Introduction to Large Language Models in Research
Analyzing large data sets in physical activity research has always been a complex task. The sheer volume and variety of data collected—from accelerometer readings to self-reported activity logs—pose significant challenges. How can researchers make sense of such extensive information efficiently? This is where large language models (LLMs) come into play. LLMs, a type of artificial intelligence, offer a powerful solution for processing and interpreting big data, uncovering patterns that traditional methods might miss. In this article, we explore how LLMs can revolutionize data analysis in physical activity research.
What Are Large Language Models?
Large language models are advanced AI systems designed to understand and generate human language. They are trained on vast amounts of text data, enabling them to recognize patterns, comprehend context, and generate meaningful insights. Key capabilities of LLMs include:
- Efficient Data Processing: LLMs can handle large volumes of data quickly and accurately.
- Pattern Recognition: These models excel at identifying trends and correlations within complex data sets.
- Contextual Understanding: LLMs can interpret the context of data, providing deeper insights beyond surface-level analysis.
By leveraging these capabilities, researchers can gain a more comprehensive understanding of their data, leading to more informed decisions and better research outcomes.
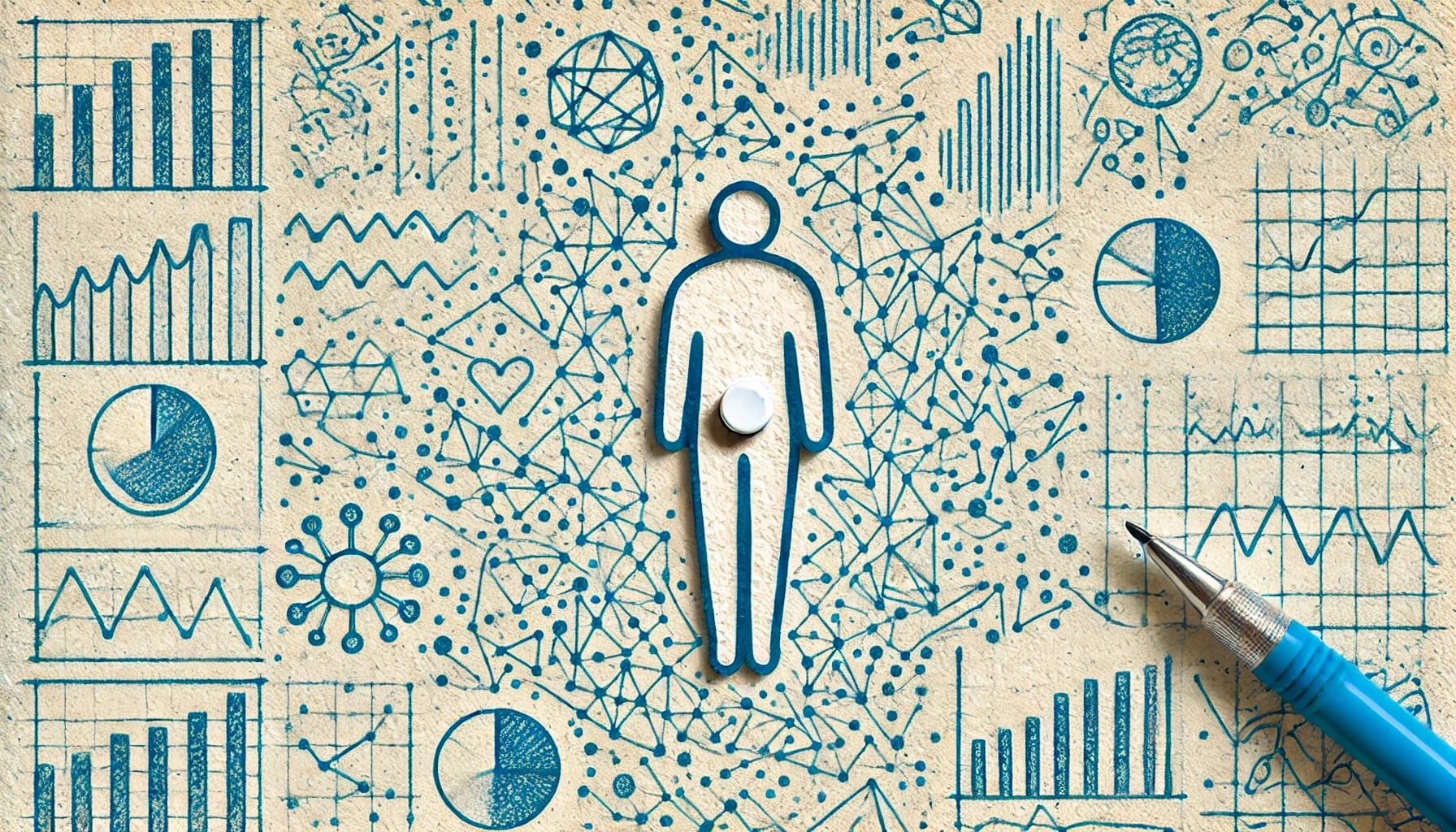
The Big Data Challenge in Physical Activity Studies
Physical activity research involves collecting diverse data types, such as movement patterns, heart rate, and sleep quality. These data sets are often massive and multifaceted, making traditional analysis methods time-consuming and prone to errors. Key challenges include:
- Data Complexity: The intricate nature of physical activity data, which combines quantitative and qualitative information.
