Introduction
Combining textual and visual data in research presents unique challenges. How can researchers effectively integrate these diverse data types to gain deeper insights?
Large language models (LLMs) offer a revolutionary solution for this complex task. In this article, we explore how LLMs can enhance data interpretation by seamlessly integrating textual and visual data, particularly in sedentary behavior and physical activity research.

Understanding Large Language Models (LLMs)
Large language models are advanced AI systems designed to process and understand human language. Their capabilities extend beyond text to include visual and audio data interpretation, making them powerful tools for comprehensive data analysis.
Key capabilities of LLMs include:
- Textual Analysis: Processing and understanding large volumes of text data, including handwritten logs and printed materials.
- Visual Analysis: Interpreting images and other visual inputs, such as photographs taken by participants.
- Audio Transcription: Transcribing and analyzing voice-based diary inputs.
- Contextual Integration: Combining textual, visual, and audio data to provide richer insights.
The Challenge of Combining Textual and Visual Data
In sedentary behavior and physical activity research, data is often collected from a variety of sources. This can include self-reported activity logs, images from participants, and audio diaries.
Integrating these diverse data types traditionally requires extensive manual effort, making it time-consuming and prone to errors. Researchers often struggle to merge these data types effectively, leading to incomplete or biased interpretations.
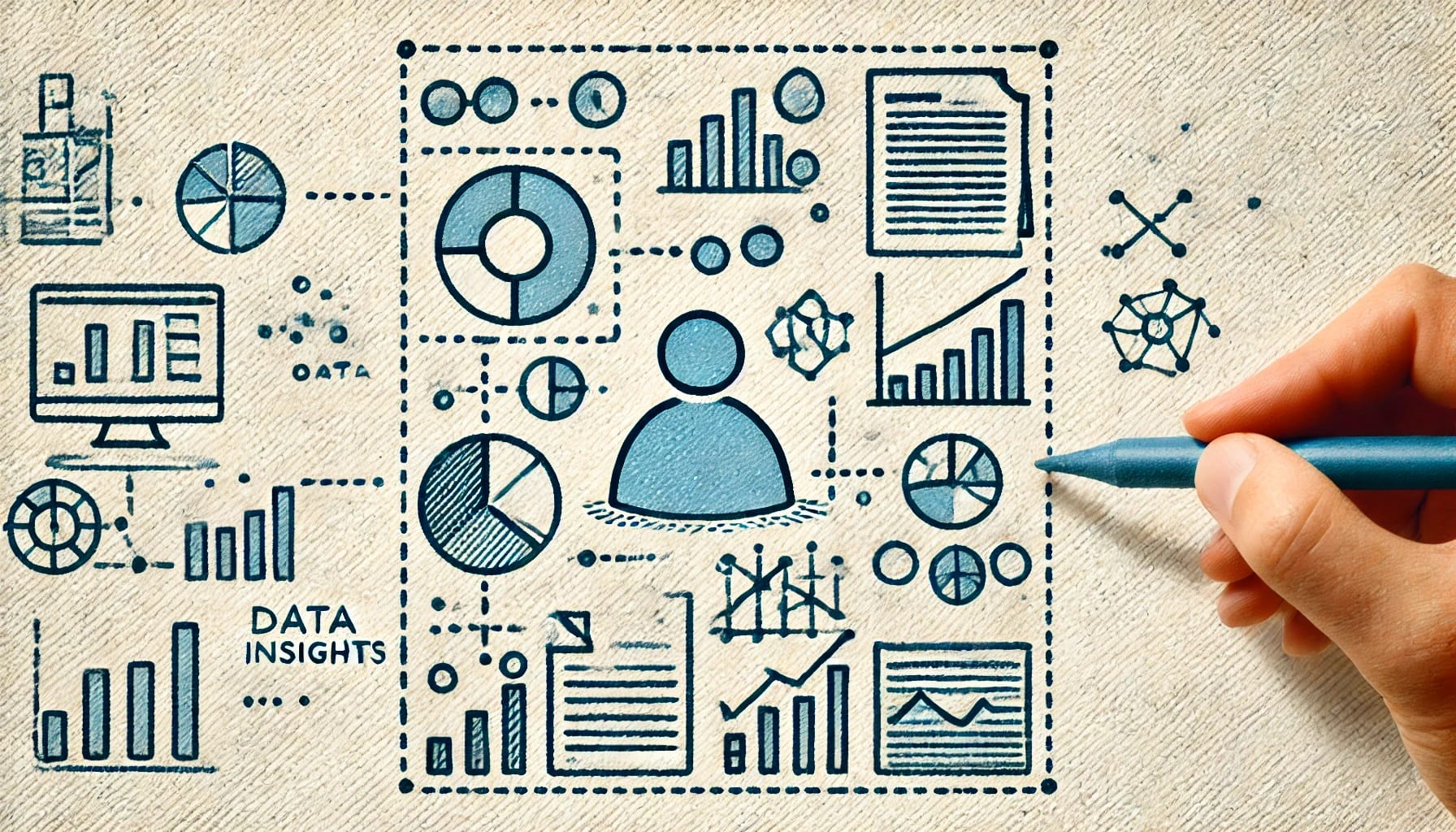
How LLMs Facilitate Advanced Data Interpretation
LLMs excel at processing both textual and visual data, enabling researchers to integrate these diverse data types seamlessly. This capability significantly reduces the manual work required, opening up new possibilities for research and different study designs.
