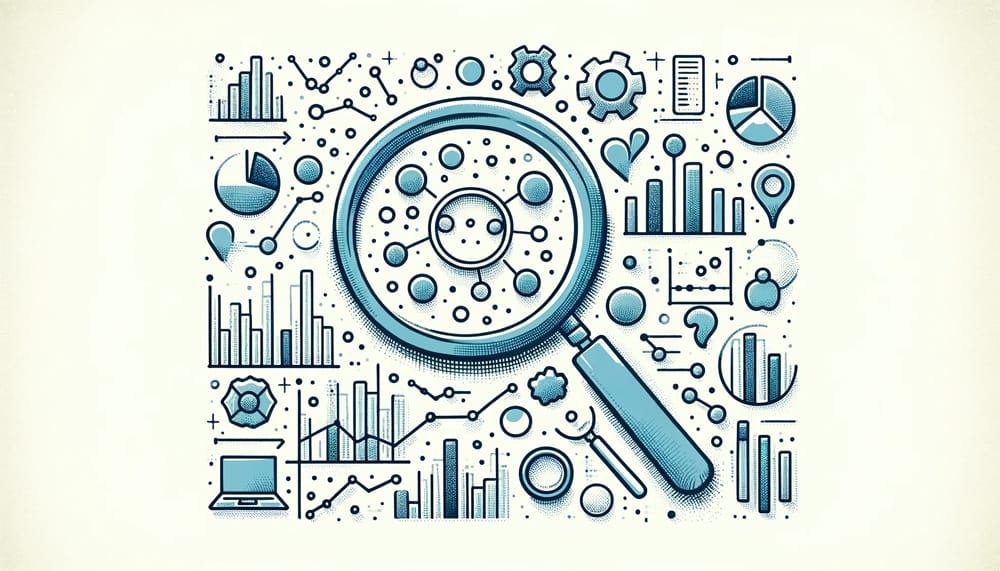
Application in Studying Mood and Behavior
Understanding mood and behavior is a pivotal aspect of psychological research. EMA offers an unparalleled opportunity to capture these dynamics in real-time. Explore the effective integration of the Experience Sampling Method (ESM) with other research methodologies in our comprehensive guide, Combining Experience Sampling Method (ESM) with Other Research Methods: A How-To Guide. This guide provides practical advice and strategies for researchers looking to enhance their studies by combining ESM with qualitative and quantitative approaches.
Key Insights:
- Real-Time Mood Tracking: EMA allows for the assessment of mood fluctuations throughout the day.
- Behavioral Context: Understanding how environmental factors influence behavior.
“EMA bridges the gap between laboratory settings and real-world behavior, providing insights into the dynamic nature of human mood and actions.”
EMA in Cognitive and Clinical Psychology
EMA is particularly valuable in cognitive and clinical psychology, offering a nuanced view of mental processes and disorders.
Areas of Impact:
- Cognitive Functioning: Assessing memory, attention, and problem-solving in daily life.
- Mental Health Monitoring: Tracking symptoms of mental health disorders.
Challenge: The complexity of mental processes requires careful consideration in EMA study design to ensure accurate and sensitive data collection.
Case Studies and Key Findings
Case studies in psychology have demonstrated the effectiveness of EMA in uncovering novel insights about human behavior and mental health.
Notable Studies:
- Mood Disorders: Examining the day-to-day variability in mood among individuals with bipolar disorder.
- Stress and Coping: Understanding how people (i.e., adults and older adults) cope with stress in their daily lives.
“Case studies in EMA reveal the intricate patterns of psychological functioning, highlighting its utility in both research and clinical settings.”
In summary, EMA’s application in psychological research offers a detailed and dynamic perspective of mood, behavior, and mental health. The method’s ability to capture real-time data in natural settings makes it a powerful tool for understanding the complexities of human psychology. The following sections will delve into the use of EMA in health and medical research, underscoring its growing significance across various domains.