- Volume: Large data sets from wearable devices and self-reports that require significant computational power to analyze.
- Traditional Analysis Limitations: Conventional methods may struggle to identify subtle patterns or correlations in the data.
These challenges highlight the need for more advanced tools, like LLMs, to effectively process and analyze physical activity data.
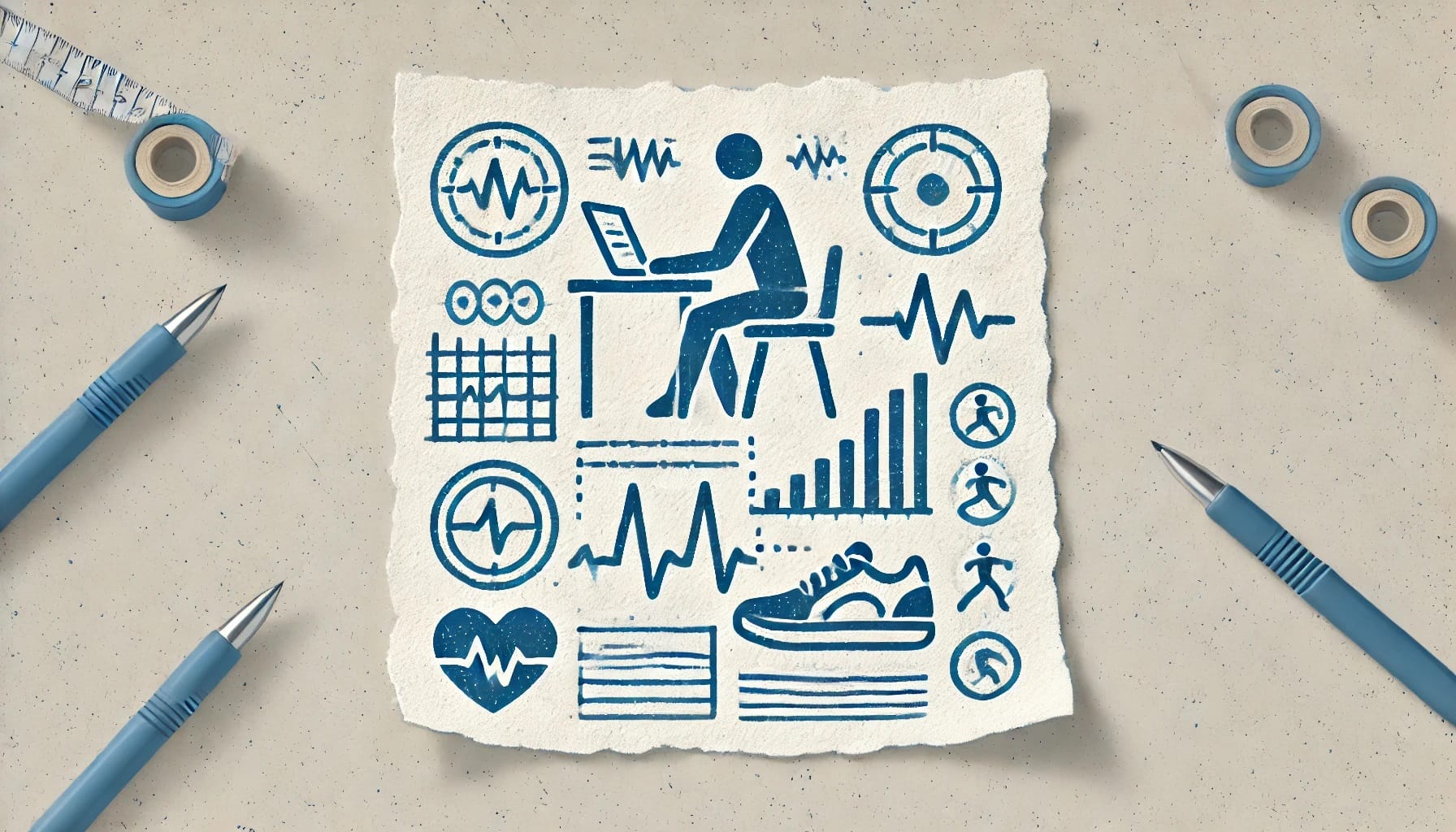
Transforming Data Analysis with LLMs
LLMs offer a transformative approach to data analysis in physical activity research. Here’s how:
- Efficient Processing: LLMs can quickly process and analyze large volumes of data, reducing the time required for data interpretation. This efficiency allows researchers to focus on generating insights and developing interventions.
- Pattern Recognition: By identifying hidden patterns and correlations, LLMs can reveal insights that might be missed by traditional methods. This capability is crucial for understanding complex behaviors and health outcomes.