Multimodal Data Processing:
- Textual Analysis: LLMs can transcribe and process handwritten logs and printed materials, reducing the need for manual data entry. This automation saves time and ensures accurate data transcription.
- Visual Analysis: Participants can be prompted to take photos of their physical activities. LLMs analyze these images to determine if the activity is indoors or outdoors, identify the environment, and assess if the participant is alone or with someone. This capability allows for detailed context analysis without the need for manual coding.
- Audio Transcription: LLMs can transcribe voice-based diary inputs, summarizing and analyzing them for patterns and insights. This enables the inclusion of rich, qualitative data without the extensive time investment traditionally required for transcription and analysis.
By combining these data types, LLMs provide a comprehensive view of the research subject. For instance, integrating handwritten activity logs, photos taken by participants, and voice recordings of daily experiences allows researchers to gain a detailed understanding of an individual’s sedentary behavior and physical activity patterns.
Benefits of LLMs in Research:
- Efficiency: Automatic transcription and image analysis streamline the data processing workflow, saving researchers significant time.
- Accuracy: LLMs improve data accuracy by minimizing manual entry errors and ensuring consistent analysis.
- New Research Designs: The ability to easily integrate diverse data types opens up new possibilities for research designs, allowing for more complex and comprehensive studies.
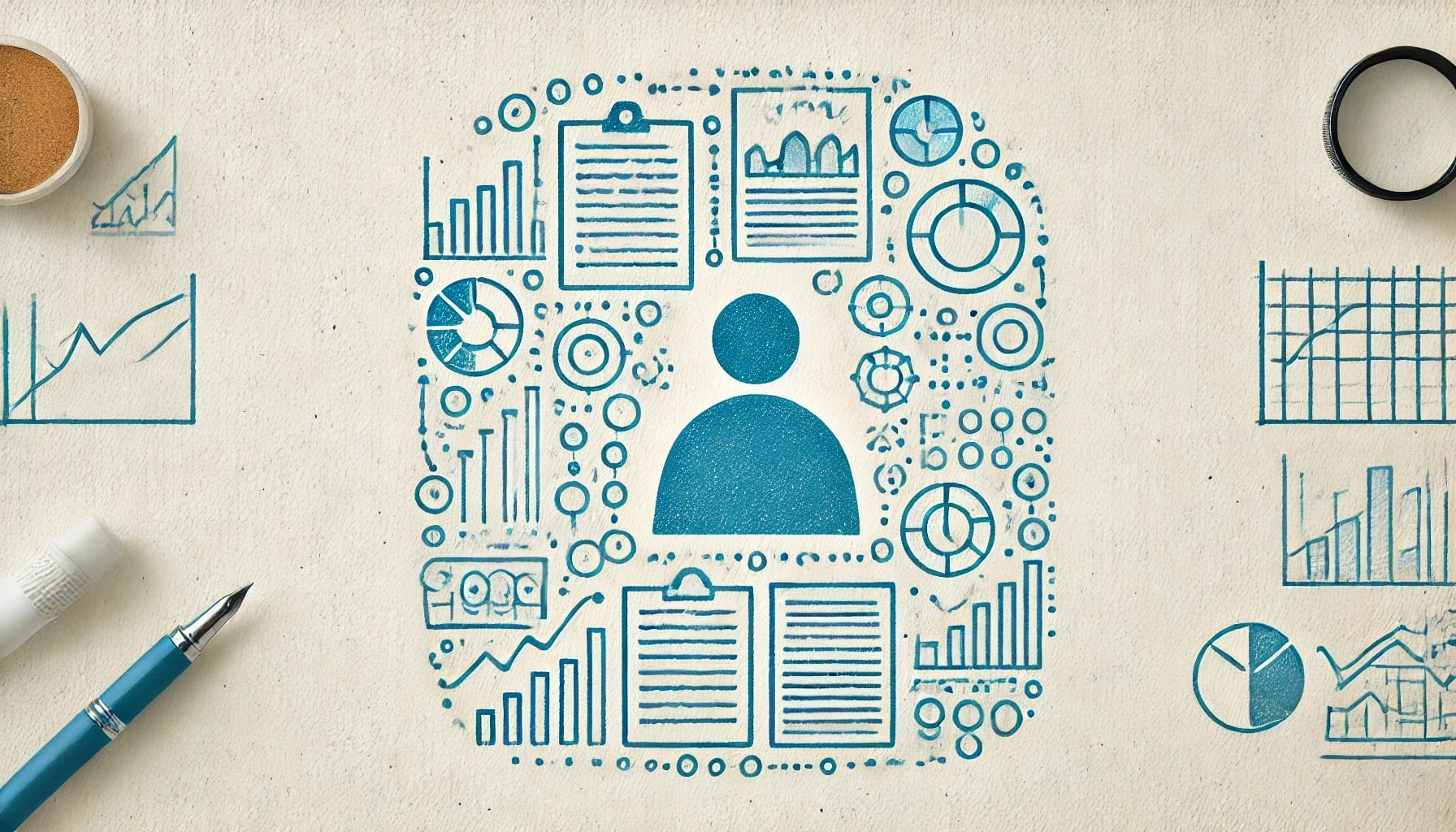
Practical Applications in Sedentary Behavior and Physical Activity Research
LLMs enable researchers to tackle the complex task of integrating various data types, leading to more insightful and actionable findings.
- Identifying Patterns: In sedentary behavior research, LLMs can analyze text logs, photos, and audio recordings to identify patterns of inactivity. For instance, if participants log their activities and submit photos, LLMs can determine when and where sedentary behavior occurs most frequently. They can analyze whether these periods are spent alone or with others, indoors or outdoors, and identify any associated environmental factors.