EMA in Health and Medical Research
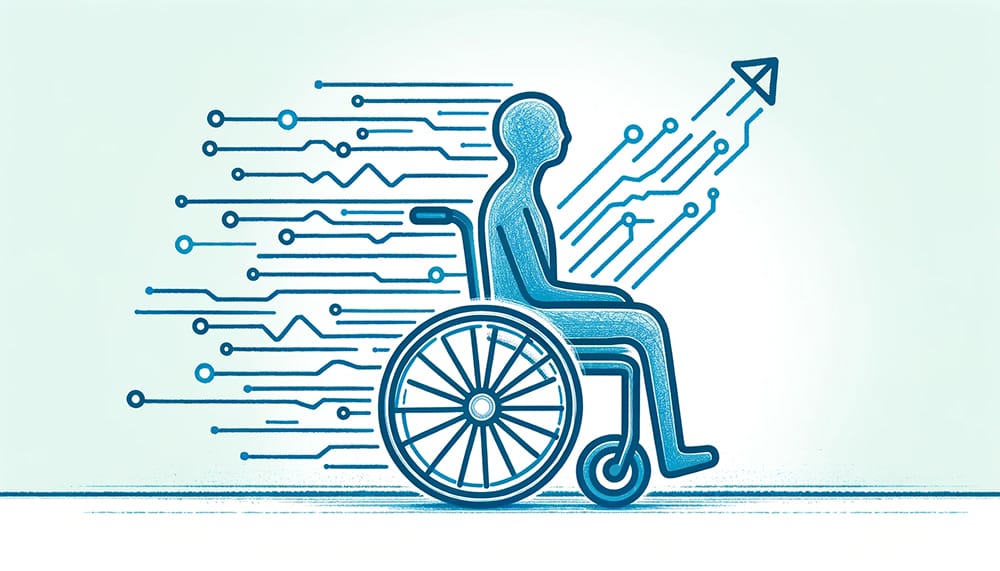
Monitoring Physical Health and Chronic Conditions
EMA has revolutionized the monitoring of physical health and chronic conditions, offering real-time insights and personalized data.
Key Contributions:
- Symptom Tracking: Patients can report symptoms as they occur, leading to more accurate diagnoses.
- Medication Adherence: EMA helps in tracking and improving medication adherence.
“Through EMA, healthcare professionals can understand the daily challenges faced by patients, enabling more effective management of chronic conditions.”
EMA in Mental Health Research
EMA’s role in mental health research is significant, providing detailed insights into the daily experiences of individuals with mental health disorders.
Advancements:
- Mood and Anxiety Disorders: Real-time monitoring of mood swings and anxiety episodes.
- Therapeutic Interventions: EMA data assists in tailoring therapies to individual needs.
Challenges: Balancing the need for detailed data with the burden of frequent reporting for patients with mental health issues.
Implications for Patient-Centered Care
EMA has profound implications for patient-centered care, promoting a more nuanced understanding of individual health experiences.
Impact Areas:
- Personalized Treatment Plans: Tailoring treatment to individual patient needs based on EMA data.
- Enhanced Patient Engagement: Involving patients more actively in their own care.
“EMA transforms patient care from a generalized approach to a deeply personal journey, fostering better health outcomes and patient satisfaction.”
In summary, EMA’s application in health and medical research underscores its capacity to provide in-depth, personalized health insights. Its use in both physical and mental health domains demonstrates its versatility and effectiveness in improving patient care. The following section will explore EMA’s role in social and behavioral sciences, further highlighting its broad applicability.
EMA in Social and Behavioral Sciences
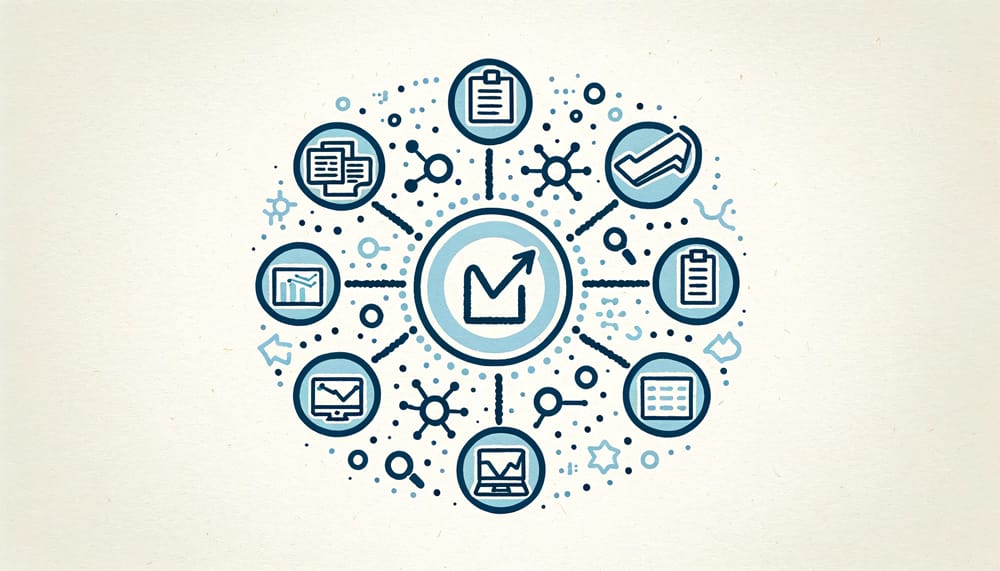
Studying Social Interactions and Relationships
EMA has opened new pathways for understanding social interactions and relationships by capturing real-time data in natural settings.
