1. Introduction
Welcome back to our mini-series on machine learning in health research! In this final article, we will explore the future trends and advancements in machine learning for physical activity research. We’ll discuss emerging technologies and methodologies that promise to further enhance our understanding and monitoring of physical activity.
2. New Developments in Machine Learning
2.1. Deep Learning
- Description: Deep learning, a specialized branch of machine learning, employs neural networks with multiple layers to model complex patterns in data. This approach excels in handling large datasets, uncovering intricate patterns that traditional machine-learning models might overlook.
- Application: Deep learning enhances the accuracy of activity recognition by processing extensive accelerometer data to detect subtle nuances in physical activities.
- Example: A study by Uddin and Soylu (2021) demonstrated the use of long short-term memory-based neural structured learning for human activity recognition using wearable sensors and discriminant analysis, achieving significant improvements in accuracy compared to traditional methods.
- Study: Uddin, M. Z., & Soylu, A. (2021). “Human activity recognition using wearable sensors, discriminant analysis, and long short-term memory-based neural structured learning.” Scientific Reports, 11(1), 16455.
2.2. Transfer Learning
- Description: Transfer learning involves taking a pre-trained model on one task and adapting it to a related task. This approach leverages existing knowledge, reducing the need for large amounts of labeled data.
- Application: In physical activity research, transfer learning can be used to apply models trained on one population (e.g., young adults) to another (e.g., older adults), improving generalizability.
- Example: Researchers at Stanford University used transfer learning to adapt models trained on accelerometer data from one study to another, enhancing the model’s applicability across different demographics.
- Study: Weiss, G. M., & Lockhart, J. W. (2012). “The Impact of Personalization on Smartphone-Based Activity Recognition.” AAAI Workshop on Activity Context Representation.
2.3. Real-Time Data Processing and Feedback
- Description: Advancements in real-time data processing enable the immediate analysis of accelerometer data, providing instant feedback to users.
- Application: This can be used in wearable fitness devices to offer real-time coaching and health monitoring, improving user engagement and outcomes.
- Example: Companies like Fitbit and Apple are already integrating real-time data processing in their wearables, providing users with instant feedback on their activity levels.
- Study: Apple Inc. (2021). “Apple Watch Series 6: Fitness and Health.”
“Emerging trends like deep learning, transfer learning, and real-time data processing are set to transform physical activity research and monitoring.”
3. Advanced Techniques
3.1. Multi-Modal Data Integration
- Description: Combining data from multiple sensors (e.g., accelerometers, heart rate monitors, GPS) provides a more comprehensive picture of physical activity and health.
- Application: This approach can enhance the accuracy of activity recognition and health predictions by leveraging diverse data sources.
- Example: A study by Wei and Wang (2024) utilized an attention mechanism time convolutional network to integrate data from multiple sensors, significantly improving the accuracy of human activity recognition.
- Study: Wei, X., & Wang, Z. (2024). “TCN-attention-HAR: human activity recognition based on attention mechanism time convolutional network.” Sci Rep, 14, 7414.
3.2. Personalized Health Recommendations
- Description: Machine learning algorithms can analyze individual activity data to provide personalized health recommendations.
- Application: Personalized feedback can help users make informed decisions about their physical activity, improving adherence to health recommendations.
- Example: Research by Apple Machine Learning Research (2020) demonstrated the use of personalized machine learning models to provide tailored heart rate estimates, which can inform fitness recommendations.
- Study: Apple Machine Learning Research. (2020). “Personalized Heart Rate Estimation.”
“Advanced techniques like multi-modal data integration and personalized health recommendations are enhancing the impact of machine learning in physical activity research.”
4. Future Directions
4.1. Explainable AI
- Description: As machine learning models become more complex, there is a growing need for explainability to understand how these models make decisions.
- Application: Explainable AI can help researchers and clinicians trust and adopt machine learning solutions by providing transparent and interpretable results.
- Example: Research on explainable AI is focusing on developing methods that make machine learning models more understandable without sacrificing performance.
- Study: Samek, W., et al. (2017). “Explainable Artificial Intelligence: Understanding, Visualizing and Interpreting Deep Learning Models.” ITU Journal: ICT Discoveries.
4.2. Ethical AI and Data Privacy
- Description: Ensuring the ethical use of AI and protecting data privacy are critical as more personal data is collected and analyzed.
- Application: Developing ethical guidelines and robust data protection frameworks will be essential for the widespread adoption of machine learning in health research.
- Example: The GDPR in Europe sets a precedent for data protection and privacy, influencing how health data is managed and used.
- Study: Voigt, P., & von dem Bussche, A. (2017). “The EU General Data Protection Regulation (GDPR): A Practical Guide.” Springer International Publishing.
“Future directions in explainable AI and ethical data practices are crucial for the sustainable development of machine learning applications in health research.”
5. Conclusion
The future of machine learning in physical activity research looks promising, with advancements in deep learning, transfer learning, real-time data processing, and multi-modal data integration. These technologies and methodologies will enhance our ability to monitor, understand, and improve physical activity and health outcomes.