- Physical Activity Analysis: For physical activity studies, LLMs can integrate data from different sources to create a comprehensive activity profile. Participants can log their exercises, upload photos, and record voice diaries. LLMs can then analyze this data to identify the types and intensities of activities performed, as well as the contexts in which they occur. This holistic view helps researchers understand not just the quantity of physical activity but also the quality and context, which are crucial for designing effective interventions.
- Real-Time Monitoring and Feedback: LLMs also facilitate real-time monitoring and feedback. Participants can receive immediate, personalized advice based on their combined textual and visual data inputs. For example, if a participant logs feeling tired and uploads a photo showing a sedentary posture, the system can provide suggestions for light physical activities to boost energy levels.
Benefits of Using LLMs for Data Integration
Using LLMs for integrating textual and visual data in research offers several significant advantages:
- Enhanced Insights: Combining different data types provides a richer and more nuanced understanding of sedentary behavior and physical activity. Researchers can uncover deeper insights that are often missed when analyzing data in isolation.
- Efficiency and Accuracy: Automating the transcription and analysis of textual and visual data significantly reduces the time and effort required. This efficiency not only speeds up the research process but also improves data accuracy by minimizing manual entry errors.
- Personalized Research: The detailed and comprehensive analysis facilitated by LLMs allows for the creation of more personalized and effective health interventions. By understanding the specific contexts and patterns of behavior, researchers can tailor interventions to meet individual needs more precisely.