- Enhanced Insights: LLMs provide a deeper understanding of the data by integrating various sources and types of information, leading to more accurate and comprehensive findings.
Benefits and Future Prospects
Using LLMs in physical activity research offers several significant benefits:
- Enhanced Insights: LLMs enable researchers to uncover deeper insights from their data, leading to more effective interventions and better health outcomes.
- Time and Accuracy: The efficiency and accuracy of LLMs reduce the time required for data analysis, allowing for faster and more reliable results.
- Looking Ahead: As LLM technology continues to evolve, its applications in physical activity research will expand. Future developments may include more sophisticated models capable of even greater data integration and analysis capabilities.
Conclusion
Large language models have the potential to revolutionize big data analysis in physical activity research. By efficiently processing large volumes of data and uncovering hidden patterns, LLMs provide researchers with deeper insights and more accurate results. As this technology continues to advance, its impact on health research will only grow, offering new opportunities for innovation and improved health outcomes. Researchers are encouraged to explore the use of LLMs in their studies to harness these powerful tools for their research endeavors.
You Might Also Be Interested
📅 If you want to learn more about Fibion SENS, do not hesitate to book a video call with our expert Dr. Miriam Cabrita.
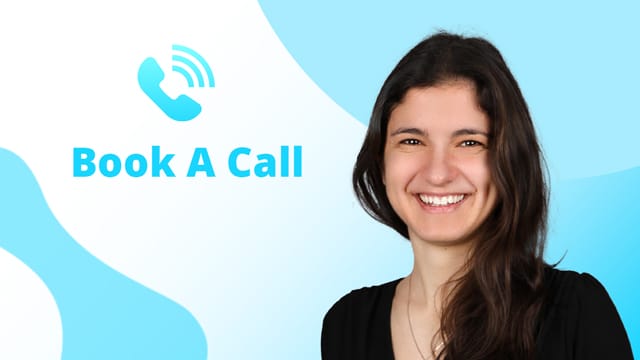
🔍 Elevate your project with Fibion SENS Motion 3 Devices Test Package for precise activity analysis. Order now for hands-on experience and comprehensive insights.
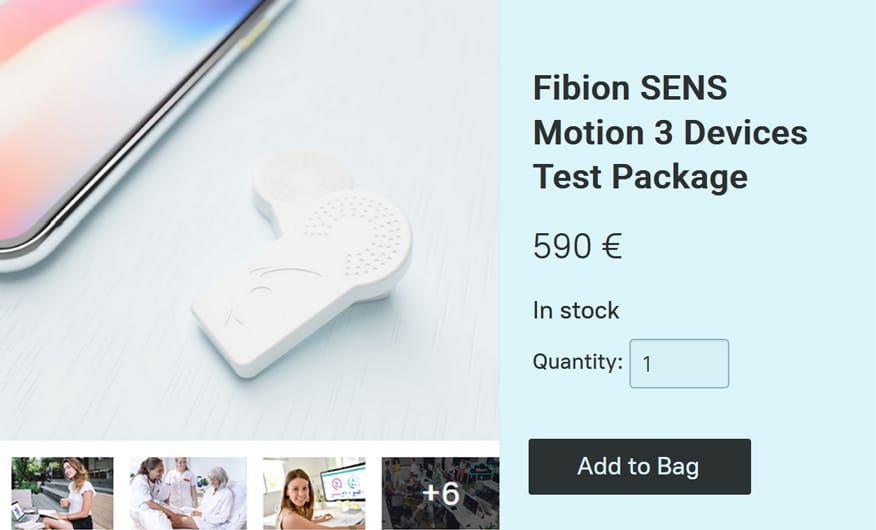
🔍 You may also discover other valid and reliable products in our portfolio, such as the Fibion Device, Fibion Sleep, Fibion Mimove, Fibion Vitals, Fibion Emfit, and Fibion Circadian, all designed to assist in research measuring physical activity, sedentary behavior, and sleep.
Frequently Asked Questions
What are large language models (LLMs) and how are they used in research? +
Large language models (LLMs) are advanced AI systems trained on vast amounts of text data. They understand and generate human language, making them valuable for processing and interpreting big data in research, including physical activity studies.
How do LLMs improve data analysis in physical activity research? +
LLMs enhance data analysis by efficiently processing large volumes of data, recognizing hidden patterns, and providing deeper contextual insights. This leads to more accurate and comprehensive findings in physical activity research.
What are the main challenges in analyzing big data in physical activity studies? +
The main challenges include the complexity of physical activity data, the large volume of data from wearable devices and self-reports, and the limitations of traditional analysis methods in identifying subtle patterns and correlations.
What benefits do LLMs offer for physical activity research? +
LLMs offer enhanced insights by uncovering deeper patterns, improved time and accuracy in data analysis, and the ability to integrate various data sources for more comprehensive findings, leading to better health outcomes.
How will the future of LLMs impact physical activity research? +
As LLM technology evolves, its applications in physical activity research will expand, offering more sophisticated models with greater data integration and analysis capabilities. This will provide researchers with even more powerful tools for innovation and improved health outcomes.