Innovations:
- Dynamic Social Processes: EMA captures the ebb and flow of social dynamics.
- Relationship Quality Assessment: It helps in assessing the quality of relationships over time.
Example: “EMA studies have shown how mood can influence social interactions, offering insights into relationship dynamics.”
Behavioral Patterns and Decision-Making
EMA is instrumental in uncovering the nuances of human behavior and decision-making processes.
Key Insights:
- Real-Time Decision Making: EMA data sheds light on how decisions are influenced by immediate contexts and emotional states.
- Habit Formation: It tracks how habits form and change over time.
Observation:
“Through EMA, researchers can see the interplay between context, mood, and choices, offering a deeper understanding of behavioral patterns.”
Contributions to Social Science Research
EMA’s contributions to social science research are significant, offering new perspectives and methodologies.
Areas of Impact:
- Cultural Differences: EMA helps in studying how cultural contexts influence behavior.
- Policy Making: Insights from EMA can inform social policies and interventions.
“EMA not only enriches our understanding of human behavior but also opens up new avenues for societal improvement through informed policy-making.”
In summary, EMA’s application in social and behavioral sciences significantly enhances our understanding of human interactions, behaviors, and decision-making processes. The following section will delve into the challenges and ethical considerations in EMA, highlighting the complexities and responsibilities inherent in this research method.
Challenges and Ethical Considerations in EMA design
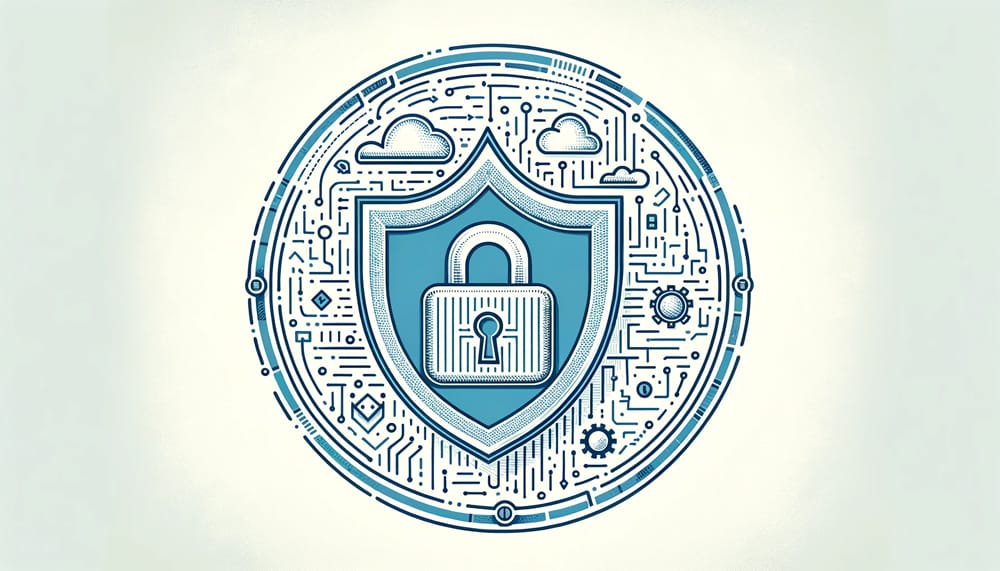
Addressing Participant Burden and Compliance
The implementation of Ecological Momentary Assessment (EMA) often encounters challenges related to participant burden and compliance.