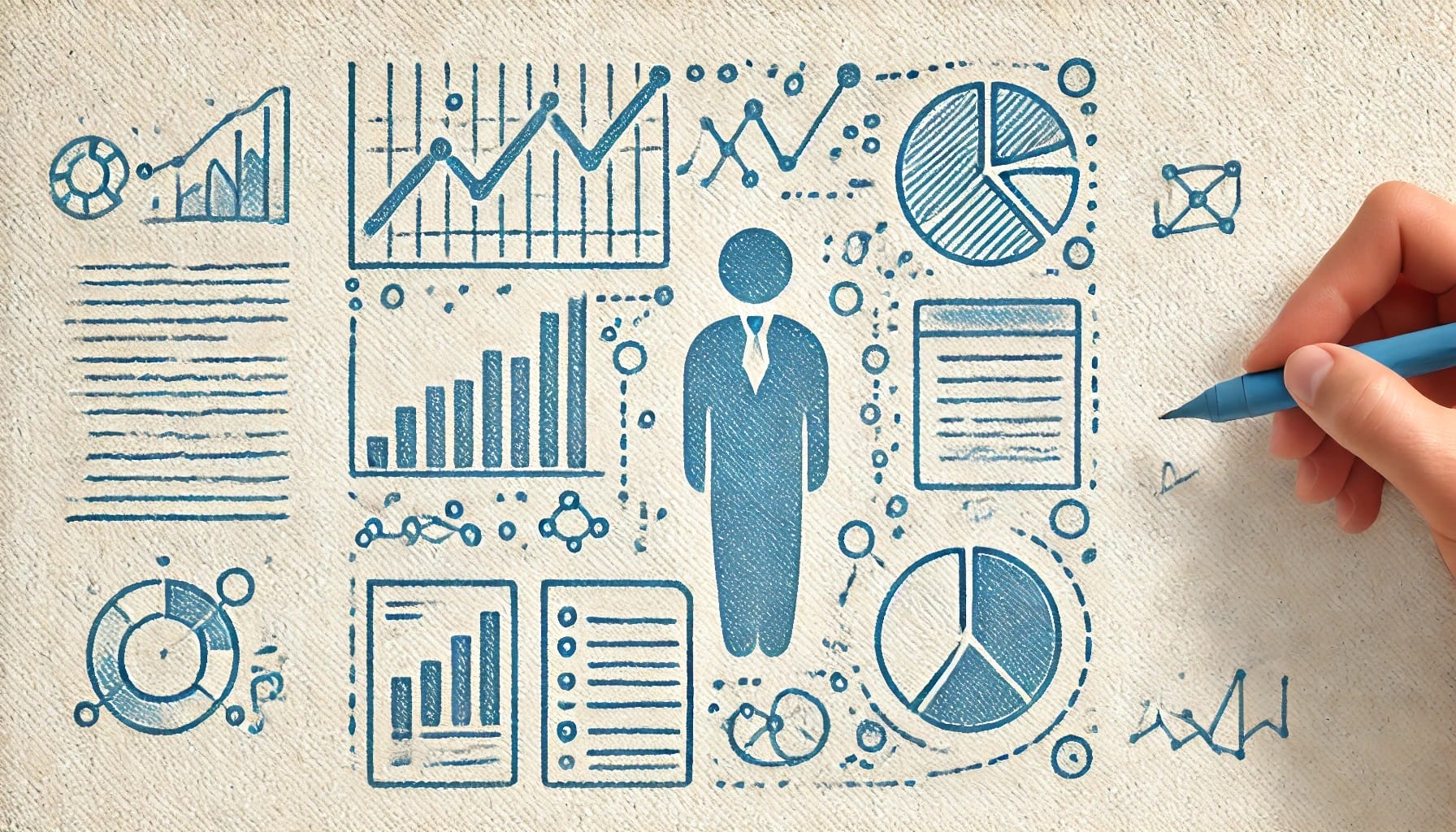
Future Directions and Implications
The future of LLMs in sedentary behavior and physical activity research is promising. As these models continue to evolve, their capabilities will expand, allowing for even more sophisticated data integration and analysis.
- Advancements in LLM Technology: Emerging features, such as enhanced natural language processing and more advanced predictive analytics, will further enhance the application of LLMs in health research. These advancements will enable even more detailed and accurate data interpretations.