References
- Uddin, M. Z., & Soylu, A. (2021). “Human activity recognition using wearable sensors, discriminant analysis, and long short-term memory-based neural structured learning.” Scientific Reports, 11(1), 16455. PMID: 34385552. Read more
- Weiss, G. M., & Lockhart, J. W. (2012). “The Impact of Personalization on Smartphone-Based Activity Recognition.” AAAI Workshop on Activity Context Representation. Read more
- Apple Inc. (2021). “Apple Watch Series 6: Fitness and Health.” Read more
- Wei, X., & Wang, Z. (2024). “TCN-attention-HAR: human activity recognition based on attention mechanism time convolutional network.” Sci Rep, 14, 7414. doi: 10.1038/s41598-024-57912-3. PMCID: PMC10978978. PMID: 38548859. Read more
- Apple Machine Learning Research. (2020). “Personalized Heart Rate Estimation.” Read more
- Samek, W., et al. (2017). “Explainable Artificial Intelligence: Understanding, Visualizing and Interpreting Deep Learning Models.” ITU Journal: ICT Discoveries. Read more
- Voigt, P., & von dem Bussche, A. (2017). “The EU General Data Protection Regulation (GDPR): A Practical Guide.” Springer International Publishing. Read more
You Might Also Be Interested
🚀📊 Learn more about how Fibion SENS can help you in your projects measuring physical activity and sedentary behavior!
🔍 Elevate your project with Fibion SENS Motion 3 Devices Test Package for precise activity analysis. Order now for hands-on experience and comprehensive insights.
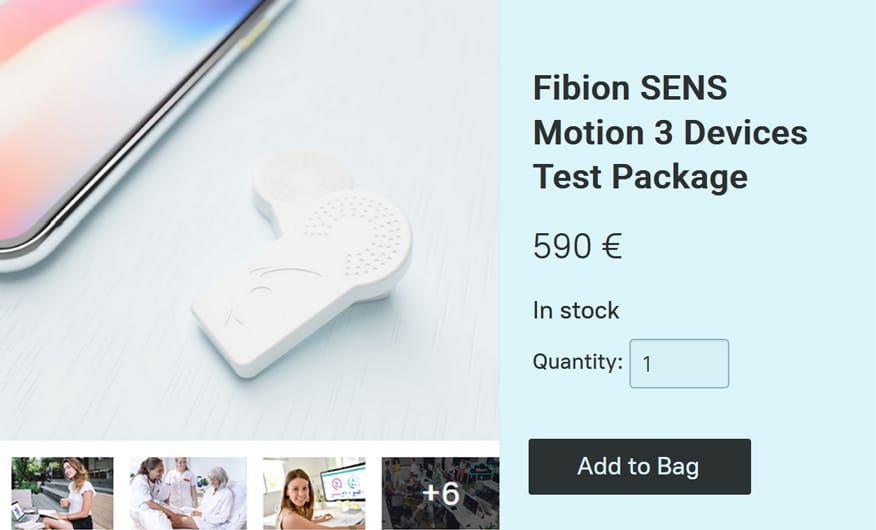
📅 If you are interested to learn more about Fibion SENS, do not hesitate to book a video call with our expert Dr. Miriam Cabrita.
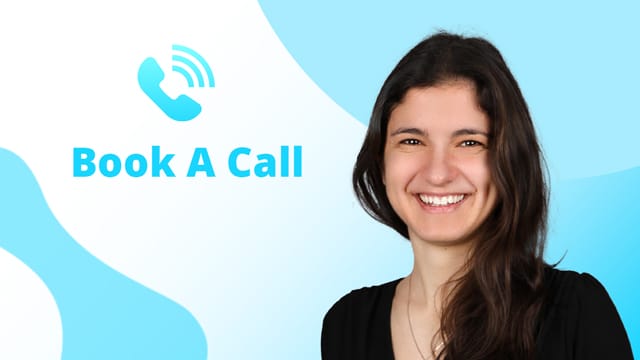
🔍 You may also discover other valid and reliable products in our portfolio, such as the Fibion Device, Fibion Sleep, Fibion Mimove, Fibion Vitals, Fibion Emfit, and Fibion Circadian, all designed to assist in research measuring physical activity, sedentary behavior, and sleep.
Frequently asked questions:
What are the emerging trends in machine learning for physical activity research? +
Emerging trends include deep learning, transfer learning, and real-time data processing. These techniques enhance activity recognition accuracy, generalize models across different populations, and provide instant feedback to users.
How does deep learning improve physical activity research? +
Deep learning utilizes neural networks with multiple layers to model complex patterns in data. It is effective in processing large datasets and uncovering intricate patterns in physical activities, improving the accuracy of activity recognition.
What is transfer learning and how is it applied in physical activity research? +
Transfer learning involves taking a pre-trained model on one task and adapting it to a related task. In physical activity research, it allows models trained on one population (e.g., young adults) to be applied to another (e.g., older adults), enhancing generalizability.
What are the benefits of real-time data processing in physical activity monitoring? +
Real-time data processing allows immediate analysis of accelerometer data, providing instant feedback to users. This can improve user engagement and outcomes by offering real-time coaching and health monitoring through wearable devices.
How does multi-modal data integration enhance physical activity research? +
Multi-modal data integration combines data from multiple sensors (e.g., accelerometers, heart rate monitors, GPS) to provide a comprehensive view of physical activity and health. This enhances the accuracy of activity recognition and health predictions by leveraging diverse data sources.
What is explainable AI and why is it important in health research? +
Explainable AI aims to make machine learning models more understandable and interpretable. In health research, it helps researchers and clinicians trust and adopt machine learning solutions by providing transparent and interpretable results, which are crucial for decision-making.