Key Concerns:
- Intrusiveness: Frequent notifications and surveys can be intrusive, affecting participant compliance.
- Time Consumption: The time required for responding to EMA can be a deterrent for sustained participation.
Strategies for Mitigation:
- Balancing Frequency and Depth: Optimizing the frequency of prompts and depth of questions to reduce perceived burden. Gain insights into the nuances of setting the right frequency and timing for data collection in Experience Sampling Method (ESM) research by exploring our informative guide, Balancing Frequency and Timing in Experience Sampling Method (ESM): A Researcher’s Guide. This resource offers valuable advice for researchers on how to optimize these crucial aspects in ESM studies.
- Incentivizing Participation: Offering incentives can motivate continued participation.
Ethical Issues in Real-Time Data Collection
Ethical considerations are paramount in EMA, given its nature of collecting sensitive, real-time data. Ensuring the privacy and confidentiality of participant data, as highlighted in Ethical Considerations in ESM Research.
Areas of Ethical Concern:
- Informed Consent: Ensuring participants are fully aware of the nature of data collection.
- Sensitive Data Handling: The potential for collecting sensitive or distressing information inadvertently.
Ethical Framework:
- Robust Consent Processes: Clear, ongoing consent processes.
- Sensitive Data Protocols: Establishing protocols for handling sensitive data, including opting out options.
Ensuring Privacy and Data Security
The privacy and security of the data collected through EMA are critical concerns.
Challenges:
- Data Security: Protecting the data from unauthorized access and breaches.
- Privacy: Ensuring that participants’ identities and responses remain confidential.
Solutions:
- Advanced Encryption: Using advanced encryption methods for data storage and transmission.
- Privacy-by-Design: Incorporating privacy considerations at every stage of EMA tool development.
In summary, while EMA offers valuable insights, it also poses unique challenges and ethical considerations. Addressing participant burden, ensuring ethical data collection practices, and maintaining privacy and data security are essential for the responsible use of EMA. The next section will explore case studies and practical applications of EMA, providing real-world contexts to these methodologies.
Case Studies and Practical Applications of EMA protocol
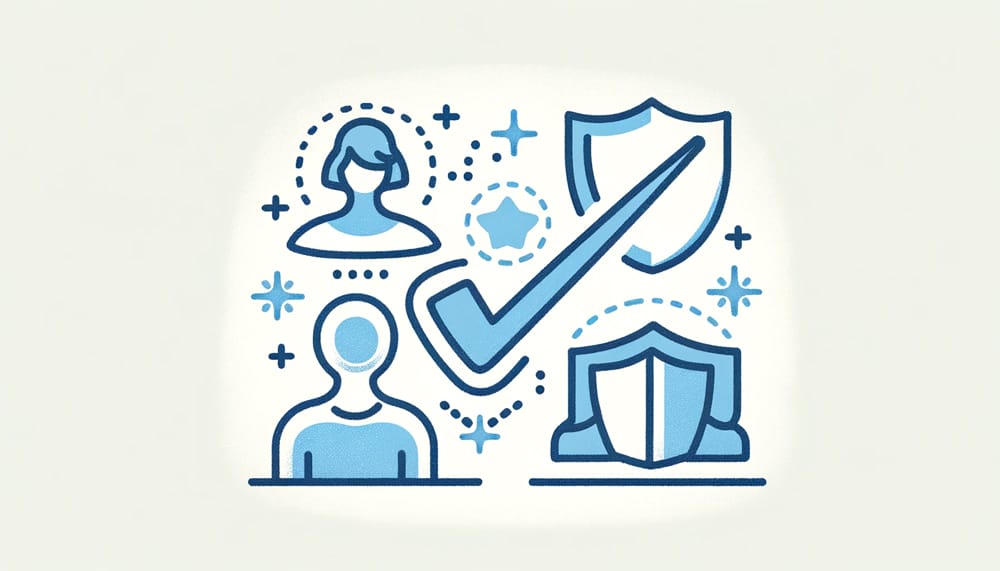
Case Study 1: EMA in Lifestyle and Wellness
Overview:
This case study focuses on how Ecological Momentary Assessment (EMA) is used to enhance lifestyle and wellness research. Research Objective:
To understand the daily health behaviors and emotional well-being of individuals. Methodology:
Participants received prompts several times a day through a mobile app, inquiring about their physical activity, dietary choices, mood, and stress levels. Enhance your understanding of creating impactful prompts in Experience Sampling Method (ESM) studies with our guide, Crafting Effective Experience Sampling Method (ESM) Prompts: Tips and Examples. This resource offers practical tips and illustrative examples to assist researchers in designing prompts that effectively elicit valuable responses in ESM research.