- Broader Implications: The broader implications for public health and personalized medicine are significant. By providing a more comprehensive understanding of sedentary behavior and physical activity, LLMs can inform more effective public health strategies and personalized treatment plans. This technology has the potential to revolutionize the way we study and address these critical health behaviors.

Conclusion
Large language models have the potential to revolutionize sedentary behavior and physical activity research. By enhancing data quality, improving research efficiency, and offering deeper insights, LLMs provide researchers with powerful tools for comprehensive data analysis.
As these technologies advance, their applications will expand, leading to new opportunities for innovation in health research. Researchers are encouraged to explore the use of LLMs in their studies, harnessing these tools to gain more profound insights and drive effective interventions.
You Might Also Be Interested
🚀📊 Learn more about how Fibion SENS can help you in your projects measuring physical activity and sedentary behavior!
🔍 Elevate your project with Fibion SENS Motion 3 Devices Test Package for precise activity analysis. Order now for hands-on experience and comprehensive insights.
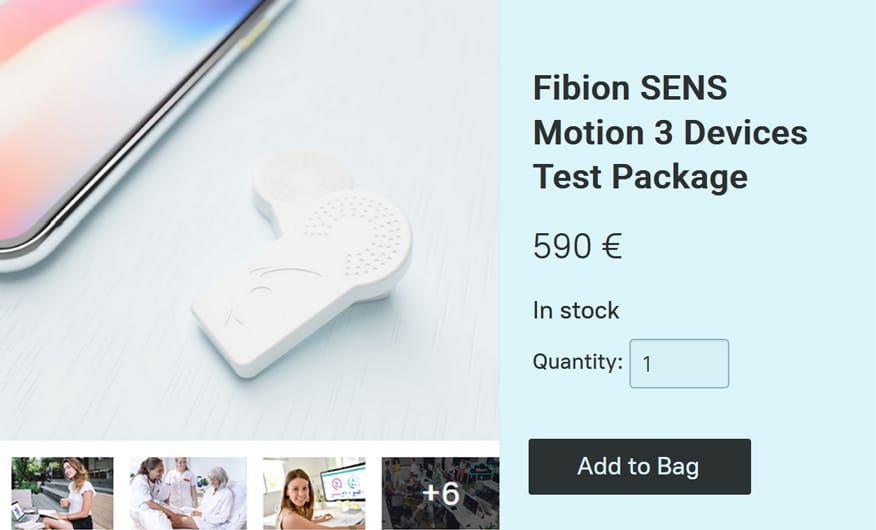
📅 If you want to learn more about Fibion SENS, do not hesitate to book a video call with our expert Dr. Miriam Cabrita.
🔍 You may also discover other valid and reliable products in our portfolio, such as the Fibion Device, Fibion Sleep, Fibion Mimove, Fibion Vitals, Fibion Emfit, and Fibion Circadian, all designed to assist in research measuring physical activity, sedentary behavior, and sleep.
Frequently Asked Questions
How do LLMs integrate textual and visual data in research? +
LLMs (large language models) process and combine diverse data types, including text, images, and audio. They can transcribe handwritten logs, analyze participant photos, and interpret voice diaries to provide a comprehensive view of research subjects.
What are the benefits of using LLMs in sedentary behavior and physical activity research? +
Using LLMs enhances data quality, improves research efficiency, and offers deeper insights. They automate data processing tasks, reduce manual errors, and enable the integration of various data types for richer, more accurate analyses.
How can LLMs improve real-time monitoring and feedback in research studies? +
LLMs facilitate real-time monitoring by analyzing incoming data and providing immediate, personalized feedback. For example, they can suggest activities based on real-time analysis of text logs, photos, and voice recordings submitted by participants.
What future advancements are expected in the use of LLMs for health research? +
Future advancements in LLM technology will include enhanced natural language processing and more sophisticated predictive analytics. These developments will further improve the integration and analysis of diverse data types, offering even more detailed and accurate health research insights.
How do LLMs handle qualitative data in research? +
LLMs handle qualitative data by processing and analyzing text from handwritten logs, printed materials, and voice-based diary inputs. They can identify patterns and insights within this qualitative data, making it easier for researchers to interpret and utilize in their studies.