Key Findings:
- Real-Time Insights: EMA provided real-time data on lifestyle choices, revealing patterns in diet and exercise.
- Emotional Well-Being: Fluctuations in mood and stress were closely related to lifestyle habits.
- Personalized Feedback: EMA enabled the provision of personalized health recommendations.
Implications:
The study demonstrates the potential of EMA in providing insights into daily health behaviors, offering a basis for personalized wellness programs.
Case Study 2: EMA in Environmental Research
Overview:
This case study illustrates the application of EMA in studying human interactions with the environment. Research Goal:
To observe the real-time impact of environmental factors on individual behavior and well-being. Approach:
Participants responded to EMA prompts about their location, activities, and subjective experiences in different environmental settings. Significant Outcomes:
- Environmental Influences: Clear correlations were found between environmental quality and mood.
- Behavioral Responses: Participants’ behavior, like seeking green spaces, varied according to environmental conditions.
- Data Richness: EMA provided detailed, situational data that traditional surveys could not capture.
Conclusions:
The case study underscores EMA’s effectiveness in environmental research, offering nuanced insights into the dynamic relationship between humans and their surroundings.
In summary, these case studies highlight the diverse applications of EMA, from lifestyle and wellness to environmental research, showcasing its ability to capture complex, real-time data for deeper insights. The next section will delve into the future directions and emerging trends in EMA, exploring how this methodology continues to evolve and adapt to new research challenges.
Future Directions and Emerging Trends in Ecological Momentary Assessment (EMA)
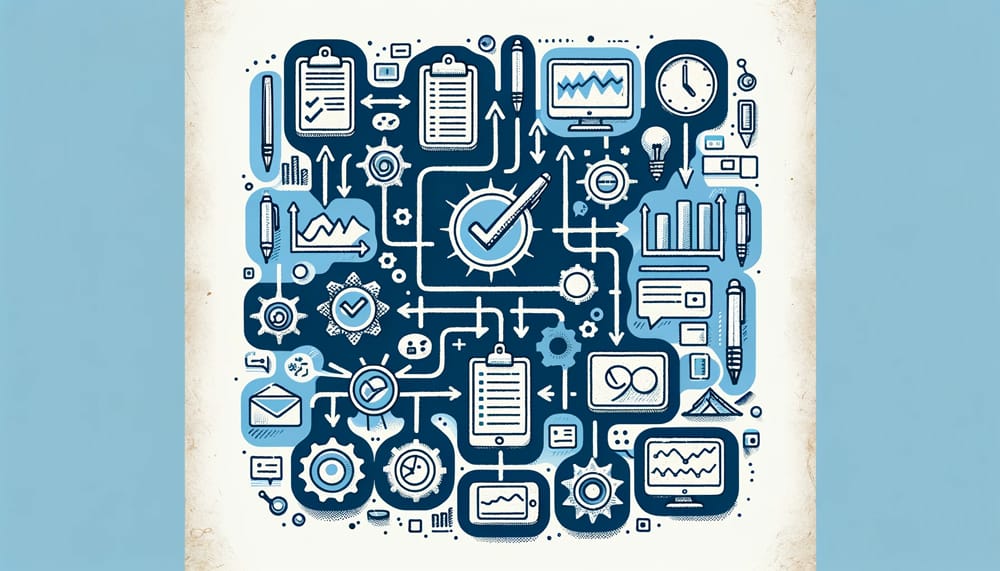
Innovations in EMA Methodology
Development of Advanced Analytical Tools:
The field of EMA is witnessing a surge in the development of sophisticated analytical tools. These tools are designed to handle the complexity and volume of data generated, enabling more nuanced and comprehensive analysis. For more in-depth guidance, exploring resources such as ESM Data Visualization Techniques can be incredibly beneficial.
Integration of Artificial Intelligence:
Artificial Intelligence (AI) and Machine Learning (ML) are increasingly being integrated into EMA. This integration allows for predictive modeling and pattern recognition, enhancing the ability to understand and anticipate behavioral and psychological trends.
Enhanced Participant Engagement Strategies:
Innovations in participant engagement, such as gamification and interactive interfaces, are being developed to improve compliance and reduce the perceived burden of participation in EMA studies. Learn the art of selecting the right participants for Experience Sampling Method (ESM) studies in our informative guide, How to Select Participants for an Experience Sampling Method (ESM) Study: Sampling Techniques. This guide delves into various sampling techniques, providing insights and strategies to help researchers ensure effective participant engagement in ESM research.
Predictions for Future Applications
Broader Scope in Healthcare:
EMA is expected to expand significantly in healthcare, particularly in chronic disease management and mental health, offering real-time monitoring and intervention capabilities.
Application in Personalized Medicine:
With its capacity for capturing individualized data, EMA is poised to play a crucial role in the burgeoning field of personalized medicine, contributing to tailored treatment strategies.
Environmental and Urban Planning:
EMA is predicted to be increasingly used in environmental research and urban planning, helping to understand the impact of environmental factors on human behavior and well-being.
Integrating EMA with Other Research Methods
Combination with Longitudinal Studies:
EMA is being integrated with traditional longitudinal research methods, providing a more dynamic and comprehensive view of behavioral and psychological changes over time. Discover solutions for ESM challenges in longitudinal research in this insightful article. In addition, explore the effective integration of the Experience Sampling Method (ESM) with other research methodologies in our comprehensive guide, Combining Experience Sampling Method (ESM) with Other Research Methods: A How-To Guide. This guide provides practical advice and strategies for researchers looking to enhance their studies by combining ESM with qualitative and quantitative approaches.
Synergy with Qualitative Research:
There is a growing trend to combine EMA with qualitative research methods, such as in-depth interviews, to enrich the data and provide deeper context to the findings.
Cross-Disciplinary Collaborations:
EMA is increasingly being used in cross-disciplinary studies, combining insights from psychology, sociology, environmental science, and technology, leading to more holistic understanding and innovative solutions.
In conclusion, the future of EMA is marked by technological innovation, expanding applications, and interdisciplinary collaborations. These developments are set to significantly enhance the capabilities and impact of EMA in various fields, providing deeper insights into human behavior and well-being. The next section will wrap up this comprehensive exploration of EMA, summarizing its impact and looking ahead to its future potential.
You Might Also Be Interested:
- 📚 Delve into our comprehensive ESM Article Collection for insightful perspectives on Experience Sampling Method (ESM).
- 🌟 See our article Best Tools for Experience Sampling Method in 2024 to discover the top tools in the field.
- 🔍 Learn how the advanced features of Fibion Insight can aid your ESM research.
- 📅 Planning an ESM study? For a chat with our ESM expert, book a session with Dr. Miriam Cabrita.
Frequently asked questions in this topic
What is Ecological Momentary Assessment (EMA)?+
EMA is a research method that captures human experiences and behaviors in real-time within their natural environments, using technology like smartphones and wearable devices for data collection.
How does EMA differ from traditional research methods?+
EMA differs by capturing immediate and contextual data, reducing retrospective bias and providing a more accurate and ecological validity of human experiences.
What are the key principles of EMA?+
The key principles include real-time data collection, ecological validity, temporal precision, reduced recall bias, contextual richness, and dynamic data capture.
What technological advancements have impacted EMA?+
Advancements in mobile and wearable technology have enhanced EMA by enabling efficient, real-time data collection and the integration of sensors for passive data collection.
What are some common challenges in conducting EMA research?+
Challenges include addressing participant burden and compliance, ensuring data accuracy and integrity, and maintaining privacy and data security.
What are the future directions and emerging trends in EMA?+
Future trends include the integration of AI and machine learning for advanced data analysis, broadening applications in healthcare and social sciences, and leveraging technology for more personalized and adaptive EMA studies.